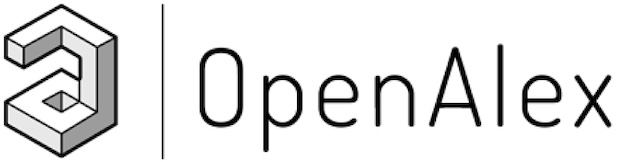
OpenAlex is a bibliographic catalogue of scientific papers, authors and institutions accessible in open access mode, named after the Library of Alexandria. It's citation coverage is excellent and I hope you will find utility in this listing of citing articles!
If you click the article title, you'll navigate to the article, as listed in CrossRef. If you click the Open Access links, you'll navigate to the "best Open Access location". Clicking the citation count will open this listing for that article. Lastly at the bottom of the page, you'll find basic pagination options.
Requested Article:
Convolutional neural networks as aid in core lithofacies classification
Rafael Pires de Lima, Fnu Suriamin, Kurt J. Marfurt, et al.
Interpretation (2019) Vol. 7, Iss. 3, pp. SF27-SF40
Closed Access | Times Cited: 53
Rafael Pires de Lima, Fnu Suriamin, Kurt J. Marfurt, et al.
Interpretation (2019) Vol. 7, Iss. 3, pp. SF27-SF40
Closed Access | Times Cited: 53
Showing 1-25 of 53 citing articles:
Convolutional Neural Network for Remote-Sensing Scene Classification: Transfer Learning Analysis
Rafael Pires de Lima, Kurt J. Marfurt
Remote Sensing (2019) Vol. 12, Iss. 1, pp. 86-86
Open Access | Times Cited: 226
Rafael Pires de Lima, Kurt J. Marfurt
Remote Sensing (2019) Vol. 12, Iss. 1, pp. 86-86
Open Access | Times Cited: 226
Impact of dataset size and convolutional neural network architecture on transfer learning for carbonate rock classification
Harriet L. Dawson, Olivier Dubrule, Cédric M. John
Computers & Geosciences (2022) Vol. 171, pp. 105284-105284
Open Access | Times Cited: 66
Harriet L. Dawson, Olivier Dubrule, Cédric M. John
Computers & Geosciences (2022) Vol. 171, pp. 105284-105284
Open Access | Times Cited: 66
Evaluation and Development of a Predictive Model for Geophysical Well Log Data Analysis and Reservoir Characterization: Machine Learning Applications to Lithology Prediction
Aditya Mishra, Anjali Sharma, Atul Kumar Patidar
Natural Resources Research (2022) Vol. 31, Iss. 6, pp. 3195-3222
Closed Access | Times Cited: 52
Aditya Mishra, Anjali Sharma, Atul Kumar Patidar
Natural Resources Research (2022) Vol. 31, Iss. 6, pp. 3195-3222
Closed Access | Times Cited: 52
Performance evaluation of boosting machine learning algorithms for lithofacies classification in heterogeneous carbonate reservoirs
Watheq J. Al‐Mudhafar, Mohammed A. Abbas, David A. Wood
Marine and Petroleum Geology (2022) Vol. 145, pp. 105886-105886
Closed Access | Times Cited: 50
Watheq J. Al‐Mudhafar, Mohammed A. Abbas, David A. Wood
Marine and Petroleum Geology (2022) Vol. 145, pp. 105886-105886
Closed Access | Times Cited: 50
Fully automated carbonate petrography using deep convolutional neural networks
Ardiansyah Koeshidayatullah, Michele Morsilli, Daniel J. Lehrmann, et al.
Marine and Petroleum Geology (2020) Vol. 122, pp. 104687-104687
Open Access | Times Cited: 63
Ardiansyah Koeshidayatullah, Michele Morsilli, Daniel J. Lehrmann, et al.
Marine and Petroleum Geology (2020) Vol. 122, pp. 104687-104687
Open Access | Times Cited: 63
Petrographic microfacies classification with deep convolutional neural networks
Rafael Pires de Lima, D. Duarte, Charles Nicholson, et al.
Computers & Geosciences (2020) Vol. 142, pp. 104481-104481
Closed Access | Times Cited: 60
Rafael Pires de Lima, D. Duarte, Charles Nicholson, et al.
