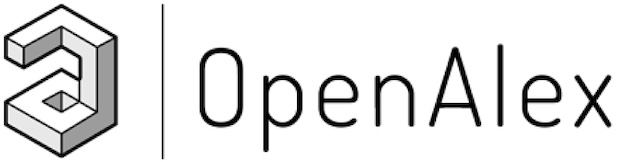
OpenAlex is a bibliographic catalogue of scientific papers, authors and institutions accessible in open access mode, named after the Library of Alexandria. It's citation coverage is excellent and I hope you will find utility in this listing of citing articles!
If you click the article title, you'll navigate to the article, as listed in CrossRef. If you click the Open Access links, you'll navigate to the "best Open Access location". Clicking the citation count will open this listing for that article. Lastly at the bottom of the page, you'll find basic pagination options.
Requested Article:
A Novel Machine Learning-Based Methodology for Tool Wear Prediction Using Acoustic Emission Signals
Juan Luís Ferrando, Telmo Fernández De Barrena Sarasola, Ander García, et al.
Sensors (2021) Vol. 21, Iss. 17, pp. 5984-5984
Open Access | Times Cited: 56
Juan Luís Ferrando, Telmo Fernández De Barrena Sarasola, Ander García, et al.
Sensors (2021) Vol. 21, Iss. 17, pp. 5984-5984
Open Access | Times Cited: 56
Showing 1-25 of 56 citing articles:
Machine learning and artificial intelligence in CNC machine tools, A review
Mohsen Soori, Behrooz Arezoo, Roza Dastres
Sustainable Manufacturing and Service Economics (2023) Vol. 2, pp. 100009-100009
Open Access | Times Cited: 96
Mohsen Soori, Behrooz Arezoo, Roza Dastres
Sustainable Manufacturing and Service Economics (2023) Vol. 2, pp. 100009-100009
Open Access | Times Cited: 96
Tool Condition Monitoring for High-Performance Machining Systems—A Review
Ayman Mohamed, Mahmoud Hassan, Rachid M’Saoubi, et al.
Sensors (2022) Vol. 22, Iss. 6, pp. 2206-2206
Open Access | Times Cited: 88
Ayman Mohamed, Mahmoud Hassan, Rachid M’Saoubi, et al.
Sensors (2022) Vol. 22, Iss. 6, pp. 2206-2206
Open Access | Times Cited: 88
Prediction and classification of tool wear and its state in sustainable machining of Bohler steel with different machine learning models
Mehmet Erdi Korkmaz, Munish Kumar Gupta, Mustafa Kuntoğlu, et al.
Measurement (2023) Vol. 223, pp. 113825-113825
Closed Access | Times Cited: 48
Mehmet Erdi Korkmaz, Munish Kumar Gupta, Mustafa Kuntoğlu, et al.
Measurement (2023) Vol. 223, pp. 113825-113825
Closed Access | Times Cited: 48
Machine learning and IoT-based approach for tool condition monitoring: A review and future prospects
Minh‐Quang Tran, Hoang-Phuong Doan, Viet Q. Vu, et al.
Measurement (2022) Vol. 207, pp. 112351-112351
Closed Access | Times Cited: 63
Minh‐Quang Tran, Hoang-Phuong Doan, Viet Q. Vu, et al.
Measurement (2022) Vol. 207, pp. 112351-112351
Closed Access | Times Cited: 63
Accurate estimation of tool wear levels during milling, drilling and turning operations by designing novel hyperparameter tuned models based on LightGBM and stacking
Jawad Mahmood, Ghulam-e Mustafa, Muhammad Ali
Measurement (2022) Vol. 190, pp. 110722-110722
Closed Access | Times Cited: 40
Jawad Mahmood, Ghulam-e Mustafa, Muhammad Ali
Measurement (2022) Vol. 190, pp. 110722-110722
Closed Access | Times Cited: 40
Measurement and prediction of wear volume of the tool in nonlinear degradation process based on multi-sensor information fusion
Kangping Gao, Xinxin Xu, Shengjie Jiao
Engineering Failure Analysis (2022) Vol. 136, pp. 106164-106164
Closed Access | Times Cited: 38
Kangping Gao, Xinxin Xu, Shengjie Jiao
Engineering Failure Analysis (2022) Vol. 136, pp. 106164-106164
Closed Access | Times Cited: 38
A SYSTEMATIC LITERATURE REVIEW: RECURSIVE FEATURE ELIMINATION ALGORITHMS
Arif Mudi Priyatno, Triyanna Widiyaningtyas
JITK (Jurnal Ilmu Pengetahuan dan Teknologi Komputer) (2024) Vol. 9, Iss. 2, pp. 196-207
Open Access | Times Cited: 13
Arif Mudi Priyatno, Triyanna Widiyaningtyas
JITK (Jurnal Ilmu Pengetahuan dan Teknologi Komputer) (2024) Vol. 9, Iss. 2, pp. 196-207
Open Access | Times Cited: 13
AI-based optimisation of total machining performance: A review
Katrin Ullrich, Magnus von Elling, Kevin Gutzeit, et al.
