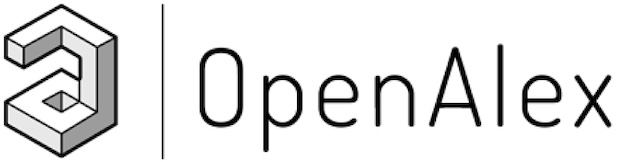
OpenAlex is a bibliographic catalogue of scientific papers, authors and institutions accessible in open access mode, named after the Library of Alexandria. It's citation coverage is excellent and I hope you will find utility in this listing of citing articles!
If you click the article title, you'll navigate to the article, as listed in CrossRef. If you click the Open Access links, you'll navigate to the "best Open Access location". Clicking the citation count will open this listing for that article. Lastly at the bottom of the page, you'll find basic pagination options.
Requested Article:
ChemOS: Orchestrating autonomous experimentation
Loı̈c M. Roch, Florian Häse, Christoph Kreisbeck, et al.
Science Robotics (2018) Vol. 3, Iss. 19
Closed Access | Times Cited: 146
Loı̈c M. Roch, Florian Häse, Christoph Kreisbeck, et al.
Science Robotics (2018) Vol. 3, Iss. 19
Closed Access | Times Cited: 146
Showing 1-25 of 146 citing articles:
Inverse molecular design using machine learning: Generative models for matter engineering
Benjamín Sánchez-Lengeling, Alán Aspuru‐Guzik
Science (2018) Vol. 361, Iss. 6400, pp. 360-365
Open Access | Times Cited: 1585
Benjamín Sánchez-Lengeling, Alán Aspuru‐Guzik
Science (2018) Vol. 361, Iss. 6400, pp. 360-365
Open Access | Times Cited: 1585
A mobile robotic chemist
Benjamin Burger, Phillip M. Maffettone, Vladimir V. Gusev, et al.
Nature (2020) Vol. 583, Iss. 7815, pp. 237-241
Closed Access | Times Cited: 1021
Benjamin Burger, Phillip M. Maffettone, Vladimir V. Gusev, et al.
Nature (2020) Vol. 583, Iss. 7815, pp. 237-241
Closed Access | Times Cited: 1021
QSAR without borders
Eugene Muratov, Jürgen Bajorath, Robert P. Sheridan, et al.
Chemical Society Reviews (2020) Vol. 49, Iss. 11, pp. 3525-3564
Open Access | Times Cited: 617
Eugene Muratov, Jürgen Bajorath, Robert P. Sheridan, et al.
Chemical Society Reviews (2020) Vol. 49, Iss. 11, pp. 3525-3564
Open Access | Times Cited: 617
Deep learning for molecular design—a review of the state of the art
Daniel C. Elton, Zois Boukouvalas, Mark Fuge, et al.
Molecular Systems Design & Engineering (2019) Vol. 4, Iss. 4, pp. 828-849
Open Access | Times Cited: 596
Daniel C. Elton, Zois Boukouvalas, Mark Fuge, et al.
Molecular Systems Design & Engineering (2019) Vol. 4, Iss. 4, pp. 828-849
Open Access | Times Cited: 596
Recent advances and applications of deep learning methods in materials science
Kamal Choudhary, Brian DeCost, Chi Chen, et al.
npj Computational Materials (2022) Vol. 8, Iss. 1
Open Access | Times Cited: 592
Kamal Choudhary, Brian DeCost, Chi Chen, et al.
npj Computational Materials (2022) Vol. 8, Iss. 1
Open Access | Times Cited: 592
Self-driving laboratory for accelerated discovery of thin-film materials
Benjamin P. MacLeod, Fraser G. L. Parlane, Thomas D. Morrissey, et al.
Science Advances (2020) Vol. 6, Iss. 20
Open Access | Times Cited: 511
Benjamin P. MacLeod, Fraser G. L. Parlane, Thomas D. Morrissey, et al.
Science Advances (2020) Vol. 6, Iss. 20
Open Access | Times Cited: 511
Data-Driven Strategies for Accelerated Materials Design
Robert Pollice, Gabriel dos Passos Gomes, Matteo Aldeghi, et al.
Accounts of Chemical Research (2021) Vol. 54, Iss. 4, pp. 849-860
Open Access | Times Cited: 323
Robert Pollice, Gabriel dos Passos Gomes, Matteo Aldeghi, et al.
