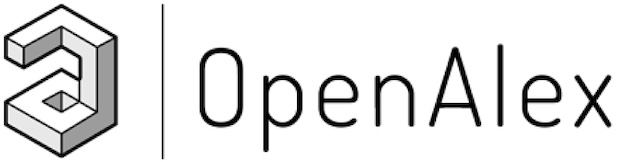
OpenAlex is a bibliographic catalogue of scientific papers, authors and institutions accessible in open access mode, named after the Library of Alexandria. It's citation coverage is excellent and I hope you will find utility in this listing of citing articles!
If you click the article title, you'll navigate to the article, as listed in CrossRef. If you click the Open Access links, you'll navigate to the "best Open Access location". Clicking the citation count will open this listing for that article. Lastly at the bottom of the page, you'll find basic pagination options.
Requested Article:
A graph neural network (GNN) approach to basin-scale river network learning: the role of physics-based connectivity and data fusion
Alexander Y. Sun, Peishi Jiang, Zong‐Liang Yang, et al.
Hydrology and earth system sciences (2022) Vol. 26, Iss. 19, pp. 5163-5184
Open Access | Times Cited: 42
Alexander Y. Sun, Peishi Jiang, Zong‐Liang Yang, et al.
Hydrology and earth system sciences (2022) Vol. 26, Iss. 19, pp. 5163-5184
Open Access | Times Cited: 42
Showing 1-25 of 42 citing articles:
Differentiable modelling to unify machine learning and physical models for geosciences
Chaopeng Shen, Alison P. Appling, Pierre Gentine, et al.
Nature Reviews Earth & Environment (2023) Vol. 4, Iss. 8, pp. 552-567
Closed Access | Times Cited: 164
Chaopeng Shen, Alison P. Appling, Pierre Gentine, et al.
Nature Reviews Earth & Environment (2023) Vol. 4, Iss. 8, pp. 552-567
Closed Access | Times Cited: 164
A review of hybrid deep learning applications for streamflow forecasting
Kin‐Wang Ng, Yuk Feng Huang, Chai Hoon Koo, et al.
Journal of Hydrology (2023) Vol. 625, pp. 130141-130141
Closed Access | Times Cited: 79
Kin‐Wang Ng, Yuk Feng Huang, Chai Hoon Koo, et al.
Journal of Hydrology (2023) Vol. 625, pp. 130141-130141
Closed Access | Times Cited: 79
Improving River Routing Using a Differentiable Muskingum‐Cunge Model and Physics‐Informed Machine Learning
Tadd Bindas, Wen‐Ping Tsai, Jiangtao Liu, et al.
Water Resources Research (2024) Vol. 60, Iss. 1
Open Access | Times Cited: 26
Tadd Bindas, Wen‐Ping Tsai, Jiangtao Liu, et al.
Water Resources Research (2024) Vol. 60, Iss. 1
Open Access | Times Cited: 26
Distributed Hydrological Modeling With Physics‐Encoded Deep Learning: A General Framework and Its Application in the Amazon
Chao Wang, Shijie Jiang, Yi Zheng, et al.
Water Resources Research (2024) Vol. 60, Iss. 4
Open Access | Times Cited: 18
Chao Wang, Shijie Jiang, Yi Zheng, et al.
Water Resources Research (2024) Vol. 60, Iss. 4
Open Access | Times Cited: 18
A novel machine learning-based framework for the water quality parameters prediction using hybrid long short-term memory and locally weighted scatterplot smoothing methods
Ana Dodig, Elisa Ricci, Goran Kvaščev, et al.
Journal of Hydroinformatics (2024) Vol. 26, Iss. 5, pp. 1059-1079
Open Access | Times Cited: 8
Ana Dodig, Elisa Ricci, Goran Kvaščev, et al.
Journal of Hydroinformatics (2024) Vol. 26, Iss. 5, pp. 1059-1079
Open Access | Times Cited: 8
Hydrogen jet and diffusion modeling by physics-informed graph neural network
Xinqi Zhang, Jihao Shi, Junjie Li, et al.
Renewable and Sustainable Energy Reviews (2024) Vol. 207, pp. 114898-114898
Closed Access | Times Cited: 8
Xinqi Zhang, Jihao Shi, Junjie Li, et al.
