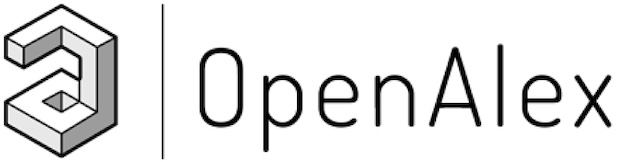
OpenAlex is a bibliographic catalogue of scientific papers, authors and institutions accessible in open access mode, named after the Library of Alexandria. It's citation coverage is excellent and I hope you will find utility in this listing of citing articles!
If you click the article title, you'll navigate to the article, as listed in CrossRef. If you click the Open Access links, you'll navigate to the "best Open Access location". Clicking the citation count will open this listing for that article. Lastly at the bottom of the page, you'll find basic pagination options.
Requested Article:
Grasp Pose Detection in Point Clouds
Andreas ten Pas, Marcus Gualtieri, Kate Saenko, et al.
The International Journal of Robotics Research (2017) Vol. 36, Iss. 13-14, pp. 1455-1473
Open Access | Times Cited: 465
Andreas ten Pas, Marcus Gualtieri, Kate Saenko, et al.
The International Journal of Robotics Research (2017) Vol. 36, Iss. 13-14, pp. 1455-1473
Open Access | Times Cited: 465
Showing 1-25 of 465 citing articles:
QT-Opt: Scalable Deep Reinforcement Learning for Vision-Based Robotic Manipulation
Dmitry Kalashnikov, Alex Irpan, Peter Pástor, et al.
arXiv (Cornell University) (2018)
Open Access | Times Cited: 578
Dmitry Kalashnikov, Alex Irpan, Peter Pástor, et al.
arXiv (Cornell University) (2018)
Open Access | Times Cited: 578
Learning ambidextrous robot grasping policies
Jeffrey Mahler, Matthew Matl, Vishal Satish, et al.
Science Robotics (2019) Vol. 4, Iss. 26
Closed Access | Times Cited: 487
Jeffrey Mahler, Matthew Matl, Vishal Satish, et al.
Science Robotics (2019) Vol. 4, Iss. 26
Closed Access | Times Cited: 487
6-DOF GraspNet: Variational Grasp Generation for Object Manipulation
Arsalan Mousavian, Clemens Eppner, Dieter Fox
2021 IEEE/CVF International Conference on Computer Vision (ICCV) (2019), pp. 2901-2910
Open Access | Times Cited: 443
Arsalan Mousavian, Clemens Eppner, Dieter Fox
2021 IEEE/CVF International Conference on Computer Vision (ICCV) (2019), pp. 2901-2910
Open Access | Times Cited: 443
How to train your robot with deep reinforcement learning: lessons we have learned
Julian Ibarz, Jie Tan, Chelsea Finn, et al.
The International Journal of Robotics Research (2021) Vol. 40, Iss. 4-5, pp. 698-721
Open Access | Times Cited: 380
Julian Ibarz, Jie Tan, Chelsea Finn, et al.
The International Journal of Robotics Research (2021) Vol. 40, Iss. 4-5, pp. 698-721
Open Access | Times Cited: 380
Sim-To-Real via Sim-To-Sim: Data-Efficient Robotic Grasping via Randomized-To-Canonical Adaptation Networks
Stephen James, Paul Wohlhart, Mrinal Kalakrishnan, et al.
2022 IEEE/CVF Conference on Computer Vision and Pattern Recognition (CVPR) (2019), pp. 12619-12629
Closed Access | Times Cited: 366
Stephen James, Paul Wohlhart, Mrinal Kalakrishnan, et al.
2022 IEEE/CVF Conference on Computer Vision and Pattern Recognition (CVPR) (2019), pp. 12619-12629
Closed Access | Times Cited: 366
Vision-based robotic grasping from object localization, object pose estimation to grasp estimation for parallel grippers: a review
Guoguang Du, Kai Wang, Shiguo Lian, et al.
Artificial Intelligence Review (2020) Vol. 54, Iss. 3, pp. 1677-1734
Open Access | Times Cited: 351
Guoguang Du, Kai Wang, Shiguo Lian, et al.
