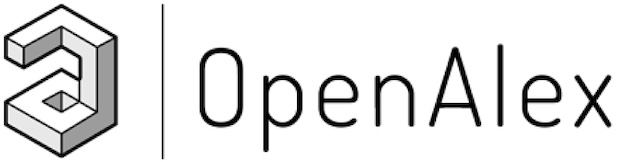
OpenAlex is a bibliographic catalogue of scientific papers, authors and institutions accessible in open access mode, named after the Library of Alexandria. It's citation coverage is excellent and I hope you will find utility in this listing of citing articles!
If you click the article title, you'll navigate to the article, as listed in CrossRef. If you click the Open Access links, you'll navigate to the "best Open Access location". Clicking the citation count will open this listing for that article. Lastly at the bottom of the page, you'll find basic pagination options.
Requested Article:
AutoPrompt: Eliciting Knowledge from Language Models with Automatically Generated Prompts
Taylor Shin, Yasaman Razeghi, Robert L. Logan, et al.
(2020)
Open Access | Times Cited: 879
Taylor Shin, Yasaman Razeghi, Robert L. Logan, et al.
(2020)
Open Access | Times Cited: 879
Showing 1-25 of 879 citing articles:
Pre-train, Prompt, and Predict: A Systematic Survey of Prompting Methods in Natural Language Processing
Pengfei Liu, Weizhe Yuan, Jinlan Fu, et al.
ACM Computing Surveys (2022) Vol. 55, Iss. 9, pp. 1-35
Open Access | Times Cited: 2013
Pengfei Liu, Weizhe Yuan, Jinlan Fu, et al.
ACM Computing Surveys (2022) Vol. 55, Iss. 9, pp. 1-35
Open Access | Times Cited: 2013
The Power of Scale for Parameter-Efficient Prompt Tuning
Brian Lester, Rami Al‐Rfou, Noah Constant
Proceedings of the 2021 Conference on Empirical Methods in Natural Language Processing (2021)
Open Access | Times Cited: 1606
Brian Lester, Rami Al‐Rfou, Noah Constant
Proceedings of the 2021 Conference on Empirical Methods in Natural Language Processing (2021)
Open Access | Times Cited: 1606
Prefix-Tuning: Optimizing Continuous Prompts for Generation
Xiang Lisa Li, Percy Liang
(2021)
Open Access | Times Cited: 1551
Xiang Lisa Li, Percy Liang
(2021)
Open Access | Times Cited: 1551
On the Opportunities and Risks of Foundation Models
Rishi Bommasani, Drew A. Hudson, Ehsan Adeli, et al.
arXiv (Cornell University) (2021)
Open Access | Times Cited: 1539
Rishi Bommasani, Drew A. Hudson, Ehsan Adeli, et al.
arXiv (Cornell University) (2021)
Open Access | Times Cited: 1539
Learning to Prompt for Vision-Language Models
Kaiyang Zhou, Jingkang Yang, Chen Change Loy, et al.
International Journal of Computer Vision (2022) Vol. 130, Iss. 9, pp. 2337-2348
Closed Access | Times Cited: 1170
Kaiyang Zhou, Jingkang Yang, Chen Change Loy, et al.
International Journal of Computer Vision (2022) Vol. 130, Iss. 9, pp. 2337-2348
Closed Access | Times Cited: 1170
Conditional Prompt Learning for Vision-Language Models
Kaiyang Zhou, Jingkang Yang, Chen Change Loy, et al.
2022 IEEE/CVF Conference on Computer Vision and Pattern Recognition (CVPR) (2022)
Open Access | Times Cited: 690
Kaiyang Zhou, Jingkang Yang, Chen Change Loy, et al.
2022 IEEE/CVF Conference on Computer Vision and Pattern Recognition (CVPR) (2022)
Open Access | Times Cited: 690
Pre-trained models: Past, present and future
Xu Han, Zhengyan Zhang, Ning Ding, et al.
AI Open (2021) Vol. 2, pp. 225-250
Open Access | Times Cited: 636
Xu Han, Zhengyan Zhang, Ning Ding, et al.
