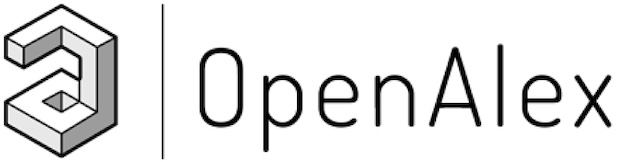
OpenAlex is a bibliographic catalogue of scientific papers, authors and institutions accessible in open access mode, named after the Library of Alexandria. It's citation coverage is excellent and I hope you will find utility in this listing of citing articles!
If you click the article title, you'll navigate to the article, as listed in CrossRef. If you click the Open Access links, you'll navigate to the "best Open Access location". Clicking the citation count will open this listing for that article. Lastly at the bottom of the page, you'll find basic pagination options.
Requested Article:
Rainfall–runoff prediction at multiple timescales with a single Long Short-Term Memory network
Martin Gauch, Frederik Kratzert, Daniel Klotz, et al.
Hydrology and earth system sciences (2021) Vol. 25, Iss. 4, pp. 2045-2062
Open Access | Times Cited: 182
Martin Gauch, Frederik Kratzert, Daniel Klotz, et al.
Hydrology and earth system sciences (2021) Vol. 25, Iss. 4, pp. 2045-2062
Open Access | Times Cited: 182
Showing 1-25 of 182 citing articles:
Deep learning rainfall–runoff predictions of extreme events
Jonathan Frame, Frederik Kratzert, Daniel Klotz, et al.
Hydrology and earth system sciences (2022) Vol. 26, Iss. 13, pp. 3377-3392
Open Access | Times Cited: 208
Jonathan Frame, Frederik Kratzert, Daniel Klotz, et al.
Hydrology and earth system sciences (2022) Vol. 26, Iss. 13, pp. 3377-3392
Open Access | Times Cited: 208
Post‐Processing the National Water Model with Long Short‐Term Memory Networks for Streamflow Predictions and Model Diagnostics
Jonathan Frame, Frederik Kratzert, Austin Raney, et al.
JAWRA Journal of the American Water Resources Association (2021) Vol. 57, Iss. 6, pp. 885-905
Open Access | Times Cited: 201
Jonathan Frame, Frederik Kratzert, Austin Raney, et al.
JAWRA Journal of the American Water Resources Association (2021) Vol. 57, Iss. 6, pp. 885-905
Open Access | Times Cited: 201
Benchmarking data-driven rainfall–runoff models in Great Britain: a comparison of long short-term memory (LSTM)-based models with four lumped conceptual models
Thomas Lees, Marcus Buechel, Bailey Anderson, et al.
Hydrology and earth system sciences (2021) Vol. 25, Iss. 10, pp. 5517-5534
Open Access | Times Cited: 172
Thomas Lees, Marcus Buechel, Bailey Anderson, et al.
Hydrology and earth system sciences (2021) Vol. 25, Iss. 10, pp. 5517-5534
Open Access | Times Cited: 172
NeuralHydrology — A Python library for Deep Learning research in hydrology
Frederik Kratzert, Martin Gauch, Grey Nearing, et al.
The Journal of Open Source Software (2022) Vol. 7, Iss. 71, pp. 4050-4050
Open Access | Times Cited: 137
Frederik Kratzert, Martin Gauch, Grey Nearing, et al.
The Journal of Open Source Software (2022) Vol. 7, Iss. 71, pp. 4050-4050
Open Access | Times Cited: 137
The Data Synergy Effects of Time‐Series Deep Learning Models in Hydrology
Kuai Fang, Daniel Kifer, Kathryn Lawson, et al.
Water Resources Research (2022) Vol. 58, Iss. 4
Open Access | Times Cited: 134
Kuai Fang, Daniel Kifer, Kathryn Lawson, et al.
Water Resources Research (2022) Vol. 58, Iss. 4
Open Access | Times Cited: 134
Hydrological concept formation inside long short-term memory (LSTM) networks
Thomas Lees, Steven Reece, Frederik Kratzert, et al.
Hydrology and earth system sciences (2022) Vol. 26, Iss. 12, pp. 3079-3101
Open Access | Times Cited: 130
Thomas Lees, Steven Reece, Frederik Kratzert, et al.
Hydrology and earth system sciences (2022) Vol. 26, Iss. 12, pp. 3079-3101
Open Access | Times Cited: 130
Flood forecasting with machine learning models in an operational framework
Sella Nevo, Efrat Morin, Adi Gerzi Rosenthal, et al.
