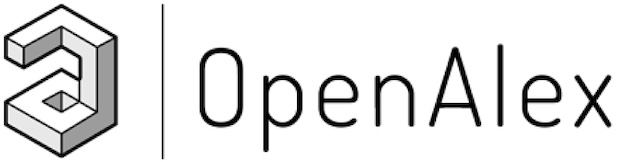
OpenAlex is a bibliographic catalogue of scientific papers, authors and institutions accessible in open access mode, named after the Library of Alexandria. It's citation coverage is excellent and I hope you will find utility in this listing of citing articles!
If you click the article title, you'll navigate to the article, as listed in CrossRef. If you click the Open Access links, you'll navigate to the "best Open Access location". Clicking the citation count will open this listing for that article. Lastly at the bottom of the page, you'll find basic pagination options.
Requested Article:
When ancient numerical demons meet physics-informed machine learning: adjoint-based gradients for implicit differentiable modeling
Yalan Song, Wouter Knoben, Martyn Clark, et al.
Hydrology and earth system sciences (2024) Vol. 28, Iss. 13, pp. 3051-3077
Open Access | Times Cited: 6
Yalan Song, Wouter Knoben, Martyn Clark, et al.
Hydrology and earth system sciences (2024) Vol. 28, Iss. 13, pp. 3051-3077
Open Access | Times Cited: 6
Showing 6 citing articles:
Machine Learning for a Heterogeneous Water Modeling Framework
Jonathan Frame, Ryoko Araki, Soelem Aafnan Bhuiyan, et al.
JAWRA Journal of the American Water Resources Association (2025) Vol. 61, Iss. 1
Open Access | Times Cited: 1
Jonathan Frame, Ryoko Araki, Soelem Aafnan Bhuiyan, et al.
JAWRA Journal of the American Water Resources Association (2025) Vol. 61, Iss. 1
Open Access | Times Cited: 1
Coupling SWAT+ with LSTM for enhanced and interpretable streamflow estimation in arid and semi-arid watersheds, a case study of the Tagus Headwaters River Basin, Spain
Sara Asadi, Patricia Jimeno‐Sáez, Adrián López-Ballesteros, et al.
Environmental Modelling & Software (2025), pp. 106360-106360
Open Access
Sara Asadi, Patricia Jimeno‐Sáez, Adrián López-Ballesteros, et al.
Environmental Modelling & Software (2025), pp. 106360-106360
Open Access
Protocols for Water and Environmental Modeling Using Machine Learning in California
Minxue He, Prabhjot Sandhu, Peyman Namadi, et al.
Hydrology (2025) Vol. 12, Iss. 3, pp. 59-59
Open Access
Minxue He, Prabhjot Sandhu, Peyman Namadi, et al.
Hydrology (2025) Vol. 12, Iss. 3, pp. 59-59
Open Access
High‐Resolution National‐Scale Water Modeling Is Enhanced by Multiscale Differentiable Physics‐Informed Machine Learning
Yalan Song, Tadd Bindas, Chaopeng Shen, et al.
Water Resources Research (2025) Vol. 61, Iss. 4
Open Access
Yalan Song, Tadd Bindas, Chaopeng Shen, et al.
Water Resources Research (2025) Vol. 61, Iss. 4
Open Access
Deep dive into hydrologic simulations at global scale: harnessing the power of deep learning and physics-informed differentiable models (δHBV-globe1.0-hydroDL)
Dapeng Feng, Hylke E. Beck, Jens de Bruijn, et al.
Geoscientific model development (2024) Vol. 17, Iss. 18, pp. 7181-7198
Open Access | Times Cited: 4
Dapeng Feng, Hylke E. Beck, Jens de Bruijn, et al.
Geoscientific model development (2024) Vol. 17, Iss. 18, pp. 7181-7198
Open Access | Times Cited: 4
Learning Regionalization Using Accurate Spatial Cost Gradients Within a Differentiable High‐Resolution Hydrological Model: Application to the French Mediterranean Region
Ngo Nghi Truyen Huynh, Pierre‐André Garambois, François Colleoni, et al.
Water Resources Research (2024) Vol. 60, Iss. 11
Open Access | Times Cited: 1
Ngo Nghi Truyen Huynh, Pierre‐André Garambois, François Colleoni, et al.
Water Resources Research (2024) Vol. 60, Iss. 11
Open Access | Times Cited: 1
Rapid 2D hydrodynamic flood modeling using deep learning surrogates
Francisco Haces‐Garcia, Natalya Maslennikova, Craig Glennie, et al.
Journal of Hydrology (2024), pp. 132561-132561
Closed Access
Francisco Haces‐Garcia, Natalya Maslennikova, Craig Glennie, et al.
Journal of Hydrology (2024), pp. 132561-132561
Closed Access