Renewable and Sustainable Energy Reviews (2024) Vol. 207, pp. 114898-114898
Closed Access | Times Cited: 8
Spatiotemporal estimation of groundwater and surface water conditions by integrating deep learning and physics-based watershed models
Soobin Kim, Eunhee Lee, Hyoun‐Tae Hwang, et al.
Water Research X (2024) Vol. 23, pp. 100228-100228
Open Access | Times Cited: 7
Soobin Kim, Eunhee Lee, Hyoun‐Tae Hwang, et al.
Water Research X (2024) Vol. 23, pp. 100228-100228
Open Access | Times Cited: 7
Enhancing hydrological data completeness: A performance evaluation of various machine learning techniques using probabilistic fusion imputer with neural networks for streamflow data reconstruction
G.R. Arathy Nair, S. Adarsh, Ahmed El‐Shafie, et al.
Journal of Hydrology (2024) Vol. 639, pp. 131583-131583
Closed Access | Times Cited: 6
G.R. Arathy Nair, S. Adarsh, Ahmed El‐Shafie, et al.
Journal of Hydrology (2024) Vol. 639, pp. 131583-131583
Closed Access | Times Cited: 6
A national-scale hybrid model for enhanced streamflow estimation – consolidating a physically based hydrological model with long short-term memory (LSTM) networks
Jun Liu, Julian Koch, Simon Stisen, et al.
Hydrology and earth system sciences (2024) Vol. 28, Iss. 13, pp. 2871-2893
Open Access | Times Cited: 5
Jun Liu, Julian Koch, Simon Stisen, et al.
Hydrology and earth system sciences (2024) Vol. 28, Iss. 13, pp. 2871-2893
Open Access | Times Cited: 5
Using tide for rainfall runoff simulation with feature projection and reversible instance normalization
Zheng Fang, Simin Qu, Xiaoqiang Yang, et al.
Scientific Reports (2025) Vol. 15, Iss. 1
Open Access
Zheng Fang, Simin Qu, Xiaoqiang Yang, et al.
Scientific Reports (2025) Vol. 15, Iss. 1
Open Access
Better localized predictions with Out-of-Scope information and Explainable AI: One-Shot SAR backscatter nowcast framework with data from neighboring region
Zhouyayan Li, İbrahim Demir
ISPRS Journal of Photogrammetry and Remote Sensing (2023) Vol. 207, pp. 92-103
Open Access | Times Cited: 11
Zhouyayan Li, İbrahim Demir
ISPRS Journal of Photogrammetry and Remote Sensing (2023) Vol. 207, pp. 92-103
Open Access | Times Cited: 11
A framework on utilizing of publicly availability stream gauges datasets and deep learning in estimating monthly basin-scale runoff in ungauged regions
Manh‐Hung Le, Hyunglok Kim, Hong Xuan, et al.
Advances in Water Resources (2024) Vol. 188, pp. 104694-104694
Closed Access | Times Cited: 4
Manh‐Hung Le, Hyunglok Kim, Hong Xuan, et al.
Advances in Water Resources (2024) Vol. 188, pp. 104694-104694
Closed Access | Times Cited: 4
Integrating Euclidean and non-Euclidean spatial information for deep learning-based spatiotemporal hydrological simulation
Liangkun Deng, Xiang Zhang, Louise Slater, et al.
Journal of Hydrology (2024) Vol. 638, pp. 131438-131438
Closed Access | Times Cited: 4
Liangkun Deng, Xiang Zhang, Louise Slater, et al.
Journal of Hydrology (2024) Vol. 638, pp. 131438-131438
Closed Access | Times Cited: 4
Time series predictions in unmonitored sites: a survey of machine learning techniques in water resources
Jared Willard, Charuleka Varadharajan, Xiaowei Jia, et al.
Environmental Data Science (2025) Vol. 4
Open Access
Jared Willard, Charuleka Varadharajan, Xiaowei Jia, et al.
Environmental Data Science (2025) Vol. 4
Open Access
Spatio-Temporal Graph Neural Networks for Streamflow Prediction in the Upper Colorado Basin
Akhila Akkala, Soukaïna Filali Boubrahimi, Shah Muhammad Hamdi, et al.
