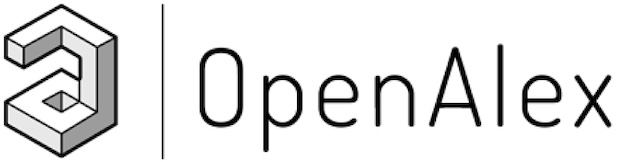
OpenAlex is a bibliographic catalogue of scientific papers, authors and institutions accessible in open access mode, named after the Library of Alexandria. It's citation coverage is excellent and I hope you will find utility in this listing of citing articles!
If you click the article title, you'll navigate to the article, as listed in CrossRef. If you click the Open Access links, you'll navigate to the "best Open Access location". Clicking the citation count will open this listing for that article. Lastly at the bottom of the page, you'll find basic pagination options.
Requested Article:
It’s Not Just Size That Matters: Small Language Models Are Also Few-Shot Learners
Timo Schick, Hinrich Schütze
Proceedings of the 2022 Conference of the North American Chapter of the Association for Computational Linguistics: Human Language Technologies (2021)
Open Access | Times Cited: 568
Timo Schick, Hinrich Schütze
Proceedings of the 2022 Conference of the North American Chapter of the Association for Computational Linguistics: Human Language Technologies (2021)
Open Access | Times Cited: 568
Showing 1-25 of 568 citing articles:
Pre-train, Prompt, and Predict: A Systematic Survey of Prompting Methods in Natural Language Processing
Pengfei Liu, Weizhe Yuan, Jinlan Fu, et al.
ACM Computing Surveys (2022) Vol. 55, Iss. 9, pp. 1-35
Open Access | Times Cited: 2013
Pengfei Liu, Weizhe Yuan, Jinlan Fu, et al.
ACM Computing Surveys (2022) Vol. 55, Iss. 9, pp. 1-35
Open Access | Times Cited: 2013
On the Opportunities and Risks of Foundation Models
Rishi Bommasani, Drew A. Hudson, Ehsan Adeli, et al.
arXiv (Cornell University) (2021)
Open Access | Times Cited: 1539
Rishi Bommasani, Drew A. Hudson, Ehsan Adeli, et al.
arXiv (Cornell University) (2021)
Open Access | Times Cited: 1539
Text Data Augmentation for Deep Learning
Connor Shorten, Taghi M. Khoshgoftaar, Borko Furht
Journal Of Big Data (2021) Vol. 8, Iss. 1
Open Access | Times Cited: 1420
Connor Shorten, Taghi M. Khoshgoftaar, Borko Furht
Journal Of Big Data (2021) Vol. 8, Iss. 1
Open Access | Times Cited: 1420
Making Pre-trained Language Models Better Few-shot Learners
Tianyu Gao, Adam Fisch, Danqi Chen
(2021)
Open Access | Times Cited: 1030
Tianyu Gao, Adam Fisch, Danqi Chen
(2021)
Open Access | Times Cited: 1030
Pre-trained models: Past, present and future
Xu Han, Zhengyan Zhang, Ning Ding, et al.
AI Open (2021) Vol. 2, pp. 225-250
Open Access | Times Cited: 636
Xu Han, Zhengyan Zhang, Ning Ding, et al.
AI Open (2021) Vol. 2, pp. 225-250
Open Access | Times Cited: 636
Recent Advances in Natural Language Processing via Large Pre-trained Language Models: A Survey
Bonan Min, Hayley Ross, Elior Sulem, et al.
ACM Computing Surveys (2023) Vol. 56, Iss. 2, pp. 1-40
Open Access | Times Cited: 558
Bonan Min, Hayley Ross, Elior Sulem, et al.
ACM Computing Surveys (2023) Vol. 56, Iss. 2, pp. 1-40
Open Access | Times Cited: 558
GLM: General Language Model Pretraining with Autoregressive Blank Infilling
Zhengxiao Du, Yujie Qian, Xiao Liu, et al.
Proceedings of the 60th Annual Meeting of the Association for Computational Linguistics (Volume 1: Long Papers) (2022)
Open Access | Times Cited: 515
Zhengxiao Du, Yujie Qian, Xiao Liu, et al.
