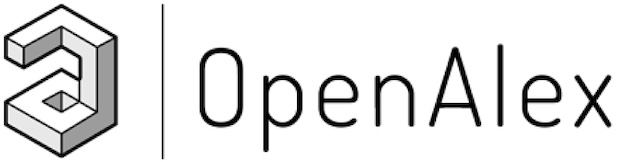
OpenAlex is a bibliographic catalogue of scientific papers, authors and institutions accessible in open access mode, named after the Library of Alexandria. It's citation coverage is excellent and I hope you will find utility in this listing of citing articles!
If you click the article title, you'll navigate to the article, as listed in CrossRef. If you click the Open Access links, you'll navigate to the "best Open Access location". Clicking the citation count will open this listing for that article. Lastly at the bottom of the page, you'll find basic pagination options.
Requested Article:
iEnhancer-EBLSTM: Identifying Enhancers and Strengths by Ensembles of Bidirectional Long Short-Term Memory
Kun Niu, Ximei Luo, Shumei Zhang, et al.
Frontiers in Genetics (2021) Vol. 12
Open Access | Times Cited: 31
Kun Niu, Ximei Luo, Shumei Zhang, et al.
Frontiers in Genetics (2021) Vol. 12
Open Access | Times Cited: 31
Showing 1-25 of 31 citing articles:
CLNN-loop: a deep learning model to predict CTCF-mediated chromatin loops in the different cell lines and CTCF-binding sites (CBS) pair types
Pengyu Zhang, Yingfu Wu, Haoru Zhou, et al.
Bioinformatics (2022) Vol. 38, Iss. 19, pp. 4497-4504
Closed Access | Times Cited: 45
Pengyu Zhang, Yingfu Wu, Haoru Zhou, et al.
Bioinformatics (2022) Vol. 38, Iss. 19, pp. 4497-4504
Closed Access | Times Cited: 45
ADH-Enhancer: an attention-based deep hybrid framework for enhancer identification and strength prediction
Faiza Mehmood, Shazia Arshad, Muhammad Shoaib
Briefings in Bioinformatics (2024) Vol. 25, Iss. 2
Open Access | Times Cited: 9
Faiza Mehmood, Shazia Arshad, Muhammad Shoaib
Briefings in Bioinformatics (2024) Vol. 25, Iss. 2
Open Access | Times Cited: 9
Integrative machine learning framework for the identification of cell-specific enhancers from the human genome
Shaherin Basith, Md Mehedi Hasan, Gwang Lee, et al.
Briefings in Bioinformatics (2021) Vol. 22, Iss. 6
Closed Access | Times Cited: 54
Shaherin Basith, Md Mehedi Hasan, Gwang Lee, et al.
Briefings in Bioinformatics (2021) Vol. 22, Iss. 6
Closed Access | Times Cited: 54
Prediction of Enhancers in DNA Sequence Data using a Hybrid CNN-DLSTM Model
Amandeep Kaur, Ajay Pal Singh Chauhan, Ashwani Kumar Aggarwal
IEEE/ACM Transactions on Computational Biology and Bioinformatics (2022) Vol. 20, Iss. 2, pp. 1327-1336
Closed Access | Times Cited: 32
Amandeep Kaur, Ajay Pal Singh Chauhan, Ashwani Kumar Aggarwal
IEEE/ACM Transactions on Computational Biology and Bioinformatics (2022) Vol. 20, Iss. 2, pp. 1327-1336
Closed Access | Times Cited: 32
iEnhancer-DCSV: Predicting enhancers and their strength based on DenseNet and improved convolutional block attention module
Jianhua Jia, Rufeng Lei, Lulu Qin, et al.
Frontiers in Genetics (2023) Vol. 14
Open Access | Times Cited: 17
Jianhua Jia, Rufeng Lei, Lulu Qin, et al.