CIRP journal of manufacturing science and technology (2024) Vol. 50, pp. 40-54
Open Access | Times Cited: 13
Katrin Ullrich, Magnus von Elling, Kevin Gutzeit, et al.
CIRP journal of manufacturing science and technology (2024) Vol. 50, pp. 40-54
Open Access | Times Cited: 13
Tool remaining useful life prediction using bidirectional recurrent neural networks (BRNN)
Telmo Fernández De Barrena Sarasola, Juan Luís Ferrando, Ander García, et al.
The International Journal of Advanced Manufacturing Technology (2023) Vol. 125, Iss. 9-10, pp. 4027-4045
Open Access | Times Cited: 17
Telmo Fernández De Barrena Sarasola, Juan Luís Ferrando, Ander García, et al.
The International Journal of Advanced Manufacturing Technology (2023) Vol. 125, Iss. 9-10, pp. 4027-4045
Open Access | Times Cited: 17
A single-sensor method for structural damage localization in wind turbine blades: Laboratory assessment on a blade segment
Zhimin Zhao, Nian-Zhong Chen
Mechanical Systems and Signal Processing (2024) Vol. 214, pp. 111370-111370
Closed Access | Times Cited: 5
Zhimin Zhao, Nian-Zhong Chen
Mechanical Systems and Signal Processing (2024) Vol. 214, pp. 111370-111370
Closed Access | Times Cited: 5
Machine-Learning- and Internet-of-Things-Driven Techniques for Monitoring Tool Wear in Machining Process: A Comprehensive Review
Sudhan Kasiviswanathan, G. Sakthivel, T. Mohanraj, et al.
Journal of Sensor and Actuator Networks (2024) Vol. 13, Iss. 5, pp. 53-53
Open Access | Times Cited: 5
Sudhan Kasiviswanathan, G. Sakthivel, T. Mohanraj, et al.
Journal of Sensor and Actuator Networks (2024) Vol. 13, Iss. 5, pp. 53-53
Open Access | Times Cited: 5
Predictive modelling of tool wear in CFRP drilling using acoustic emission sensors under dry and cryogenic conditions
Vijayathithan Mathiyazhagan, Anil Meena
Wear (2025), pp. 205930-205930
Closed Access
Vijayathithan Mathiyazhagan, Anil Meena
Wear (2025), pp. 205930-205930
Closed Access
Deep-learning-driven intelligent tool wear identification of high-precision machining with multi-scale CNN-BiLSTM-GCN
Zhicheng Xu, Baolong Zhang, Louis Luo Fan, et al.
Advanced Engineering Informatics (2025) Vol. 65, pp. 103234-103234
Closed Access
Zhicheng Xu, Baolong Zhang, Louis Luo Fan, et al.
Advanced Engineering Informatics (2025) Vol. 65, pp. 103234-103234
Closed Access
In Situ Measurement of the Machining State in Small-Diameter Drilling by Acoustic Emission Sensing
Alan HASE
(2024)
Open Access | Times Cited: 4
Alan HASE
(2024)
Open Access | Times Cited: 4
Ball-end tool wear monitoring and multi-step forecasting with multi-modal information under variable cutting conditions
Yanpeng Hao, Lida Zhu, Jinsheng Wang, et al.
Journal of Manufacturing Systems (2024) Vol. 76, pp. 234-258
Closed Access | Times Cited: 4
Yanpeng Hao, Lida Zhu, Jinsheng Wang, et al.
Journal of Manufacturing Systems (2024) Vol. 76, pp. 234-258
Closed Access | Times Cited: 4
Sensorless tool wear estimation by using the artificial intelligence (AI) tools from the currents of motors generating linear motions
Mustafa Demetgül, Anand D. Darji, İbrahim N. Tansel, et al.
