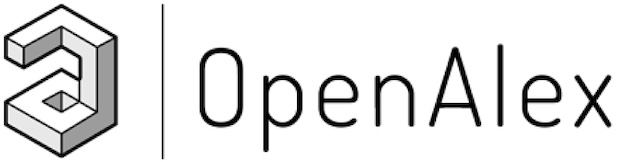
OpenAlex is a bibliographic catalogue of scientific papers, authors and institutions accessible in open access mode, named after the Library of Alexandria. It's citation coverage is excellent and I hope you will find utility in this listing of citing articles!
If you click the article title, you'll navigate to the article, as listed in CrossRef. If you click the Open Access links, you'll navigate to the "best Open Access location". Clicking the citation count will open this listing for that article. Lastly at the bottom of the page, you'll find basic pagination options.
Requested Article:
Expert-augmented automated machine learning optimizes hemodynamic predictors of spinal cord injury outcome
Austin Chou, Abel Torres‐Espín, Nikos Kyritsis, et al.
PLoS ONE (2022) Vol. 17, Iss. 4, pp. e0265254-e0265254
Open Access | Times Cited: 16
Austin Chou, Abel Torres‐Espín, Nikos Kyritsis, et al.
PLoS ONE (2022) Vol. 17, Iss. 4, pp. e0265254-e0265254
Open Access | Times Cited: 16
Showing 16 citing articles:
Automated machine learning with interpretation: A systematic review of methodologies and applications in healthcare
Han Yuan, Kunyu Yu, Feng Xie, et al.
Medicine Advances (2024) Vol. 2, Iss. 3, pp. 205-237
Open Access | Times Cited: 11
Han Yuan, Kunyu Yu, Feng Xie, et al.
Medicine Advances (2024) Vol. 2, Iss. 3, pp. 205-237
Open Access | Times Cited: 11
Machine learning in clinical diagnosis, prognostication, and management of acute traumatic spinal cord injury (SCI): A systematic review
Nicholas Dietz, Vaitheesh L. Jaganathan, Victoria Alkin, et al.
Journal of Clinical Orthopaedics and Trauma (2022) Vol. 35, pp. 102046-102046
Open Access | Times Cited: 32
Nicholas Dietz, Vaitheesh L. Jaganathan, Victoria Alkin, et al.
Journal of Clinical Orthopaedics and Trauma (2022) Vol. 35, pp. 102046-102046
Open Access | Times Cited: 32
Towards explainable artificial intelligence through expert-augmented supervised feature selection
Meysam Rabiee, Mohsen Mirhashemi, Michael S. Pangburn, et al.
Decision Support Systems (2024) Vol. 181, pp. 114214-114214
Closed Access | Times Cited: 5
Meysam Rabiee, Mohsen Mirhashemi, Michael S. Pangburn, et al.
Decision Support Systems (2024) Vol. 181, pp. 114214-114214
Closed Access | Times Cited: 5
Enhancing Bacterial Phenotype Classification Through the Integration of Autogating and Automated Machine Learning in Flow Cytometric Analysis
In Jae Jeong, Jin‐Kyung Hong, Y. Bae, et al.
Cytometry Part A (2025)
Open Access
In Jae Jeong, Jin‐Kyung Hong, Y. Bae, et al.
Cytometry Part A (2025)
Open Access
Predicting the Outcome and Survival of Patients with Spinal Cord Injury Using Machine Learning Algorithms: A Systematic Review
Mohammad Amin Habibi, Seyed Ahmad Naseri Alavi, Ali Soltani Farsani, et al.
World Neurosurgery (2024) Vol. 188, pp. 150-160
Closed Access | Times Cited: 4
Mohammad Amin Habibi, Seyed Ahmad Naseri Alavi, Ali Soltani Farsani, et al.
World Neurosurgery (2024) Vol. 188, pp. 150-160
Closed Access | Times Cited: 4
Data-driven prediction of spinal cord injury recovery: An exploration of current status and future perspectives
Samuel Håkansson, Miklovana Tuci, Marc Bolliger, et al.
Experimental Neurology (2024) Vol. 380, pp. 114913-114913
Open Access | Times Cited: 4
Samuel Håkansson, Miklovana Tuci, Marc Bolliger, et al.