Proceedings of the 60th Annual Meeting of the Association for Computational Linguistics (Volume 1: Long Papers) (2022)
Open Access | Times Cited: 515
P-Tuning: Prompt Tuning Can Be Comparable to Fine-tuning Across Scales and Tasks
Xiao Liu, Kaixuan Ji, Yicheng Fu, et al.
(2022)
Open Access | Times Cited: 456
Xiao Liu, Kaixuan Ji, Yicheng Fu, et al.
(2022)
Open Access | Times Cited: 456
Pre-trained models for natural language processing: A survey
Xipeng Qiu, Tianxiang Sun, Yige Xu, et al.
Science China Technological Sciences (2020) Vol. 63, Iss. 10, pp. 1872-1897
Closed Access | Times Cited: 439
Xipeng Qiu, Tianxiang Sun, Yige Xu, et al.
Science China Technological Sciences (2020) Vol. 63, Iss. 10, pp. 1872-1897
Closed Access | Times Cited: 439
Rethinking the Role of Demonstrations: What Makes In-Context Learning Work?
Sewon Min, Xinxi Lyu, Ari Holtzman, et al.
Proceedings of the 2021 Conference on Empirical Methods in Natural Language Processing (2022)
Open Access | Times Cited: 378
Sewon Min, Xinxi Lyu, Ari Holtzman, et al.
Proceedings of the 2021 Conference on Empirical Methods in Natural Language Processing (2022)
Open Access | Times Cited: 378
Deep Learning applications for COVID-19
Connor Shorten, Taghi M. Khoshgoftaar, Borko Furht
Journal Of Big Data (2021) Vol. 8, Iss. 1
Open Access | Times Cited: 304
Connor Shorten, Taghi M. Khoshgoftaar, Borko Furht
Journal Of Big Data (2021) Vol. 8, Iss. 1
Open Access | Times Cited: 304
Learning How to Ask: Querying LMs with Mixtures of Soft Prompts
Guanghui Qin, Jason Eisner
Proceedings of the 2022 Conference of the North American Chapter of the Association for Computational Linguistics: Human Language Technologies (2021)
Open Access | Times Cited: 286
Guanghui Qin, Jason Eisner
Proceedings of the 2022 Conference of the North American Chapter of the Association for Computational Linguistics: Human Language Technologies (2021)
Open Access | Times Cited: 286
Fantastically Ordered Prompts and Where to Find Them: Overcoming Few-Shot Prompt Order Sensitivity
Yao Lu, Max Bartolo, Alastair Moore, et al.
Proceedings of the 60th Annual Meeting of the Association for Computational Linguistics (Volume 1: Long Papers) (2022)
Open Access | Times Cited: 251
Yao Lu, Max Bartolo, Alastair Moore, et al.
Proceedings of the 60th Annual Meeting of the Association for Computational Linguistics (Volume 1: Long Papers) (2022)
Open Access | Times Cited: 251
A Comprehensive Survey of Few-shot Learning: Evolution, Applications, Challenges, and Opportunities
Yisheng Song, Ting Wang, Puyu Cai, et al.
ACM Computing Surveys (2023) Vol. 55, Iss. 13s, pp. 1-40
Open Access | Times Cited: 236
Yisheng Song, Ting Wang, Puyu Cai, et al.
ACM Computing Surveys (2023) Vol. 55, Iss. 13s, pp. 1-40
Open Access | Times Cited: 236
GPT understands, too
Xiao Liu, Yanan Zheng, Zhengxiao Du, et al.
AI Open (2023) Vol. 5, pp. 208-215
Open Access | Times Cited: 236
Xiao Liu, Yanan Zheng, Zhengxiao Du, et al.
