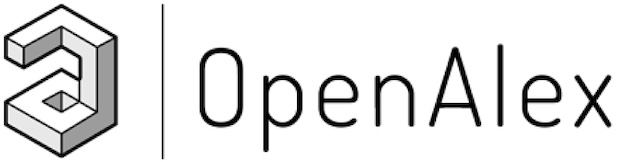
OpenAlex is a bibliographic catalogue of scientific papers, authors and institutions accessible in open access mode, named after the Library of Alexandria. It's citation coverage is excellent and I hope you will find utility in this listing of citing articles!
If you click the article title, you'll navigate to the article, as listed in CrossRef. If you click the Open Access links, you'll navigate to the "best Open Access location". Clicking the citation count will open this listing for that article. Lastly at the bottom of the page, you'll find basic pagination options.
Requested Article:
SkipCPP-Pred: an improved and promising sequence-based predictor for predicting cell-penetrating peptides
Leyi Wei, Jijun Tang, Quan Zou
BMC Genomics (2017) Vol. 18, Iss. S7
Open Access | Times Cited: 123
Leyi Wei, Jijun Tang, Quan Zou
BMC Genomics (2017) Vol. 18, Iss. S7
Open Access | Times Cited: 123
Showing 1-25 of 123 citing articles:
ACPred-FL: a sequence-based predictor using effective feature representation to improve the prediction of anti-cancer peptides
Leyi Wei, Chen Zhou, Huangrong Chen, et al.
Bioinformatics (2018) Vol. 34, Iss. 23, pp. 4007-4016
Open Access | Times Cited: 386
Leyi Wei, Chen Zhou, Huangrong Chen, et al.
Bioinformatics (2018) Vol. 34, Iss. 23, pp. 4007-4016
Open Access | Times Cited: 386
Machine intelligence in peptide therapeutics: A next‐generation tool for rapid disease screening
Shaherin Basith, Balachandran Manavalan, Tae Hwan Shin, et al.
Medicinal Research Reviews (2020) Vol. 40, Iss. 4, pp. 1276-1314
Closed Access | Times Cited: 253
Shaherin Basith, Balachandran Manavalan, Tae Hwan Shin, et al.
Medicinal Research Reviews (2020) Vol. 40, Iss. 4, pp. 1276-1314
Closed Access | Times Cited: 253
Improved prediction of protein–protein interactions using novel negative samples, features, and an ensemble classifier
Leyi Wei, Pengwei Xing, Jiancang Zeng, et al.
Artificial Intelligence in Medicine (2017) Vol. 83, pp. 67-74
Closed Access | Times Cited: 229
Leyi Wei, Pengwei Xing, Jiancang Zeng, et al.
Artificial Intelligence in Medicine (2017) Vol. 83, pp. 67-74
Closed Access | Times Cited: 229
PEPred-Suite: improved and robust prediction of therapeutic peptides using adaptive feature representation learning
Leyi Wei, Chen Zhou, Ran Su, et al.
Bioinformatics (2019) Vol. 35, Iss. 21, pp. 4272-4280
Closed Access | Times Cited: 161
Leyi Wei, Chen Zhou, Ran Su, et al.
Bioinformatics (2019) Vol. 35, Iss. 21, pp. 4272-4280
Closed Access | Times Cited: 161
MLCPP 2.0: An Updated Cell-penetrating Peptides and Their Uptake Efficiency Predictor
Balachandran Manavalan, Mahesh Chandra Patra
Journal of Molecular Biology (2022) Vol. 434, Iss. 11, pp. 167604-167604
Closed Access | Times Cited: 72
Balachandran Manavalan, Mahesh Chandra Patra
Journal of Molecular Biology (2022) Vol. 434, Iss. 11, pp. 167604-167604
Closed Access | Times Cited: 72
Empirical comparison and analysis of web-based cell-penetrating peptide prediction tools
Ran Su, Jie Hu, Quan Zou, et al.
Briefings in Bioinformatics (2018) Vol. 21, Iss. 2, pp. 408-420
Closed Access | Times Cited: 148
Ran Su, Jie Hu, Quan Zou, et al.
Briefings in Bioinformatics (2018) Vol. 21, Iss. 2, pp. 408-420
Closed Access | Times Cited: 148
ACPred-Fuse: fusing multi-view information improves the prediction of anticancer peptides
B. Dharma Rao, Chen Zhou, Guoying Zhang, et al.
Briefings in Bioinformatics (2019) Vol. 21, Iss. 5, pp. 1846-1855
Closed Access | Times Cited: 142
B. Dharma Rao, Chen Zhou, Guoying Zhang, et al.
Briefings in Bioinformatics (2019) Vol. 21, Iss. 5, pp. 1846-1855
Closed Access | Times Cited: 142
StackCPPred: a stacking and pairwise energy content-based prediction of cell-penetrating peptides and their uptake efficiency
Xiangzheng Fu, Lijun Cai, Xiangxiang Zeng, et al.