Computers & Geosciences (2020) Vol. 142, pp. 104481-104481
Closed Access | Times Cited: 60
Estimation of reservoir porosity based on seismic inversion results using deep learning methods
Runhai Feng
Journal of Natural Gas Science and Engineering (2020) Vol. 77, pp. 103270-103270
Closed Access | Times Cited: 51
Runhai Feng
Journal of Natural Gas Science and Engineering (2020) Vol. 77, pp. 103270-103270
Closed Access | Times Cited: 51
Automatic identification of fossils and abiotic grains during carbonate microfacies analysis using deep convolutional neural networks
Xiaokang Liu, Haijun Song
Sedimentary Geology (2020) Vol. 410, pp. 105790-105790
Open Access | Times Cited: 42
Xiaokang Liu, Haijun Song
Sedimentary Geology (2020) Vol. 410, pp. 105790-105790
Open Access | Times Cited: 42
Forecasting Megaelectron‐Volt Electrons Inside Earth's Outer Radiation Belt: PreMevE 2.0 Based on Supervised Machine Learning Algorithms
Rafael Pires de Lima, Yue Chen, Youzuo Lin
Space Weather (2020) Vol. 18, Iss. 2
Open Access | Times Cited: 41
Rafael Pires de Lima, Yue Chen, Youzuo Lin
Space Weather (2020) Vol. 18, Iss. 2
Open Access | Times Cited: 41
FaciesViT: Vision transformer for an improved core lithofacies prediction
Ardiansyah Koeshidayatullah, Sadam Al-Azani, E. E. Baraboshkin, et al.
Frontiers in Earth Science (2022) Vol. 10
Open Access | Times Cited: 23
Ardiansyah Koeshidayatullah, Sadam Al-Azani, E. E. Baraboshkin, et al.
Frontiers in Earth Science (2022) Vol. 10
Open Access | Times Cited: 23
Machine learning model interpretability using SHAP values: Application to a seismic facies classification task
David Lubo-Robles, Deepak Devegowda, Vikram Jayaram, et al.
(2020), pp. 1460-1464
Closed Access | Times Cited: 32
David Lubo-Robles, Deepak Devegowda, Vikram Jayaram, et al.
(2020), pp. 1460-1464
Closed Access | Times Cited: 32
Centimeter-Scale Lithology and Facies Prediction in Cored Wells Using Machine Learning
Thomas Martin, Ross Meyer, Zane Jobe
Frontiers in Earth Science (2021) Vol. 9
Open Access | Times Cited: 29
Thomas Martin, Ross Meyer, Zane Jobe
Frontiers in Earth Science (2021) Vol. 9
Open Access | Times Cited: 29
PCA-VGG16 model for classification of rock types
Yan Zhang, Yulong Ye, Dao-Jing Guo, et al.
Earth Science Informatics (2024) Vol. 17, Iss. 2, pp. 1553-1567
Closed Access | Times Cited: 4
Yan Zhang, Yulong Ye, Dao-Jing Guo, et al.
Earth Science Informatics (2024) Vol. 17, Iss. 2, pp. 1553-1567
Closed Access | Times Cited: 4
CONVOLUTIONAL NEURAL NETWORKS AS AN AID TO BIOSTRATIGRAPHY AND MICROPALEONTOLOGY: A TEST ON LATE PALEOZOIC MICROFOSSILS
Rafael Pires de Lima, Katie F. Welch, James E. Barrick, et al.
Palaios (2020) Vol. 35, Iss. 9, pp. 391-402
Closed Access | Times Cited: 29
Rafael Pires de Lima, Katie F. Welch, James E. Barrick, et al.
Palaios (2020) Vol. 35, Iss. 9, pp. 391-402
Closed Access | Times Cited: 29
Identification of tight sandstone reservoir lithofacies based on CNN image recognition technology: A case study of Fuyu reservoir of Sanzhao Sag in Songliao Basin
Fang Liu, Xin Wang, Zongbao Liu, et al.
Geoenergy Science and Engineering (2023) Vol. 222, pp. 211459-211459
Closed Access | Times Cited: 9
Fang Liu, Xin Wang, Zongbao Liu, et al.
Geoenergy Science and Engineering (2023) Vol. 222, pp. 211459-211459
Closed Access | Times Cited: 9
Impact of data pre-processing techniques on recurrent neural network performance in context of real-time drilling logs in an automated prediction framework
Andrzej T. Tunkiel, Dan Sui, Tomasz Wiktorski
Journal of Petroleum Science and Engineering (2021) Vol. 208, pp. 109760-109760
Open Access | Times Cited: 22
Andrzej T. Tunkiel, Dan Sui, Tomasz Wiktorski
Journal of Petroleum Science and Engineering (2021) Vol. 208, pp. 109760-109760
Open Access | Times Cited: 22
Machine learning for seismic exploration: Where are we and how far are we from the holy grail?