Frontiers in Genetics (2023) Vol. 14
Open Access | Times Cited: 17
Enhancer-MDLF: a novel deep learning framework for identifying cell-specific enhancers
Yao Zhang, Pengyu Zhang, Hao Wu
Briefings in Bioinformatics (2024) Vol. 25, Iss. 2
Open Access | Times Cited: 6
Yao Zhang, Pengyu Zhang, Hao Wu
Briefings in Bioinformatics (2024) Vol. 25, Iss. 2
Open Access | Times Cited: 6
Utilizing a deep learning model based on BERT for identifying enhancers and their strength
Tong Wang, Mengqi Gao
PLoS ONE (2025) Vol. 20, Iss. 4, pp. e0320085-e0320085
Open Access
Tong Wang, Mengqi Gao
PLoS ONE (2025) Vol. 20, Iss. 4, pp. e0320085-e0320085
Open Access
im7G-DCT: A two-branch strategy model based on improved DenseNet and Transformer for m7G site prediction
Rufeng Lei, Jian Jia, Lulu Qin
Computational Biology and Chemistry (2025), pp. 108473-108473
Closed Access
Rufeng Lei, Jian Jia, Lulu Qin
Computational Biology and Chemistry (2025), pp. 108473-108473
Closed Access
Improving Enhancer Identification with a Multi-Classifier Stacked Ensemble Model
Bilal Ahmad Mir, Mobeen Ur Rehman, Hilal Tayara, et al.
Journal of Molecular Biology (2023) Vol. 435, Iss. 23, pp. 168314-168314
Closed Access | Times Cited: 10
Bilal Ahmad Mir, Mobeen Ur Rehman, Hilal Tayara, et al.
Journal of Molecular Biology (2023) Vol. 435, Iss. 23, pp. 168314-168314
Closed Access | Times Cited: 10
Unveiling the Potential Pattern Representation of RNA 5-Methyluridine Modification Sites Through a Novel Feature Fusion Model Leveraging Convolutional Neural Network and Tetranucleotide Composition
Waleed Alam, Muhammad Tahir, Shahid Hussain, et al.
IEEE Access (2024) Vol. 12, pp. 10023-10035
Open Access | Times Cited: 3
Waleed Alam, Muhammad Tahir, Shahid Hussain, et al.
IEEE Access (2024) Vol. 12, pp. 10023-10035
Open Access | Times Cited: 3
CapsEnhancer: An Effective Computational Framework for Identifying Enhancers Based on Chaos Game Representation and Capsule Network
Lantian Yao, Peilin Xie, Jiahui Guan, et al.
Journal of Chemical Information and Modeling (2024) Vol. 64, Iss. 14, pp. 5725-5736
Open Access | Times Cited: 3
Lantian Yao, Peilin Xie, Jiahui Guan, et al.
Journal of Chemical Information and Modeling (2024) Vol. 64, Iss. 14, pp. 5725-5736
Open Access | Times Cited: 3
iEnhancer-DCLA: using the original sequence to identify enhancers and their strength based on a deep learning framework
Meng Liao, Jianping Zhao, Jing Tian, et al.
BMC Bioinformatics (2022) Vol. 23, Iss. 1
Open Access | Times Cited: 14
Meng Liao, Jianping Zhao, Jing Tian, et al.
BMC Bioinformatics (2022) Vol. 23, Iss. 1
Open Access | Times Cited: 14
iEnhancer-SKNN: a stacking ensemble learning-based method for enhancer identification and classification using sequence information
Hao Wu, Mengdi Liu, Pengyu Zhang, et al.
Briefings in Functional Genomics (2023) Vol. 22, Iss. 3, pp. 302-311
Open Access | Times Cited: 8
Hao Wu, Mengdi Liu, Pengyu Zhang, et al.
Briefings in Functional Genomics (2023) Vol. 22, Iss. 3, pp. 302-311
Open Access | Times Cited: 8
Enhancer-LSTMAtt: A Bi-LSTM and Attention-Based Deep Learning Method for Enhancer Recognition
Guohua Huang, Wei Luo, Guiyang Zhang, et al.
Biomolecules (2022) Vol. 12, Iss. 7, pp. 995-995
Open Access | Times Cited: 13
Guohua Huang, Wei Luo, Guiyang Zhang, et al.
Biomolecules (2022) Vol. 12, Iss. 7, pp. 995-995
Open Access | Times Cited: 13
A comprehensive revisit of the machine‐learning tools developed for the identification of enhancers in the human genome
Le Thi Phan, Changmin Oh, Tao He, et al.
PROTEOMICS (2023) Vol. 23, Iss. 13-14
Closed Access | Times Cited: 5
Le Thi Phan, Changmin Oh, Tao He, et al.
PROTEOMICS (2023) Vol. 23, Iss. 13-14
Closed Access | Times Cited: 5
iEnhancer-DCSA: identifying enhancers via dual-scale convolution and spatial attention
Wenjun Wang, Qingyao Wu, Chunshan Li
BMC Genomics (2023) Vol. 24, Iss. 1
Open Access | Times Cited: 4
Wenjun Wang, Qingyao Wu, Chunshan Li
BMC Genomics (2023) Vol. 24, Iss. 1
Open Access | Times Cited: 4
A Novel Predictor for the Analysis and Prediction of Enhancers and Their Strength via Multi-View Features and Deep Forest
Mehwish Gill, Saeed Ahmed, Muhammad Kabir, et al.