The International Journal of Advanced Manufacturing Technology (2025)
Closed Access
Mustafa Demetgül, Anand D. Darji, İbrahim N. Tansel, et al.
The International Journal of Advanced Manufacturing Technology (2025)
Closed Access
Exploring the correlation between design of experiments and machine learning prediction accuracy in ultra-precision hard turning of AISI D2 with CBN insert: a comparative study of Taguchi and full factorial designs
Ugonna Loveday Adizue, Márton Takács
The International Journal of Advanced Manufacturing Technology (2025)
Open Access
Ugonna Loveday Adizue, Márton Takács
The International Journal of Advanced Manufacturing Technology (2025)
Open Access
A novel simultaneous monitoring method for surface roughness and tool wear in milling process
Ruilin Liu, Wenwen Tian
Scientific Reports (2025) Vol. 15, Iss. 1
Open Access
Ruilin Liu, Wenwen Tian
Scientific Reports (2025) Vol. 15, Iss. 1
Open Access
Multi-dimensional tool wear detection using Non-Subsampled Shearlet Transform and enhanced pulse coupled neural network in optical microscopy reconstruction
Lei Li, Bing Li, Xiang Wei, et al.
Optics & Laser Technology (2025) Vol. 187, pp. 112849-112849
Closed Access
Lei Li, Bing Li, Xiang Wei, et al.
Optics & Laser Technology (2025) Vol. 187, pp. 112849-112849
Closed Access
A Physics-Assisted Online Learning Method for Tool Wear Prediction
Dezhi Yuan, Ting Luo, Dan Zhang, et al.
IEEE Transactions on Instrumentation and Measurement (2023) Vol. 72, pp. 1-11
Closed Access | Times Cited: 10
Dezhi Yuan, Ting Luo, Dan Zhang, et al.
IEEE Transactions on Instrumentation and Measurement (2023) Vol. 72, pp. 1-11
Closed Access | Times Cited: 10
A GAN-Based Multi-Sensor Data Augmentation Technique for CNC Machine Tool Wear Prediction
Yuechi Jiang, Benny Drescher, Guoguang Yuan
IEEE Access (2023) Vol. 11, pp. 95782-95795
Open Access | Times Cited: 9
Yuechi Jiang, Benny Drescher, Guoguang Yuan
IEEE Access (2023) Vol. 11, pp. 95782-95795
Open Access | Times Cited: 9
Machine learning coupled with acoustic emission signal features for tool wear estimation during ultrasonic machining of Inconel 718
Mehdi Mehtab Mirad, Bipul Das
Machining Science and Technology (2024) Vol. 28, Iss. 2, pp. 119-142
Closed Access | Times Cited: 3
Mehdi Mehtab Mirad, Bipul Das
Machining Science and Technology (2024) Vol. 28, Iss. 2, pp. 119-142
Closed Access | Times Cited: 3
Overview of Tool Wear Monitoring Methods Based on Convolutional Neural Network
Qun Wang, Hengsheng Wang, Liwei Hou, et al.
Applied Sciences (2021) Vol. 11, Iss. 24, pp. 12041-12041
Open Access | Times Cited: 21
Qun Wang, Hengsheng Wang, Liwei Hou, et al.
Applied Sciences (2021) Vol. 11, Iss. 24, pp. 12041-12041
Open Access | Times Cited: 21
Analysis of Spindle AE Signals and Development of AE-Based Tool Wear Monitoring System in Micro-Milling
Bing-Syun Wan, Ming‐Chyuan Lu, Shean-Juinn Chiou
Journal of Manufacturing and Materials Processing (2022) Vol. 6, Iss. 2, pp. 42-42
Open Access | Times Cited: 13
Bing-Syun Wan, Ming‐Chyuan Lu, Shean-Juinn Chiou
Journal of Manufacturing and Materials Processing (2022) Vol. 6, Iss. 2, pp. 42-42
Open Access | Times Cited: 13
Artificial intelligence and its relevance in mechanical engineering from Industry 4.0 perspective
P. K. Ambadekar, Sarita Ambadekar, Chandrashekhar Choudhari, et al.
Australian Journal of Mechanical Engineering (2023), pp. 1-21
Closed Access | Times Cited: 7
P. K. Ambadekar, Sarita Ambadekar, Chandrashekhar Choudhari, et al.
Australian Journal of Mechanical Engineering (2023), pp. 1-21
Closed Access | Times Cited: 7