Artificial Intelligence Review (2020) Vol. 54, Iss. 3, pp. 1677-1734
Open Access | Times Cited: 351
Learning robust, real-time, reactive robotic grasping
Douglas Morrison, Peter Corke, Jürgen Leitner
The International Journal of Robotics Research (2019) Vol. 39, Iss. 2-3, pp. 183-201
Open Access | Times Cited: 335
Douglas Morrison, Peter Corke, Jürgen Leitner
The International Journal of Robotics Research (2019) Vol. 39, Iss. 2-3, pp. 183-201
Open Access | Times Cited: 335
GraspNet-1Billion: A Large-Scale Benchmark for General Object Grasping
Hao-Shu Fang, Chenxi Wang, Minghao Gou, et al.
2022 IEEE/CVF Conference on Computer Vision and Pattern Recognition (CVPR) (2020), pp. 11441-11450
Closed Access | Times Cited: 332
Hao-Shu Fang, Chenxi Wang, Minghao Gou, et al.
2022 IEEE/CVF Conference on Computer Vision and Pattern Recognition (CVPR) (2020), pp. 11441-11450
Closed Access | Times Cited: 332
PointNetGPD: Detecting Grasp Configurations from Point Sets
Hongzhuo Liang, Xiaojian Ma, Shuang Li, et al.
2022 International Conference on Robotics and Automation (ICRA) (2019)
Open Access | Times Cited: 290
Hongzhuo Liang, Xiaojian Ma, Shuang Li, et al.
2022 International Conference on Robotics and Automation (ICRA) (2019)
Open Access | Times Cited: 290
QT-Opt: Scalable Deep Reinforcement Learning for Vision-Based Robotic Manipulation
Dmitry Kalashnikov, Alex Irpan, Peter Pástor, et al.
Conference on Robot Learning (2018), pp. 651-673
Closed Access | Times Cited: 216
Dmitry Kalashnikov, Alex Irpan, Peter Pástor, et al.
Conference on Robot Learning (2018), pp. 651-673
Closed Access | Times Cited: 216
Deep Reinforcement Learning for Vision-Based Robotic Grasping: A Simulated Comparative Evaluation of Off-Policy Methods
Deirdre Quillen, Eric Jang, Ofir Nachum, et al.
(2018)
Open Access | Times Cited: 208
Deirdre Quillen, Eric Jang, Ofir Nachum, et al.
(2018)
Open Access | Times Cited: 208
A Survey on Learning-Based Robotic Grasping
Kilian Kleeberger, Richard Bormann, Werner Kraus, et al.
Current Robotics Reports (2020) Vol. 1, Iss. 4, pp. 239-249
Open Access | Times Cited: 208
Kilian Kleeberger, Richard Bormann, Werner Kraus, et al.
Current Robotics Reports (2020) Vol. 1, Iss. 4, pp. 239-249
Open Access | Times Cited: 208
Contact-GraspNet: Efficient 6-DoF Grasp Generation in Cluttered Scenes
Martin Sundermeyer, Arsalan Mousavian, Rudolph Triebel, et al.
(2021)
Open Access | Times Cited: 185
Martin Sundermeyer, Arsalan Mousavian, Rudolph Triebel, et al.
(2021)
Open Access | Times Cited: 185
6-DOF Grasping for Target-driven Object Manipulation in Clutter
Adithyavairavan Murali, Arsalan Mousavian, Clemens Eppner, et al.
(2020), pp. 6232-6238
Open Access | Times Cited: 173
Adithyavairavan Murali, Arsalan Mousavian, Clemens Eppner, et al.
(2020), pp. 6232-6238
Open Access | Times Cited: 173
Review of Deep Learning Methods in Robotic Grasp Detection
Shehan Caldera, Alexander Rassau, Douglas Chai
Multimodal Technologies and Interaction (2018) Vol. 2, Iss. 3, pp. 57-57
Open Access | Times Cited: 163
Shehan Caldera, Alexander Rassau, Douglas Chai
Multimodal Technologies and Interaction (2018) Vol. 2, Iss. 3, pp. 57-57
Open Access | Times Cited: 163
Learning task-oriented grasping for tool manipulation from simulated self-supervision
Kuan Fang, Yuke Zhu, Animesh Garg, et al.
The International Journal of Robotics Research (2019) Vol. 39, Iss. 2-3, pp. 202-216
Open Access | Times Cited: 156
Kuan Fang, Yuke Zhu, Animesh Garg, et al.