AI Open (2021) Vol. 2, pp. 225-250
Open Access | Times Cited: 636
Visual Prompt Tuning
Menglin Jia, Luming Tang, Bor-Chun Chen, et al.
Lecture notes in computer science (2022), pp. 709-727
Closed Access | Times Cited: 576
Menglin Jia, Luming Tang, Bor-Chun Chen, et al.
Lecture notes in computer science (2022), pp. 709-727
Closed Access | Times Cited: 576
Recent Advances in Natural Language Processing via Large Pre-trained Language Models: A Survey
Bonan Min, Hayley Ross, Elior Sulem, et al.
ACM Computing Surveys (2023) Vol. 56, Iss. 2, pp. 1-40
Open Access | Times Cited: 558
Bonan Min, Hayley Ross, Elior Sulem, et al.
ACM Computing Surveys (2023) Vol. 56, Iss. 2, pp. 1-40
Open Access | Times Cited: 558
Grounded Language-Image Pre-training
Liunian Harold Li, Pengchuan Zhang, Haotian Zhang, et al.
2022 IEEE/CVF Conference on Computer Vision and Pattern Recognition (CVPR) (2022), pp. 10955-10965
Open Access | Times Cited: 467
Liunian Harold Li, Pengchuan Zhang, Haotian Zhang, et al.
2022 IEEE/CVF Conference on Computer Vision and Pattern Recognition (CVPR) (2022), pp. 10955-10965
Open Access | Times Cited: 467
P-Tuning: Prompt Tuning Can Be Comparable to Fine-tuning Across Scales and Tasks
Xiao Liu, Kaixuan Ji, Yicheng Fu, et al.
(2022)
Open Access | Times Cited: 456
Xiao Liu, Kaixuan Ji, Yicheng Fu, et al.
(2022)
Open Access | Times Cited: 456
Pre-trained models for natural language processing: A survey
Xipeng Qiu, Tianxiang Sun, Yige Xu, et al.
Science China Technological Sciences (2020) Vol. 63, Iss. 10, pp. 1872-1897
Closed Access | Times Cited: 439
Xipeng Qiu, Tianxiang Sun, Yige Xu, et al.
Science China Technological Sciences (2020) Vol. 63, Iss. 10, pp. 1872-1897
Closed Access | Times Cited: 439
CLIP-Adapter: Better Vision-Language Models with Feature Adapters
Peng Gao, Shijie Geng, Renrui Zhang, et al.
International Journal of Computer Vision (2023) Vol. 132, Iss. 2, pp. 581-595
Closed Access | Times Cited: 330
Peng Gao, Shijie Geng, Renrui Zhang, et al.
International Journal of Computer Vision (2023) Vol. 132, Iss. 2, pp. 581-595
Closed Access | Times Cited: 330
Unifying Large Language Models and Knowledge Graphs: A Roadmap
Shirui Pan, Linhao Luo, Yufei Wang, et al.
IEEE Transactions on Knowledge and Data Engineering (2024) Vol. 36, Iss. 7, pp. 3580-3599
Closed Access | Times Cited: 297
Shirui Pan, Linhao Luo, Yufei Wang, et al.
IEEE Transactions on Knowledge and Data Engineering (2024) Vol. 36, Iss. 7, pp. 3580-3599
Closed Access | Times Cited: 297
What Makes Good In-Context Examples for GPT-3?
Jiachang Liu, Dinghan Shen, Yizhe Zhang, et al.
(2022)
Open Access | Times Cited: 296
Jiachang Liu, Dinghan Shen, Yizhe Zhang, et al.
(2022)
Open Access | Times Cited: 296
Learning How to Ask: Querying LMs with Mixtures of Soft Prompts
Guanghui Qin, Jason Eisner
Proceedings of the 2022 Conference of the North American Chapter of the Association for Computational Linguistics: Human Language Technologies (2021)
Open Access | Times Cited: 286
Guanghui Qin, Jason Eisner
Proceedings of the 2022 Conference of the North American Chapter of the Association for Computational Linguistics: Human Language Technologies (2021)
Open Access | Times Cited: 286
KnowPrompt: Knowledge-aware Prompt-tuning with Synergistic Optimization for Relation Extraction
Xiang Chen, Ningyu Zhang, Xin Xie, et al.