Bioinformatics (2020) Vol. 36, Iss. 10, pp. 3028-3034
Open Access | Times Cited: 124
Xiangzheng Fu, Lijun Cai, Xiangxiang Zeng, et al.
Bioinformatics (2020) Vol. 36, Iss. 10, pp. 3028-3034
Open Access | Times Cited: 124
CPPred-FL: a sequence-based predictor for large-scale identification of cell-penetrating peptides by feature representation learning
Xiaoli Qiang, Chen Zhou, Xiucai Ye, et al.
Briefings in Bioinformatics (2018)
Closed Access | Times Cited: 121
Xiaoli Qiang, Chen Zhou, Xiucai Ye, et al.
Briefings in Bioinformatics (2018)
Closed Access | Times Cited: 121
Comparative analysis and prediction of quorum-sensing peptides using feature representation learning and machine learning algorithms
Leyi Wei, Jie Hu, Fuyi Li, et al.
Briefings in Bioinformatics (2018)
Closed Access | Times Cited: 113
Leyi Wei, Jie Hu, Fuyi Li, et al.
Briefings in Bioinformatics (2018)
Closed Access | Times Cited: 113
iGHBP: Computational identification of growth hormone binding proteins from sequences using extremely randomised tree
Shaherin Basith, Balachandran Manavalan, Tae Hwan Shin, et al.
Computational and Structural Biotechnology Journal (2018) Vol. 16, pp. 412-420
Open Access | Times Cited: 111
Shaherin Basith, Balachandran Manavalan, Tae Hwan Shin, et al.
Computational and Structural Biotechnology Journal (2018) Vol. 16, pp. 412-420
Open Access | Times Cited: 111
Resistance against Sclerotinia sclerotiorum in soybean involves a reprogramming of the phenylpropanoid pathway and up‐regulation of antifungal activity targeting ergosterol biosynthesis
Ashish Ranjan, Nathaniel Westrick, Sachin Jain, et al.
Plant Biotechnology Journal (2019) Vol. 17, Iss. 8, pp. 1567-1581
Open Access | Times Cited: 102
Ashish Ranjan, Nathaniel Westrick, Sachin Jain, et al.
Plant Biotechnology Journal (2019) Vol. 17, Iss. 8, pp. 1567-1581
Open Access | Times Cited: 102
Predicting cell-penetrating peptides using machine learning algorithms and navigating in their chemical space
Ewerton Cristhian Lima de Oliveira, Kauê Santana da Costa, Luiz Patrick Cordeiro Josino, et al.
Scientific Reports (2021) Vol. 11, Iss. 1
Open Access | Times Cited: 87
Ewerton Cristhian Lima de Oliveira, Kauê Santana da Costa, Luiz Patrick Cordeiro Josino, et al.
Scientific Reports (2021) Vol. 11, Iss. 1
Open Access | Times Cited: 87
Incorporating Distance-Based Top-n-gram and Random Forest To Identify Electron Transport Proteins
Xiaoqing Ru, Lihong Li, Quan Zou
Journal of Proteome Research (2019) Vol. 18, Iss. 7, pp. 2931-2939
Closed Access | Times Cited: 79
Xiaoqing Ru, Lihong Li, Quan Zou
Journal of Proteome Research (2019) Vol. 18, Iss. 7, pp. 2931-2939
Closed Access | Times Cited: 79
k-Skip-n-Gram-RF: A Random Forest Based Method for Alzheimer's Disease Protein Identification
Lei Xu, Guangmin Liang, Changrui Liao, et al.
Frontiers in Genetics (2019) Vol. 10
Open Access | Times Cited: 76
Lei Xu, Guangmin Liang, Changrui Liao, et al.
Frontiers in Genetics (2019) Vol. 10
Open Access | Times Cited: 76
ATSE: a peptide toxicity predictor by exploiting structural and evolutionary information based on graph neural network and attention mechanism
Lesong Wei, Xiucai Ye, Yuyang Xue, et al.
Briefings in Bioinformatics (2021) Vol. 22, Iss. 5
Closed Access | Times Cited: 72
Lesong Wei, Xiucai Ye, Yuyang Xue, et al.