Hydrology and earth system sciences (2022) Vol. 26, Iss. 15, pp. 4013-4032
Open Access | Times Cited: 122
Sella Nevo, Efrat Morin, Adi Gerzi Rosenthal, et al.
Hydrology and earth system sciences (2022) Vol. 26, Iss. 15, pp. 4013-4032
Open Access | Times Cited: 122
Continuous streamflow prediction in ungauged basins: long short-term memory neural networks clearly outperform traditional hydrological models
Richard Arsenault, Jean‐Luc Martel, Frédéric Brunet, et al.
Hydrology and earth system sciences (2023) Vol. 27, Iss. 1, pp. 139-157
Open Access | Times Cited: 105
Richard Arsenault, Jean‐Luc Martel, Frédéric Brunet, et al.
Hydrology and earth system sciences (2023) Vol. 27, Iss. 1, pp. 139-157
Open Access | Times Cited: 105
Assessing the Physical Realism of Deep Learning Hydrologic Model Projections Under Climate Change
Sungwook Wi, Scott Steinschneider
Water Resources Research (2022) Vol. 58, Iss. 9
Closed Access | Times Cited: 86
Sungwook Wi, Scott Steinschneider
Water Resources Research (2022) Vol. 58, Iss. 9
Closed Access | Times Cited: 86
The Great Lakes Runoff Intercomparison Project Phase 4: the Great Lakes (GRIP-GL)
Juliane Mai, Hongren Shen, Bryan A. Tolson, et al.
Hydrology and earth system sciences (2022) Vol. 26, Iss. 13, pp. 3537-3572
Open Access | Times Cited: 82
Juliane Mai, Hongren Shen, Bryan A. Tolson, et al.
Hydrology and earth system sciences (2022) Vol. 26, Iss. 13, pp. 3537-3572
Open Access | Times Cited: 82
Using a long short-term memory (LSTM) neural network to boost river streamflow forecasts over the western United States
Kieran M. R. Hunt, Gwyneth Matthews, Florian Pappenberger, et al.
Hydrology and earth system sciences (2022) Vol. 26, Iss. 21, pp. 5449-5472
Open Access | Times Cited: 80
Kieran M. R. Hunt, Gwyneth Matthews, Florian Pappenberger, et al.
Hydrology and earth system sciences (2022) Vol. 26, Iss. 21, pp. 5449-5472
Open Access | Times Cited: 80
Deep transfer learning based on transformer for flood forecasting in data-sparse basins
Yuanhao Xu, Kairong Lin, Caihong Hu, et al.
Journal of Hydrology (2023) Vol. 625, pp. 129956-129956
Closed Access | Times Cited: 63
Yuanhao Xu, Kairong Lin, Caihong Hu, et al.
Journal of Hydrology (2023) Vol. 625, pp. 129956-129956
Closed Access | Times Cited: 63
In Defense of Metrics: Metrics Sufficiently Encode Typical Human Preferences Regarding Hydrological Model Performance
Martin Gauch, Frederik Kratzert, Oren Gilon, et al.
Water Resources Research (2023) Vol. 59, Iss. 6
Open Access | Times Cited: 46
Martin Gauch, Frederik Kratzert, Oren Gilon, et al.
Water Resources Research (2023) Vol. 59, Iss. 6
Open Access | Times Cited: 46
HESS Opinions: Never train a Long Short-Term Memory (LSTM) network on a single basin
Frederik Kratzert, Martin Gauch, Daniel Klotz, et al.
Hydrology and earth system sciences (2024) Vol. 28, Iss. 17, pp. 4187-4201
Open Access | Times Cited: 19
Frederik Kratzert, Martin Gauch, Daniel Klotz, et al.
Hydrology and earth system sciences (2024) Vol. 28, Iss. 17, pp. 4187-4201
Open Access | Times Cited: 19
Enhanced streamflow forecasting using hybrid modelling integrating glacio-hydrological outputs, deep learning and wavelet transformation
Jamal Hassan Ougahi, John S. Rowan
Scientific Reports (2025) Vol. 15, Iss. 1
Open Access | Times Cited: 2
Jamal Hassan Ougahi, John S. Rowan
Scientific Reports (2025) Vol. 15, Iss. 1
Open Access | Times Cited: 2
Continental-scale streamflow modeling of basins with reservoirs: Towards a coherent deep-learning-based strategy
Wenyu Ouyang, Kathryn Lawson, Dapeng Feng, et al.
Journal of Hydrology (2021) Vol. 599, pp. 126455-126455
Closed Access | Times Cited: 77
Wenyu Ouyang, Kathryn Lawson, Dapeng Feng, et al.