Proceedings of the ACM Web Conference 2022 (2022), pp. 2778-2788
Open Access | Times Cited: 279
Xiang Chen, Ningyu Zhang, Xin Xie, et al.
Proceedings of the ACM Web Conference 2022 (2022), pp. 2778-2788
Open Access | Times Cited: 279
Fantastically Ordered Prompts and Where to Find Them: Overcoming Few-Shot Prompt Order Sensitivity
Yao Lu, Max Bartolo, Alastair Moore, et al.
Proceedings of the 60th Annual Meeting of the Association for Computational Linguistics (Volume 1: Long Papers) (2022)
Open Access | Times Cited: 251
Yao Lu, Max Bartolo, Alastair Moore, et al.
Proceedings of the 60th Annual Meeting of the Association for Computational Linguistics (Volume 1: Long Papers) (2022)
Open Access | Times Cited: 251
A Comprehensive Survey of Few-shot Learning: Evolution, Applications, Challenges, and Opportunities
Yisheng Song, Ting Wang, Puyu Cai, et al.
ACM Computing Surveys (2023) Vol. 55, Iss. 13s, pp. 1-40
Open Access | Times Cited: 236
Yisheng Song, Ting Wang, Puyu Cai, et al.
ACM Computing Surveys (2023) Vol. 55, Iss. 13s, pp. 1-40
Open Access | Times Cited: 236
GPT understands, too
Xiao Liu, Yanan Zheng, Zhengxiao Du, et al.
AI Open (2023) Vol. 5, pp. 208-215
Open Access | Times Cited: 236
Xiao Liu, Yanan Zheng, Zhengxiao Du, et al.
AI Open (2023) Vol. 5, pp. 208-215
Open Access | Times Cited: 236
WARP: Word-level Adversarial ReProgramming
Karen Hambardzumyan, Hrant Khachatrian, Jonathan May
(2021)
Open Access | Times Cited: 212
Karen Hambardzumyan, Hrant Khachatrian, Jonathan May
(2021)
Open Access | Times Cited: 212
Learning To Retrieve Prompts for In-Context Learning
Ohad Rubin, Jonathan Herzig, Jonathan Berant
Proceedings of the 2022 Conference of the North American Chapter of the Association for Computational Linguistics: Human Language Technologies (2022)
Open Access | Times Cited: 207
Ohad Rubin, Jonathan Herzig, Jonathan Berant
Proceedings of the 2022 Conference of the North American Chapter of the Association for Computational Linguistics: Human Language Technologies (2022)
Open Access | Times Cited: 207
AI Chains: Transparent and Controllable Human-AI Interaction by Chaining Large Language Model Prompts
Tongshuang Wu, Michael Terry, Carrie J. Cai
CHI Conference on Human Factors in Computing Systems (2022), pp. 1-22
Open Access | Times Cited: 199
Tongshuang Wu, Michael Terry, Carrie J. Cai
CHI Conference on Human Factors in Computing Systems (2022), pp. 1-22
Open Access | Times Cited: 199
Prompting Visual-Language Models for Efficient Video Understanding
Chen Ju, Tengda Han, Kunhao Zheng, et al.
Lecture notes in computer science (2022), pp. 105-124
Closed Access | Times Cited: 196
Chen Ju, Tengda Han, Kunhao Zheng, et al.
Lecture notes in computer science (2022), pp. 105-124
Closed Access | Times Cited: 196
True Few-Shot Learning with Language Models
Ethan Perez, Douwe Kiela, Kyunghyun Cho
arXiv (Cornell University) (2021)
Open Access | Times Cited: 179
Ethan Perez, Douwe Kiela, Kyunghyun Cho
arXiv (Cornell University) (2021)
Open Access | Times Cited: 179