The International Journal of Robotics Research (2019) Vol. 39, Iss. 2-3, pp. 202-216
Open Access | Times Cited: 156
Real-Time Fruit Recognition and Grasping Estimation for Robotic Apple Harvesting
Hanwen Kang, Hongyu Zhou, Xing Wang, et al.
Sensors (2020) Vol. 20, Iss. 19, pp. 5670-5670
Open Access | Times Cited: 139
Hanwen Kang, Hongyu Zhou, Xing Wang, et al.
Sensors (2020) Vol. 20, Iss. 19, pp. 5670-5670
Open Access | Times Cited: 139
Deep Learning Approaches to Grasp Synthesis: A Review
R. Newbury, Morris Gu, Lachlan Chumbley, et al.
IEEE Transactions on Robotics (2023) Vol. 39, Iss. 5, pp. 3994-4015
Open Access | Times Cited: 91
R. Newbury, Morris Gu, Lachlan Chumbley, et al.
IEEE Transactions on Robotics (2023) Vol. 39, Iss. 5, pp. 3994-4015
Open Access | Times Cited: 91
AnyGrasp: Robust and Efficient Grasp Perception in Spatial and Temporal Domains
Hao-Shu Fang, Chenxi Wang, Hongjie Fang, et al.
IEEE Transactions on Robotics (2023) Vol. 39, Iss. 5, pp. 3929-3945
Open Access | Times Cited: 46
Hao-Shu Fang, Chenxi Wang, Hongjie Fang, et al.
IEEE Transactions on Robotics (2023) Vol. 39, Iss. 5, pp. 3929-3945
Open Access | Times Cited: 46
On-Policy Dataset Synthesis for Learning Robot Grasping Policies Using Fully Convolutional Deep Networks
Vishal Satish, Jeffrey Mahler, Ken Goldberg
IEEE Robotics and Automation Letters (2019) Vol. 4, Iss. 2, pp. 1357-1364
Closed Access | Times Cited: 122
Vishal Satish, Jeffrey Mahler, Ken Goldberg
IEEE Robotics and Automation Letters (2019) Vol. 4, Iss. 2, pp. 1357-1364
Closed Access | Times Cited: 122
PointNet++ Grasping: Learning An End-to-end Spatial Grasp Generation Algorithm from Sparse Point Clouds
Peiyuan Ni, Wenguang Zhang, Xiaoxiao Zhu, et al.
(2020), pp. 3619-3625
Open Access | Times Cited: 108
Peiyuan Ni, Wenguang Zhang, Xiaoxiao Zhu, et al.
(2020), pp. 3619-3625
Open Access | Times Cited: 108
ACRONYM: A Large-Scale Grasp Dataset Based on Simulation
Clemens Eppner, Arsalan Mousavian, Dieter Fox
(2021)
Open Access | Times Cited: 97
Clemens Eppner, Arsalan Mousavian, Dieter Fox
(2021)
Open Access | Times Cited: 97
EGAD! An Evolved Grasping Analysis Dataset for Diversity and Reproducibility in Robotic Manipulation
Douglas Morrison, Peter Corke, Jürgen Leitner
IEEE Robotics and Automation Letters (2020) Vol. 5, Iss. 3, pp. 4368-4375
Open Access | Times Cited: 89
Douglas Morrison, Peter Corke, Jürgen Leitner
IEEE Robotics and Automation Letters (2020) Vol. 5, Iss. 3, pp. 4368-4375
Open Access | Times Cited: 89
RGB Matters: Learning 7-DoF Grasp Poses on Monocular RGBD Images
Minghao Gou, Hao-Shu Fang, Zhanda Zhu, et al.
(2021), pp. 13459-13466
Open Access | Times Cited: 83
Minghao Gou, Hao-Shu Fang, Zhanda Zhu, et al.
(2021), pp. 13459-13466
Open Access | Times Cited: 83
Review of Deep Reinforcement Learning-Based Object Grasping: Techniques, Open Challenges, and Recommendations
Marwan Qaid Mohammed, Lee Chung Kwek, Shing Chyi Chua
IEEE Access (2020) Vol. 8, pp. 178450-178481
Open Access | Times Cited: 81
Marwan Qaid Mohammed, Lee Chung Kwek, Shing Chyi Chua
IEEE Access (2020) Vol. 8, pp. 178450-178481
Open Access | Times Cited: 81