Hydrology (2025) Vol. 12, Iss. 3, pp. 60-60
Open Access
Akhila Akkala, Soukaïna Filali Boubrahimi, Shah Muhammad Hamdi, et al.
Hydrology (2025) Vol. 12, Iss. 3, pp. 60-60
Open Access
An efficient parallel runoff forecasting model for capturing global and local feature information
Yang-hao Hong, Dongmei Xu, Wenchuan Wang, et al.
Scientific Reports (2025) Vol. 15, Iss. 1
Open Access
Yang-hao Hong, Dongmei Xu, Wenchuan Wang, et al.
Scientific Reports (2025) Vol. 15, Iss. 1
Open Access
Multi-scale dynamic spatiotemporal graph attention network for forecasting karst spring discharge
Renjie Zhou
Journal of Hydrology (2025), pp. 133289-133289
Closed Access
Renjie Zhou
Journal of Hydrology (2025), pp. 133289-133289
Closed Access
Multi-step ahead probabilistic forecasting of multiple hydrological variables for multiple stations
Zhendong Zhang, Haihua Tang, Hui Qin, et al.
Journal of Hydrology (2023) Vol. 617, pp. 129094-129094
Closed Access | Times Cited: 10
Zhendong Zhang, Haihua Tang, Hui Qin, et al.
Journal of Hydrology (2023) Vol. 617, pp. 129094-129094
Closed Access | Times Cited: 10
Simulation of spring discharge using graph neural networks at Niangziguan Springs, China
Yujing Gai, Mingyang Wang, Yue Wu, et al.
Journal of Hydrology (2023) Vol. 625, pp. 130079-130079
Open Access | Times Cited: 10
Yujing Gai, Mingyang Wang, Yue Wu, et al.
Journal of Hydrology (2023) Vol. 625, pp. 130079-130079
Open Access | Times Cited: 10
Generative deep learning for probabilistic streamflow forecasting: Conditional variational auto-encoder
Mohammad Sina Jahangir, John Quilty
Journal of Hydrology (2023) Vol. 629, pp. 130498-130498
Closed Access | Times Cited: 10
Mohammad Sina Jahangir, John Quilty
Journal of Hydrology (2023) Vol. 629, pp. 130498-130498
Closed Access | Times Cited: 10
Rapid Flood Inundation Forecast Using Fourier Neural Operator
Alexander Y. Sun, Zhi Li, Wonhyun Lee, et al.
(2023), pp. 3735-3741
Open Access | Times Cited: 9
Alexander Y. Sun, Zhi Li, Wonhyun Lee, et al.
(2023), pp. 3735-3741
Open Access | Times Cited: 9
Graph neural network method for the intelligent selection of river system
Di Wang, Haizhong Qian
Geocarto International (2023) Vol. 38, Iss. 1
Open Access | Times Cited: 8
Di Wang, Haizhong Qian
Geocarto International (2023) Vol. 38, Iss. 1
Open Access | Times Cited: 8
Runoff Prediction Based on Dynamic Spatiotemporal Graph Neural Network
Shuai Yang, Yueqin Zhang, Zehua Zhang
Water (2023) Vol. 15, Iss. 13, pp. 2463-2463
Open Access | Times Cited: 7
Shuai Yang, Yueqin Zhang, Zehua Zhang
Water (2023) Vol. 15, Iss. 13, pp. 2463-2463
Open Access | Times Cited: 7
Predicting the growth trajectory and yield of greenhouse strawberries based on knowledge-guided computer vision
Qi Yang, Licheng Liu, Junxiong Zhou, et al.
Computers and Electronics in Agriculture (2024) Vol. 220, pp. 108911-108911
Closed Access | Times Cited: 2
Qi Yang, Licheng Liu, Junxiong Zhou, et al.
Computers and Electronics in Agriculture (2024) Vol. 220, pp. 108911-108911
Closed Access | Times Cited: 2
Predicting the Urban Stormwater Drainage System State using the Graph-WaveNet
Mengru Li, Xiaoming Shi, Zhongming Lu, et al.
Sustainable Cities and Society (2024), pp. 105877-105877
Closed Access | Times Cited: 2
Mengru Li, Xiaoming Shi, Zhongming Lu, et al.
Sustainable Cities and Society (2024), pp. 105877-105877
Closed Access | Times Cited: 2