Journal of Hydrology (2021) Vol. 599, pp. 126455-126455
Closed Access | Times Cited: 77
Can machine learning accelerate process understanding and decision‐relevant predictions of river water quality?
Charuleka Varadharajan, Alison P. Appling, Bhavna Arora, et al.
Hydrological Processes (2022) Vol. 36, Iss. 4
Open Access | Times Cited: 53
Charuleka Varadharajan, Alison P. Appling, Bhavna Arora, et al.
Hydrological Processes (2022) Vol. 36, Iss. 4
Open Access | Times Cited: 53
Improving hydrologic models for predictions and process understanding using neural ODEs
Marvin Höge, Andreas Scheidegger, Marco Baity‐Jesi, et al.
Hydrology and earth system sciences (2022) Vol. 26, Iss. 19, pp. 5085-5102
Open Access | Times Cited: 48
Marvin Höge, Andreas Scheidegger, Marco Baity‐Jesi, et al.
Hydrology and earth system sciences (2022) Vol. 26, Iss. 19, pp. 5085-5102
Open Access | Times Cited: 48
Optimal Postprocessing Strategies With LSTM for Global Streamflow Prediction in Ungauged Basins
Senlin Tang, Fubao Sun, Wenbin Liu, et al.
Water Resources Research (2023) Vol. 59, Iss. 7
Closed Access | Times Cited: 32
Senlin Tang, Fubao Sun, Wenbin Liu, et al.
Water Resources Research (2023) Vol. 59, Iss. 7
Closed Access | Times Cited: 32
On strictly enforced mass conservation constraints for modelling the Rainfall‐Runoff process
Jonathan Frame, Frederik Kratzert, Hoshin V. Gupta, et al.
Hydrological Processes (2023) Vol. 37, Iss. 3
Closed Access | Times Cited: 29
Jonathan Frame, Frederik Kratzert, Hoshin V. Gupta, et al.
Hydrological Processes (2023) Vol. 37, Iss. 3
Closed Access | Times Cited: 29
Evaluation of Transformer model and Self-Attention mechanism in the Yangtze River basin runoff prediction
Xikun Wei, Guojie Wang, Britta Schmalz, et al.
Journal of Hydrology Regional Studies (2023) Vol. 47, pp. 101438-101438
Open Access | Times Cited: 29
Xikun Wei, Guojie Wang, Britta Schmalz, et al.
Journal of Hydrology Regional Studies (2023) Vol. 47, pp. 101438-101438
Open Access | Times Cited: 29
Effects of mass balance, energy balance, and storage-discharge constraints on LSTM for streamflow prediction
Sudan Pokharel, Tirthankar Roy, David M. Admiraal
Environmental Modelling & Software (2023) Vol. 166, pp. 105730-105730
Open Access | Times Cited: 27
Sudan Pokharel, Tirthankar Roy, David M. Admiraal
Environmental Modelling & Software (2023) Vol. 166, pp. 105730-105730
Open Access | Times Cited: 27
A Novel Smoothing-Based Deep Learning Time-Series Approach for Daily Suspended Sediment Load Prediction
Bibhuti Bhusan Sahoo, Sovan Sankalp, Özgür Kişi
Water Resources Management (2023) Vol. 37, Iss. 11, pp. 4271-4292
Closed Access | Times Cited: 25
Bibhuti Bhusan Sahoo, Sovan Sankalp, Özgür Kişi
Water Resources Management (2023) Vol. 37, Iss. 11, pp. 4271-4292
Closed Access | Times Cited: 25
Predicting streamflow with LSTM networks using global datasets
Katharina Wilbrand, Riccardo Taormina, Marie‐Claire ten Veldhuis, et al.
Frontiers in Water (2023) Vol. 5
Open Access | Times Cited: 24
Katharina Wilbrand, Riccardo Taormina, Marie‐Claire ten Veldhuis, et al.
Frontiers in Water (2023) Vol. 5
Open Access | Times Cited: 24
On the need for physical constraints in deep learning rainfall–runoff projections under climate change: a sensitivity analysis to warming and shifts in potential evapotranspiration
Sungwook Wi, Scott Steinschneider
Hydrology and earth system sciences (2024) Vol. 28, Iss. 3, pp. 479-503
Open Access | Times Cited: 15
Sungwook Wi, Scott Steinschneider
Hydrology and earth system sciences (2024) Vol. 28, Iss. 3, pp. 479-503
Open Access | Times Cited: 15