Information (2023) Vol. 14, Iss. 12, pp. 636-636
Open Access | Times Cited: 4
Mehwish Gill, Saeed Ahmed, Muhammad Kabir, et al.
Information (2023) Vol. 14, Iss. 12, pp. 636-636
Open Access | Times Cited: 4
i5mC-DCGA: an improved hybrid network framework based on the CBAM attention mechanism for identifying promoter 5mC sites
Jianhua Jia, Rufeng Lei, Lulu Qin, et al.
BMC Genomics (2024) Vol. 25, Iss. 1
Open Access | Times Cited: 1
Jianhua Jia, Rufeng Lei, Lulu Qin, et al.
BMC Genomics (2024) Vol. 25, Iss. 1
Open Access | Times Cited: 1
iPro2L-DG: Hybrid network based on improved densenet and global attention mechanism for identifying promoter sequences
Rufeng Lei, Jianhua Jia, Lulu Qin, et al.
Heliyon (2024) Vol. 10, Iss. 6, pp. e27364-e27364
Open Access | Times Cited: 1
Rufeng Lei, Jianhua Jia, Lulu Qin, et al.
Heliyon (2024) Vol. 10, Iss. 6, pp. e27364-e27364
Open Access | Times Cited: 1
DeepDualEnhancer: A Dual-Feature Input DNABert Based Deep Learning Method for Enhancer Recognition
Tao Song, Haonan Song, Zhiyi Pan, et al.
International Journal of Molecular Sciences (2024) Vol. 25, Iss. 21, pp. 11744-11744
Open Access | Times Cited: 1
Tao Song, Haonan Song, Zhiyi Pan, et al.
International Journal of Molecular Sciences (2024) Vol. 25, Iss. 21, pp. 11744-11744
Open Access | Times Cited: 1
iEnhancer-MRBF: Identifying enhancers and their strength with a multiple Laplacian-regularized radial basis function network
Zhichao Xiao, Lizhuang Wang, Yijie Ding, et al.
Methods (2022) Vol. 208, pp. 1-8
Closed Access | Times Cited: 7
Zhichao Xiao, Lizhuang Wang, Yijie Ding, et al.
Methods (2022) Vol. 208, pp. 1-8
Closed Access | Times Cited: 7
Genome-wide identification and characterization of DNA enhancers with a stacked multivariate fusion framework
Yansong Wang, Zilong Hou, Yuning Yang, et al.
PLoS Computational Biology (2022) Vol. 18, Iss. 12, pp. e1010779-e1010779
Open Access | Times Cited: 7
Yansong Wang, Zilong Hou, Yuning Yang, et al.
PLoS Computational Biology (2022) Vol. 18, Iss. 12, pp. e1010779-e1010779
Open Access | Times Cited: 7
iEnhancer-DLRA: identification of enhancers and their strengths by a self-attention fusion strategy for local and global features
Li Zeng, Yang Liu, Zu‐Guo Yu, et al.
Briefings in Functional Genomics (2022) Vol. 21, Iss. 5, pp. 399-407
Closed Access | Times Cited: 6
Li Zeng, Yang Liu, Zu‐Guo Yu, et al.
Briefings in Functional Genomics (2022) Vol. 21, Iss. 5, pp. 399-407
Closed Access | Times Cited: 6
A deep learning based two-layer predictor to identify enhancers and their strength
Di Zhu, Wen Yang, Dali Xu, et al.
Methods (2023) Vol. 211, pp. 23-30
Closed Access | Times Cited: 3
Di Zhu, Wen Yang, Dali Xu, et al.
Methods (2023) Vol. 211, pp. 23-30
Closed Access | Times Cited: 3
AOPM: Application of Antioxidant Protein Classification Model in Predicting the Composition of Antioxidant Drugs
Yixiao Zhai, Jingyu Zhang, Tianjiao Zhang, et al.
Frontiers in Pharmacology (2022) Vol. 12
Open Access | Times Cited: 3
Yixiao Zhai, Jingyu Zhang, Tianjiao Zhang, et al.
Frontiers in Pharmacology (2022) Vol. 12
Open Access | Times Cited: 3