Farbod Khosro Anjom, Francesco Vaccarino, Laura Valentina Socco
Geophysics (2023) Vol. 89, Iss. 1, pp. WA157-WA178
Open Access | Times Cited: 8
Farbod Khosro Anjom, Francesco Vaccarino, Laura Valentina Socco
Geophysics (2023) Vol. 89, Iss. 1, pp. WA157-WA178
Open Access | Times Cited: 8
Predicting Magnetization Directions Using Convolutional Neural Networks
Felicia Nurindrawati, Jiajia Sun
Journal of Geophysical Research Solid Earth (2020) Vol. 125, Iss. 10
Closed Access | Times Cited: 23
Felicia Nurindrawati, Jiajia Sun
Journal of Geophysical Research Solid Earth (2020) Vol. 125, Iss. 10
Closed Access | Times Cited: 23
Multi-task learning for digital rock segmentation and characteristic parameters computation
Danping Cao, Siqi Ji, Rongang Cui, et al.
Journal of Petroleum Science and Engineering (2021) Vol. 208, pp. 109202-109202
Closed Access | Times Cited: 20
Danping Cao, Siqi Ji, Rongang Cui, et al.
Journal of Petroleum Science and Engineering (2021) Vol. 208, pp. 109202-109202
Closed Access | Times Cited: 20
RockDNet: Deep Learning Approach for Lithology Classification
Mohammed A. M. Abdullah, Ahmed Mohammed, Sohaib R. Awad
Applied Sciences (2024) Vol. 14, Iss. 13, pp. 5511-5511
Open Access | Times Cited: 2
Mohammed A. M. Abdullah, Ahmed Mohammed, Sohaib R. Awad
Applied Sciences (2024) Vol. 14, Iss. 13, pp. 5511-5511
Open Access | Times Cited: 2
Pretraining Convolutional Neural Networks for Mudstone Petrographic Thin-Section Image Classification
Rafael Pires de Lima, D. Duarte
Geosciences (2021) Vol. 11, Iss. 8, pp. 336-336
Open Access | Times Cited: 17
Rafael Pires de Lima, D. Duarte
Geosciences (2021) Vol. 11, Iss. 8, pp. 336-336
Open Access | Times Cited: 17
Hierarchical multi-label taxonomic classification of carbonate skeletal grains with deep learning
Madison Ho, Sidhant Idgunji, Jonathan L. Payne, et al.
Sedimentary Geology (2022) Vol. 443, pp. 106298-106298
Closed Access | Times Cited: 11
Madison Ho, Sidhant Idgunji, Jonathan L. Payne, et al.
Sedimentary Geology (2022) Vol. 443, pp. 106298-106298
Closed Access | Times Cited: 11
Assessment of the effectiveness of a convolutional autoencoder for digital image-based automated core logging
Jaesung Park, Jina Jeong
Geoenergy Science and Engineering (2023) Vol. 227, pp. 211802-211802
Open Access | Times Cited: 5
Jaesung Park, Jina Jeong
Geoenergy Science and Engineering (2023) Vol. 227, pp. 211802-211802
Open Access | Times Cited: 5
Lithology classification of whole core CT scans using convolutional neural networks
Kurdistan Chawshin, Carl Fredrik Berg, Damiano Varagnolo, et al.
SN Applied Sciences (2021) Vol. 3, Iss. 6
Open Access | Times Cited: 12
Kurdistan Chawshin, Carl Fredrik Berg, Damiano Varagnolo, et al.
SN Applied Sciences (2021) Vol. 3, Iss. 6
Open Access | Times Cited: 12
Applying convolutional neural networks to identify lithofacies of large-n cores from the Permian Basin and Gulf of Mexico: The importance of the quantity and quality of training data
Jinyu Zhang, William A. Ambrose, Wei Xie
Marine and Petroleum Geology (2021) Vol. 133, pp. 105307-105307
Closed Access | Times Cited: 12
Jinyu Zhang, William A. Ambrose, Wei Xie
Marine and Petroleum Geology (2021) Vol. 133, pp. 105307-105307
Closed Access | Times Cited: 12