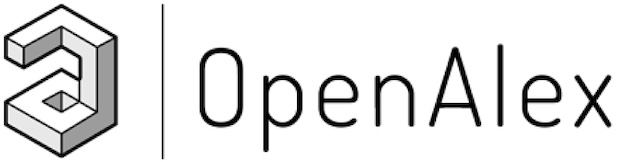
OpenAlex is a bibliographic catalogue of scientific papers, authors and institutions accessible in open access mode, named after the Library of Alexandria. It's citation coverage is excellent and I hope you will find utility in this listing of citing articles!
If you click the article title, you'll navigate to the article, as listed in CrossRef. If you click the Open Access links, you'll navigate to the "best Open Access location". Clicking the citation count will open this listing for that article. Lastly at the bottom of the page, you'll find basic pagination options.
Requested Article:
Estimating the global abundance of ground level presence of particulate matter (PM2.5)
David J. Lary, Fazlay Faruque, N. K. Malakar, et al.
Geospatial health (2014) Vol. 8, Iss. 3, pp. 611-611
Open Access | Times Cited: 99
David J. Lary, Fazlay Faruque, N. K. Malakar, et al.
Geospatial health (2014) Vol. 8, Iss. 3, pp. 611-611
Open Access | Times Cited: 99
Showing 1-25 of 99 citing articles:
Machine learning in geosciences and remote sensing
David J. Lary, Amir H. Alavi, Amir H. Gandomi, et al.
Geoscience Frontiers (2015) Vol. 7, Iss. 1, pp. 3-10
Open Access | Times Cited: 951
David J. Lary, Amir H. Alavi, Amir H. Gandomi, et al.
Geoscience Frontiers (2015) Vol. 7, Iss. 1, pp. 3-10
Open Access | Times Cited: 951
Desert dust hazards: A global review
Nick Middleton
Aeolian Research (2016) Vol. 24, pp. 53-63
Open Access | Times Cited: 506
Nick Middleton
Aeolian Research (2016) Vol. 24, pp. 53-63
Open Access | Times Cited: 506
Improving performance of spatio-temporal machine learning models using forward feature selection and target-oriented validation
Hanna Meyer, Christoph Reudenbach, Tomislav Hengl, et al.
Environmental Modelling & Software (2017) Vol. 101, pp. 1-9
Closed Access | Times Cited: 388
Hanna Meyer, Christoph Reudenbach, Tomislav Hengl, et al.
Environmental Modelling & Software (2017) Vol. 101, pp. 1-9
Closed Access | Times Cited: 388
Estimating Ground‐Level PM2.5 by Fusing Satellite and Station Observations: A Geo‐Intelligent Deep Learning Approach
Tongwen Li, Huanfeng Shen, Qiangqiang Yuan, et al.
Geophysical Research Letters (2017) Vol. 44, Iss. 23
Open Access | Times Cited: 379
Tongwen Li, Huanfeng Shen, Qiangqiang Yuan, et al.
Geophysical Research Letters (2017) Vol. 44, Iss. 23
Open Access | Times Cited: 379
Review on recent progress in observations, source identifications and countermeasures of PM2.5
Chun‐Sheng Liang, Fengkui Duan, Kebin He, et al.
Environment International (2015) Vol. 86, pp. 150-170
Closed Access | Times Cited: 325
Chun‐Sheng Liang, Fengkui Duan, Kebin He, et al.
Environment International (2015) Vol. 86, pp. 150-170
Closed Access | Times Cited: 325
A systematic review of data mining and machine learning for air pollution epidemiology
Colin Bellinger, Mohomed Shazan Mohomed Jabbar, Osmar R. Zaı̈ane, et al.
BMC Public Health (2017) Vol. 17, Iss. 1
Open Access | Times Cited: 218
Colin Bellinger, Mohomed Shazan Mohomed Jabbar, Osmar R. Zaı̈ane, et al.
BMC Public Health (2017) Vol. 17, Iss. 1
Open Access | Times Cited: 218
A Review on Predicting Ground PM2.5 Concentration Using Satellite Aerosol Optical Depth
Yuanyuan Chu, Yisi Liu, Xiangyu Li, et al.
Atmosphere (2016) Vol. 7, Iss. 10, pp. 129-129
Open Access | Times Cited: 179
Yuanyuan Chu, Yisi Liu, Xiangyu Li, et al.
