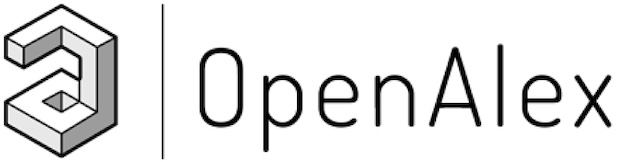
OpenAlex is a bibliographic catalogue of scientific papers, authors and institutions accessible in open access mode, named after the Library of Alexandria. It's citation coverage is excellent and I hope you will find utility in this listing of citing articles!
If you click the article title, you'll navigate to the article, as listed in CrossRef. If you click the Open Access links, you'll navigate to the "best Open Access location". Clicking the citation count will open this listing for that article. Lastly at the bottom of the page, you'll find basic pagination options.
Requested Article:
Physics-Informed Machine Learning—An Emerging Trend in Tribology
Max Marian, Stephan Tremmel
Lubricants (2023) Vol. 11, Iss. 11, pp. 463-463
Open Access | Times Cited: 13
Max Marian, Stephan Tremmel
Lubricants (2023) Vol. 11, Iss. 11, pp. 463-463
Open Access | Times Cited: 13
Showing 13 citing articles:
Physics-Informed Neural Network (PINN) for Solving Frictional Contact Temperature and Inversely Evaluating Relevant Input Parameters
Yichun Xia, Yonggang Meng
Lubricants (2024) Vol. 12, Iss. 2, pp. 62-62
Open Access | Times Cited: 6
Yichun Xia, Yonggang Meng
Lubricants (2024) Vol. 12, Iss. 2, pp. 62-62
Open Access | Times Cited: 6
Harnessing Machine Learning to Predict MoS2 Solid Lubricant Performance
Dayton J. Vogel, Tomas F. Babuska, Alexander Mings, et al.
Tribology Letters (2025) Vol. 73, Iss. 1
Closed Access
Dayton J. Vogel, Tomas F. Babuska, Alexander Mings, et al.
Tribology Letters (2025) Vol. 73, Iss. 1
Closed Access
Extrapolation of cavitation and hydrodynamic pressure in lubricated contacts: a physics-informed neural network approach
Faras Brumand‐Poor, Freddy Kokou Azanledji, Nils Plückhahn, et al.
Advanced Modeling and Simulation in Engineering Sciences (2025) Vol. 12, Iss. 1
Open Access
Faras Brumand‐Poor, Freddy Kokou Azanledji, Nils Plückhahn, et al.
Advanced Modeling and Simulation in Engineering Sciences (2025) Vol. 12, Iss. 1
Open Access
Simulation of lubrication on rough surfaces with multiscale lubrication neural networks
Yihu Tang, Li Huang, Limin Wu, et al.
Science China Technological Sciences (2025) Vol. 68, Iss. 3
Closed Access
Yihu Tang, Li Huang, Limin Wu, et al.
Science China Technological Sciences (2025) Vol. 68, Iss. 3
Closed Access
Rolling Bearing Dynamics Simulation Information-Assisted Fault Diagnosis with Multi-Adversarial Domain Transfer Learning
Zhe Li, Zhidan Zhong, Zhihui Zhang, et al.
Lubricants (2025) Vol. 13, Iss. 3, pp. 116-116
Open Access
Zhe Li, Zhidan Zhong, Zhihui Zhang, et al.
Lubricants (2025) Vol. 13, Iss. 3, pp. 116-116
Open Access
Extrapolation of Hydrodynamic Pressure in Lubricated Contacts: A Novel Multi-Case Physics-Informed Neural Network Framework
Faras Brumand‐Poor, Niklas Bauer, Nils Plückhahn, et al.
Lubricants (2024) Vol. 12, Iss. 4, pp. 122-122
Open Access | Times Cited: 3
Faras Brumand‐Poor, Niklas Bauer, Nils Plückhahn, et al.
Lubricants (2024) Vol. 12, Iss. 4, pp. 122-122
Open Access | Times Cited: 3
Application of machine learning for film thickness prediction in elliptical EHL contact with varying entrainment angle
Marko Tošić, Max Marian, Wassim Habchi, et al.
Tribology International (2024) Vol. 199, pp. 109940-109940
Open Access | Times Cited: 2
Marko Tošić, Max Marian, Wassim Habchi, et al.
Tribology International (2024) Vol. 199, pp. 109940-109940
Open Access | Times Cited: 2
Enhancing practical modeling: A neural network approach for locally-resolved prediction of elastohydrodynamic line contacts
Josephine Kelley, Volker Schneider, Gerhard Poll, et al.
Tribology International (2024) Vol. 199, pp. 109988-109988
Open Access | Times Cited: 2
Josephine Kelley, Volker Schneider, Gerhard Poll, et al.
Tribology International (2024) Vol. 199, pp. 109988-109988
Open Access | Times Cited: 2
A Neural Network for Fast Modeling of Elastohydrodynamic Line Contacts
Josephine Kelley, Volker Schneider, Max Marian, et al.
(2024)
Closed Access | Times Cited: 1
Josephine Kelley, Volker Schneider, Max Marian, et al.
(2024)
Closed Access | Times Cited: 1
A review of physics-informed machine learning for building energy modeling
Zhihao Ma, Gang Yi Jiang, Yuqing Hu, et al.
Applied Energy (2024) Vol. 381, pp. 125169-125169
Closed Access | Times Cited: 1
Zhihao Ma, Gang Yi Jiang, Yuqing Hu, et al.
Applied Energy (2024) Vol. 381, pp. 125169-125169
Closed Access | Times Cited: 1
Leveraging physics-informed neural networks for wavefield analysis in laser ultrasonic testing
Yang Li, Bin Xu, Yun Zou, et al.
Nondestructive Testing And Evaluation (2024), pp. 1-23
Closed Access | Times Cited: 1
Yang Li, Bin Xu, Yun Zou, et al.
Nondestructive Testing And Evaluation (2024), pp. 1-23
Closed Access | Times Cited: 1
Sealing rubber ring design based on machine learning algorithm combined progressive optimization method
Weiru Zhou, Zonghong Xie
Tribology International (2024) Vol. 201, pp. 110173-110173
Closed Access
Weiru Zhou, Zonghong Xie
Tribology International (2024) Vol. 201, pp. 110173-110173
Closed Access
Physics-Informed Neural Networks for the Reynolds Equation with Transient Cavitation Modeling
Faras Brumand‐Poor, Florian Barlog, Nils Plückhahn, et al.
Lubricants (2024) Vol. 12, Iss. 11, pp. 365-365
Open Access
Faras Brumand‐Poor, Florian Barlog, Nils Plückhahn, et al.
Lubricants (2024) Vol. 12, Iss. 11, pp. 365-365
Open Access