Briefings in Bioinformatics (2021) Vol. 22, Iss. 5
Closed Access | Times Cited: 72
Deep learning to design nuclear-targeting abiotic miniproteins
Carly K. Schissel, Somesh Mohapatra, Justin M. Wolfe, et al.
Nature Chemistry (2021) Vol. 13, Iss. 10, pp. 992-1000
Open Access | Times Cited: 65
Carly K. Schissel, Somesh Mohapatra, Justin M. Wolfe, et al.
Nature Chemistry (2021) Vol. 13, Iss. 10, pp. 992-1000
Open Access | Times Cited: 65
Machine learning-driven multifunctional peptide engineering for sustained ocular drug delivery
Henry T. Hsueh, Renee Ti Chou, Usha Rai, et al.
Nature Communications (2023) Vol. 14, Iss. 1
Open Access | Times Cited: 33
Henry T. Hsueh, Renee Ti Chou, Usha Rai, et al.
Nature Communications (2023) Vol. 14, Iss. 1
Open Access | Times Cited: 33
PractiCPP: a deep learning approach tailored for extremely imbalanced datasets in cell-penetrating peptide prediction
Kexin Shi, Yuanpeng Xiong, Yu Wang, et al.
Bioinformatics (2024) Vol. 40, Iss. 2
Open Access | Times Cited: 11
Kexin Shi, Yuanpeng Xiong, Yu Wang, et al.
Bioinformatics (2024) Vol. 40, Iss. 2
Open Access | Times Cited: 11
A hybrid residue based sequential encoding mechanism with XGBoost improved ensemble model for identifying 5-hydroxymethylcytosine modifications
Islam Uddin, Hamid Hussain Awan, Majdi Khalid, et al.
Scientific Reports (2024) Vol. 14, Iss. 1
Open Access | Times Cited: 11
Islam Uddin, Hamid Hussain Awan, Majdi Khalid, et al.
Scientific Reports (2024) Vol. 14, Iss. 1
Open Access | Times Cited: 11
Identifying N6-methyladenosine sites using multi-interval nucleotide pair position specificity and support vector machine
Pengwei Xing, Ran Su, Fei Guo, et al.
Scientific Reports (2017) Vol. 7, Iss. 1
Open Access | Times Cited: 86
Pengwei Xing, Ran Su, Fei Guo, et al.
Scientific Reports (2017) Vol. 7, Iss. 1
Open Access | Times Cited: 86
KELM-CPPpred: Kernel Extreme Learning Machine Based Prediction Model for Cell-Penetrating Peptides
Poonam Pandey, Vinal Patel, Nithin V. George, et al.
Journal of Proteome Research (2018) Vol. 17, Iss. 9, pp. 3214-3222
Closed Access | Times Cited: 75
Poonam Pandey, Vinal Patel, Nithin V. George, et al.
Journal of Proteome Research (2018) Vol. 17, Iss. 9, pp. 3214-3222
Closed Access | Times Cited: 75
TargetCPP: accurate prediction of cell-penetrating peptides from optimized multi-scale features using gradient boost decision tree
Muhammad Arif, Saeed Ahmad, Farman Ali, et al.
Journal of Computer-Aided Molecular Design (2020) Vol. 34, Iss. 8, pp. 841-856
Closed Access | Times Cited: 67
Muhammad Arif, Saeed Ahmad, Farman Ali, et al.
Journal of Computer-Aided Molecular Design (2020) Vol. 34, Iss. 8, pp. 841-856
Closed Access | Times Cited: 67
Cppsite 2.0: An Available Database of Experimentally Validated Cell-Penetrating Peptides Predicting their Secondary and Tertiary Structures
Kimia Kardani, Azam Bolhassani
Journal of Molecular Biology (2020) Vol. 433, Iss. 11, pp. 166703-166703
Open Access | Times Cited: 60
Kimia Kardani, Azam Bolhassani
Journal of Molecular Biology (2020) Vol. 433, Iss. 11, pp. 166703-166703
Open Access | Times Cited: 60
Prediction of Amphiphilic Cell-Penetrating Peptide Building Blocks from Protein-Derived Amino Acid Sequences for Engineering of Drug Delivery Nanoassemblies
Guillaume Feger, Borislav Angelov, Angelina Angelova
The Journal of Physical Chemistry B (2020) Vol. 124, Iss. 20, pp. 4069-4078
Open Access | Times Cited: 53
Guillaume Feger, Borislav Angelov, Angelina Angelova
The Journal of Physical Chemistry B (2020) Vol. 124, Iss. 20, pp. 4069-4078
Open Access | Times Cited: 53