Atmosphere (2016) Vol. 7, Iss. 10, pp. 129-129
Open Access | Times Cited: 179
Global estimates of daily ambient fine particulate matter concentrations and unequal spatiotemporal distribution of population exposure: a machine learning modelling study
Wenhua Yu, Tingting Ye, Yiwen Zhang, et al.
The Lancet Planetary Health (2023) Vol. 7, Iss. 3, pp. e209-e218
Closed Access | Times Cited: 113
Wenhua Yu, Tingting Ye, Yiwen Zhang, et al.
The Lancet Planetary Health (2023) Vol. 7, Iss. 3, pp. e209-e218
Closed Access | Times Cited: 113
First close insight into global daily gapless 1 km PM2.5 pollution, variability, and health impact
Jing Wei, Zhanqing Li, Alexei Lyapustin, et al.
Nature Communications (2023) Vol. 14, Iss. 1
Open Access | Times Cited: 50
Jing Wei, Zhanqing Li, Alexei Lyapustin, et al.
Nature Communications (2023) Vol. 14, Iss. 1
Open Access | Times Cited: 50
Population exposure to multiple air pollutants and its compound episodes in Europe
Zhaoyue Chen, Hervé Petetin, Raúl Fernando Méndez Turrubiates, et al.
Nature Communications (2024) Vol. 15, Iss. 1
Open Access | Times Cited: 20
Zhaoyue Chen, Hervé Petetin, Raúl Fernando Méndez Turrubiates, et al.
Nature Communications (2024) Vol. 15, Iss. 1
Open Access | Times Cited: 20
A Comparison of Machine Learning-Based Approaches in Estimating Surface PM2.5 Concentrations Focusing on Artificial Neural Networks and High Pollution Events
Su-Huai Wei, Kyle Shores, Yangyang Xu
Atmosphere (2025) Vol. 16, Iss. 1, pp. 48-48
Open Access | Times Cited: 2
Su-Huai Wei, Kyle Shores, Yangyang Xu
Atmosphere (2025) Vol. 16, Iss. 1, pp. 48-48
Open Access | Times Cited: 2
Estimating Regional Ground‐Level PM2.5 Directly From Satellite Top‐Of‐Atmosphere Reflectance Using Deep Belief Networks
Huanfeng Shen, Tongwen Li, Qiangqiang Yuan, et al.
Journal of Geophysical Research Atmospheres (2018) Vol. 123, Iss. 24
Open Access | Times Cited: 133
Huanfeng Shen, Tongwen Li, Qiangqiang Yuan, et al.
Journal of Geophysical Research Atmospheres (2018) Vol. 123, Iss. 24
Open Access | Times Cited: 133
Improving the Accuracy of Daily PM2.5 Distributions Derived from the Fusion of Ground-Level Measurements with Aerosol Optical Depth Observations, a Case Study in North China
Baolei Lv, Yongtao Hu, Howard H. Chang, et al.
Environmental Science & Technology (2016) Vol. 50, Iss. 9, pp. 4752-4759
Closed Access | Times Cited: 120
Baolei Lv, Yongtao Hu, Howard H. Chang, et al.
Environmental Science & Technology (2016) Vol. 50, Iss. 9, pp. 4752-4759
Closed Access | Times Cited: 120
Geographically and temporally weighted neural networks for satellite-based mapping of ground-level PM2.5
Tongwen Li, Huanfeng Shen, Qiangqiang Yuan, et al.
ISPRS Journal of Photogrammetry and Remote Sensing (2020) Vol. 167, pp. 178-188
Open Access | Times Cited: 87
Tongwen Li, Huanfeng Shen, Qiangqiang Yuan, et al.
ISPRS Journal of Photogrammetry and Remote Sensing (2020) Vol. 167, pp. 178-188
Open Access | Times Cited: 87
Using Machine Learning to Estimate Global PM2.5 for Environmental Health Studies
David J. Lary, Tatiana Lary, Barbara Sattler
Environmental Health Insights (2015) Vol. 9s1, pp. EHI.S15664-EHI.S15664
Open Access | Times Cited: 77
David J. Lary, Tatiana Lary, Barbara Sattler
Environmental Health Insights (2015) Vol. 9s1, pp. EHI.S15664-EHI.S15664
Open Access | Times Cited: 77
Machine Learning Applications for Earth Observation
David J. Lary, Gebreab K. Zewdie, Xun Liu, et al.