Experimental Neurology (2024) Vol. 380, pp. 114913-114913
Open Access | Times Cited: 4
Spinal Cord Injury AIS Predictions Using Machine Learning
Dhruv Kapoor, Clark Xu
eNeuro (2022) Vol. 10, Iss. 1, pp. ENEURO.0149-22.2022
Open Access | Times Cited: 18
Dhruv Kapoor, Clark Xu
eNeuro (2022) Vol. 10, Iss. 1, pp. ENEURO.0149-22.2022
Open Access | Times Cited: 18
The Impact of Machine Learning and Robot-Assisted Gait Training on Spinal Cord Injury: A Systematic Review and Meta-Analysis
Dewa Putu Wisnu Wardhana, Sri Maliawan, Tjokorda Gde Bagus Mahadewa, et al.
Journal of Clinical Medicine (2023) Vol. 12, Iss. 23, pp. 7230-7230
Open Access | Times Cited: 6
Dewa Putu Wisnu Wardhana, Sri Maliawan, Tjokorda Gde Bagus Mahadewa, et al.
Journal of Clinical Medicine (2023) Vol. 12, Iss. 23, pp. 7230-7230
Open Access | Times Cited: 6
Automated Machine Learning Analysis of Patients With Chronic Skin Disease Using a Medical Smartphone App: Retrospective Study
Igor Bibi, Daniel Schaffert, Mara Blauth, et al.
Journal of Medical Internet Research (2023) Vol. 25, pp. e50886-e50886
Open Access | Times Cited: 4
Igor Bibi, Daniel Schaffert, Mara Blauth, et al.
Journal of Medical Internet Research (2023) Vol. 25, pp. e50886-e50886
Open Access | Times Cited: 4
Data-driven prediction of spinal cord injury recovery: an exploration of current status and future perspectives
Samuel Håkansson, Miklovana Tuci, Marc Bolliger, et al.
medRxiv (Cold Spring Harbor Laboratory) (2024)
Open Access | Times Cited: 1
Samuel Håkansson, Miklovana Tuci, Marc Bolliger, et al.
medRxiv (Cold Spring Harbor Laboratory) (2024)
Open Access | Times Cited: 1
Towards Augmented Financial Intelligence
Anna-Helena Mihov, Nick Firoozye, Philip Treleaven
SSRN Electronic Journal (2022)
Closed Access | Times Cited: 5
Anna-Helena Mihov, Nick Firoozye, Philip Treleaven
SSRN Electronic Journal (2022)
Closed Access | Times Cited: 5
Clinical performance of automated machine learning: a systematic review
Arun James Thirunavukarasu, Kabilan Elangovan, Laura Gutiérrez, et al.
medRxiv (Cold Spring Harbor Laboratory) (2023)
Open Access | Times Cited: 2
Arun James Thirunavukarasu, Kabilan Elangovan, Laura Gutiérrez, et al.
medRxiv (Cold Spring Harbor Laboratory) (2023)
Open Access | Times Cited: 2
Machine Learning Prediction of Self-Injurious Outcomes in Adolescents by Sexual and Gender Identity
Nadia Kako, Juno B. Pinder, John Powers, et al.
Archives of Suicide Research (2024), pp. 1-14
Closed Access
Nadia Kako, Juno B. Pinder, John Powers, et al.
Archives of Suicide Research (2024), pp. 1-14
Closed Access
Quantifying the Risk of General Health and Early COVID-19 Spread in Residential Buildings with Deep Learning and Expert-augmented Machine Learning
Jingjing Guan, Eman Leung, Kin On Kwok, et al.
medRxiv (Cold Spring Harbor Laboratory) (2023)
Open Access
Jingjing Guan, Eman Leung, Kin On Kwok, et al.
medRxiv (Cold Spring Harbor Laboratory) (2023)
Open Access
Automated Machine Learning Analysis of Patients With Chronic Skin Disease Using a Medical Smartphone App: Retrospective Study (Preprint)
Igor Bibi, Daniel Schaffert, Mara Blauth, et al.
(2023)
Open Access
Igor Bibi, Daniel Schaffert, Mara Blauth, et al.
(2023)
Open Access
Correction: Expert-augmented automated machine learning optimizes hemodynamic predictors of spinal cord injury outcome
Austin Chou, Abel Torres‐Espín, Nikos Kyritsis, et al.
PLoS ONE (2023) Vol. 18, Iss. 11, pp. e0294081-e0294081
Open Access
Austin Chou, Abel Torres‐Espín, Nikos Kyritsis, et al.
PLoS ONE (2023) Vol. 18, Iss. 11, pp. e0294081-e0294081
Open Access