AI Open (2023) Vol. 5, pp. 208-215
Open Access | Times Cited: 236
WARP: Word-level Adversarial ReProgramming
Karen Hambardzumyan, Hrant Khachatrian, Jonathan May
(2021)
Open Access | Times Cited: 212
Karen Hambardzumyan, Hrant Khachatrian, Jonathan May
(2021)
Open Access | Times Cited: 212
Factual Probing Is [MASK]: Learning vs. Learning to Recall
Zexuan Zhong, Dan Friedman, Danqi Chen
Proceedings of the 2022 Conference of the North American Chapter of the Association for Computational Linguistics: Human Language Technologies (2021)
Open Access | Times Cited: 205
Zexuan Zhong, Dan Friedman, Danqi Chen
Proceedings of the 2022 Conference of the North American Chapter of the Association for Computational Linguistics: Human Language Technologies (2021)
Open Access | Times Cited: 205
A Survey on Recent Approaches for Natural Language Processing in Low-Resource Scenarios
Michael A. Hedderich, Lukas Lange, Heike Adel, et al.
Proceedings of the 2022 Conference of the North American Chapter of the Association for Computational Linguistics: Human Language Technologies (2021)
Open Access | Times Cited: 191
Michael A. Hedderich, Lukas Lange, Heike Adel, et al.
Proceedings of the 2022 Conference of the North American Chapter of the Association for Computational Linguistics: Human Language Technologies (2021)
Open Access | Times Cited: 191
Knowledgeable Prompt-tuning: Incorporating Knowledge into Prompt Verbalizer for Text Classification
Shengding Hu, Ning Ding, Huadong Wang, et al.
Proceedings of the 60th Annual Meeting of the Association for Computational Linguistics (Volume 1: Long Papers) (2022)
Open Access | Times Cited: 175
Shengding Hu, Ning Ding, Huadong Wang, et al.
Proceedings of the 60th Annual Meeting of the Association for Computational Linguistics (Volume 1: Long Papers) (2022)
Open Access | Times Cited: 175
Self-Diagnosis and Self-Debiasing: A Proposal for Reducing Corpus-Based Bias in NLP
Timo Schick, Sahana Udupa, Hinrich Schütze
Transactions of the Association for Computational Linguistics (2021) Vol. 9, pp. 1408-1424
Open Access | Times Cited: 173
Timo Schick, Sahana Udupa, Hinrich Schütze
Transactions of the Association for Computational Linguistics (2021) Vol. 9, pp. 1408-1424
Open Access | Times Cited: 173
PPT: Pre-trained Prompt Tuning for Few-shot Learning
Yuxian Gu, Xu Han, Zhiyuan Liu, et al.
Proceedings of the 60th Annual Meeting of the Association for Computational Linguistics (Volume 1: Long Papers) (2022)
Open Access | Times Cited: 168
Yuxian Gu, Xu Han, Zhiyuan Liu, et al.
Proceedings of the 60th Annual Meeting of the Association for Computational Linguistics (Volume 1: Long Papers) (2022)
Open Access | Times Cited: 168
How many data points is a prompt worth?
Teven Le Scao, Alexander M. Rush
Proceedings of the 2022 Conference of the North American Chapter of the Association for Computational Linguistics: Human Language Technologies (2021)
Open Access | Times Cited: 164
Teven Le Scao, Alexander M. Rush
Proceedings of the 2022 Conference of the North American Chapter of the Association for Computational Linguistics: Human Language Technologies (2021)
Open Access | Times Cited: 164
Parameter-Efficient Transfer Learning with Diff Pruning
Demi Guo, Alexander M. Rush, Yoon Kim
(2021)
Open Access | Times Cited: 151
Demi Guo, Alexander M. Rush, Yoon Kim
(2021)
Open Access | Times Cited: 151
Structured Prediction as Translation between Augmented Natural Languages
Giovanni Paolini, Ben Athiwaratkun, Jason Krone, et al.
(2021)
Closed Access | Times Cited: 142
Giovanni Paolini, Ben Athiwaratkun, Jason Krone, et al.
(2021)
Closed Access | Times Cited: 142
Do Prompt-Based Models Really Understand the Meaning of Their Prompts?
Albert Webson, Ellie Pavlick
Proceedings of the 2022 Conference of the North American Chapter of the Association for Computational Linguistics: Human Language Technologies (2022)
Open Access | Times Cited: 137
Albert Webson, Ellie Pavlick
Proceedings of the 2022 Conference of the North American Chapter of the Association for Computational Linguistics: Human Language Technologies (2022)
Open Access | Times Cited: 137