Springer eBooks (2018), pp. 165-218
Closed Access | Times Cited: 73
David J. Lary, Gebreab K. Zewdie, Xun Liu, et al.
Springer eBooks (2018), pp. 165-218
Closed Access | Times Cited: 73
Earth Observation Open Science and Innovation
Pierre-Philippe Mathieu, Christoph Aubrecht
Springer eBooks (2018)
Closed Access | Times Cited: 70
Pierre-Philippe Mathieu, Christoph Aubrecht
Springer eBooks (2018)
Closed Access | Times Cited: 70
Improving satellite-based PM2.5 estimates in China using Gaussian processes modeling in a Bayesian hierarchical setting
Wenxi Yu, Yang Liu, Zongwei Ma, et al.
Scientific Reports (2017) Vol. 7, Iss. 1
Open Access | Times Cited: 65
Wenxi Yu, Yang Liu, Zongwei Ma, et al.
Scientific Reports (2017) Vol. 7, Iss. 1
Open Access | Times Cited: 65
Mapping the geogenic radon potential for Germany by machine learning
Eric Petermann, Hanna Meyer, Madlene Nussbaum, et al.
The Science of The Total Environment (2020) Vol. 754, pp. 142291-142291
Open Access | Times Cited: 65
Eric Petermann, Hanna Meyer, Madlene Nussbaum, et al.
The Science of The Total Environment (2020) Vol. 754, pp. 142291-142291
Open Access | Times Cited: 65
Meteorological parameters and gaseous pollutant concentrations as predictors of daily continuous PM2.5 concentrations using deep neural network in Beijing–Tianjin–Hebei, China
Xinpeng Wang, Wenbin Sun
Atmospheric Environment (2019) Vol. 211, pp. 128-137
Closed Access | Times Cited: 60
Xinpeng Wang, Wenbin Sun
Atmospheric Environment (2019) Vol. 211, pp. 128-137
Closed Access | Times Cited: 60
Chemical characteristics, sources, and formation mechanisms of PM2.5 before and during the Spring Festival in a coastal city in Southeast China
Shanshan Wang, Ruilian Yu, Huazhen Shen, et al.
Environmental Pollution (2019) Vol. 251, pp. 442-452
Closed Access | Times Cited: 56
Shanshan Wang, Ruilian Yu, Huazhen Shen, et al.
Environmental Pollution (2019) Vol. 251, pp. 442-452
Closed Access | Times Cited: 56
An association between air pollution and daily most frequently visits of eighteen outpatient diseases in an industrial city
Tang-Tat Chau, Kuo-Ying Wang
Scientific Reports (2020) Vol. 10, Iss. 1
Open Access | Times Cited: 53
Tang-Tat Chau, Kuo-Ying Wang
Scientific Reports (2020) Vol. 10, Iss. 1
Open Access | Times Cited: 53
Applications of artificial intelligence in the field of air pollution: A bibliometric analysis
Qiangqiang Guo, Mengjuan Ren, Shouyuan Wu, et al.
Frontiers in Public Health (2022) Vol. 10
Open Access | Times Cited: 31
Qiangqiang Guo, Mengjuan Ren, Shouyuan Wu, et al.
Frontiers in Public Health (2022) Vol. 10
Open Access | Times Cited: 31
Critical Review of Methods to Estimate PM2.5 Concentrations within Specified Research Region
Guangyuan Zhang, Xiaoping Rui, Yonglei Fan
ISPRS International Journal of Geo-Information (2018) Vol. 7, Iss. 9, pp. 368-368
Open Access | Times Cited: 56
Guangyuan Zhang, Xiaoping Rui, Yonglei Fan
ISPRS International Journal of Geo-Information (2018) Vol. 7, Iss. 9, pp. 368-368
Open Access | Times Cited: 56
A Validation Approach Considering the Uneven Distribution of Ground Stations for Satellite-Based PM2.5 Estimation
Tongwen Li, Huanfeng Shen, Chao Zeng, et al.
IEEE Journal of Selected Topics in Applied Earth Observations and Remote Sensing (2020) Vol. 13, pp. 1312-1321
Open Access | Times Cited: 47
Tongwen Li, Huanfeng Shen, Chao Zeng, et al.
IEEE Journal of Selected Topics in Applied Earth Observations and Remote Sensing (2020) Vol. 13, pp. 1312-1321
Open Access | Times Cited: 47