Accounts of Chemical Research (2021) Vol. 54, Iss. 4, pp. 849-860
Open Access | Times Cited: 323
Machine Learning for Chemical Reactions
Markus Meuwly
Chemical Reviews (2021) Vol. 121, Iss. 16, pp. 10218-10239
Closed Access | Times Cited: 310
Markus Meuwly
Chemical Reviews (2021) Vol. 121, Iss. 16, pp. 10218-10239
Closed Access | Times Cited: 310
The Role of Machine Learning in the Understanding and Design of Materials
Seyed Mohamad Moosavi, Kevin Maik Jablonka, Berend Smit
Journal of the American Chemical Society (2020) Vol. 142, Iss. 48, pp. 20273-20287
Open Access | Times Cited: 303
Seyed Mohamad Moosavi, Kevin Maik Jablonka, Berend Smit
Journal of the American Chemical Society (2020) Vol. 142, Iss. 48, pp. 20273-20287
Open Access | Times Cited: 303
Next-Generation Experimentation with Self-Driving Laboratories
Florian Häse, Loı̈c M. Roch, Alán Aspuru‐Guzik
Trends in Chemistry (2019) Vol. 1, Iss. 3, pp. 282-291
Closed Access | Times Cited: 286
Florian Häse, Loı̈c M. Roch, Alán Aspuru‐Guzik
Trends in Chemistry (2019) Vol. 1, Iss. 3, pp. 282-291
Closed Access | Times Cited: 286
Synthetic organic chemistry driven by artificial intelligence
A. Filipa Almeida, Rui Moreira, Tiago Rodrigues
Nature Reviews Chemistry (2019) Vol. 3, Iss. 10, pp. 589-604
Open Access | Times Cited: 271
A. Filipa Almeida, Rui Moreira, Tiago Rodrigues
Nature Reviews Chemistry (2019) Vol. 3, Iss. 10, pp. 589-604
Open Access | Times Cited: 271
On-the-fly closed-loop materials discovery via Bayesian active learning
A. Gilad Kusne, Heshan Yu, Changming Wu, et al.
Nature Communications (2020) Vol. 11, Iss. 1
Open Access | Times Cited: 271
A. Gilad Kusne, Heshan Yu, Changming Wu, et al.
Nature Communications (2020) Vol. 11, Iss. 1
Open Access | Times Cited: 271
Machine learning for renewable energy materials
Geun Ho Gu, Juhwan Noh, I. P. Kim, et al.
Journal of Materials Chemistry A (2019) Vol. 7, Iss. 29, pp. 17096-17117
Closed Access | Times Cited: 265
Geun Ho Gu, Juhwan Noh, I. P. Kim, et al.
Journal of Materials Chemistry A (2019) Vol. 7, Iss. 29, pp. 17096-17117
Closed Access | Times Cited: 265
Rechargeable Batteries of the Future—The State of the Art from a BATTERY 2030+ Perspective
Maximilian Fichtner, Kristina Edström, Elixabete Ayerbe, et al.
Advanced Energy Materials (2021) Vol. 12, Iss. 17
Open Access | Times Cited: 243
Maximilian Fichtner, Kristina Edström, Elixabete Ayerbe, et al.
Advanced Energy Materials (2021) Vol. 12, Iss. 17
Open Access | Times Cited: 243
Autonomous Discovery in the Chemical Sciences Part II: Outlook
Connor W. Coley, Natalie S. Eyke, Klavs F. Jensen
Angewandte Chemie International Edition (2019) Vol. 59, Iss. 52, pp. 23414-23436
Open Access | Times Cited: 237
Connor W. Coley, Natalie S. Eyke, Klavs F. Jensen
Angewandte Chemie International Edition (2019) Vol. 59, Iss. 52, pp. 23414-23436
Open Access | Times Cited: 237
Beyond Ternary OPV: High‐Throughput Experimentation and Self‐Driving Laboratories Optimize Multicomponent Systems
Stefan Langner, Florian Häse, José Darío Perea, et al.
Advanced Materials (2020) Vol. 32, Iss. 14
Open Access | Times Cited: 230
Stefan Langner, Florian Häse, José Darío Perea, et al.
Advanced Materials (2020) Vol. 32, Iss. 14
Open Access | Times Cited: 230
How to explore chemical space using algorithms and automation
Piotr S. Gromski, Alon Henson, Jarosław M. Granda, et al.
Nature Reviews Chemistry (2019) Vol. 3, Iss. 2, pp. 119-128
Closed Access | Times Cited: 221
Piotr S. Gromski, Alon Henson, Jarosław M. Granda, et al.
Nature Reviews Chemistry (2019) Vol. 3, Iss. 2, pp. 119-128
Closed Access | Times Cited: 221
Autonomous Discovery in the Chemical Sciences Part I: Progress
Connor W. Coley, Natalie S. Eyke, Klavs F. Jensen
Angewandte Chemie International Edition (2019) Vol. 59, Iss. 51, pp. 22858-22893
Open Access | Times Cited: 175
Connor W. Coley, Natalie S. Eyke, Klavs F. Jensen
Angewandte Chemie International Edition (2019) Vol. 59, Iss. 51, pp. 22858-22893
Open Access | Times Cited: 175
Bayesian Optimization for Calibrating and Selecting Hybrid-Density Functional Models
Rodrigo A. Vargas–Hernández
The Journal of Physical Chemistry A (2020) Vol. 124, Iss. 20, pp. 4053-4061
Open Access | Times Cited: 163
Rodrigo A. Vargas–Hernández
The Journal of Physical Chemistry A (2020) Vol. 124, Iss. 20, pp. 4053-4061
Open Access | Times Cited: 163
Materials science in the artificial intelligence age: high-throughput library generation, machine learning, and a pathway from correlations to the underpinning physics
Rama K. Vasudevan, Kamal Choudhary, Apurva Mehta, et al.
MRS Communications (2019) Vol. 9, Iss. 3, pp. 821-838
Open Access | Times Cited: 156
Rama K. Vasudevan, Kamal Choudhary, Apurva Mehta, et al.
MRS Communications (2019) Vol. 9, Iss. 3, pp. 821-838
Open Access | Times Cited: 156
Machine-enabled inverse design of inorganic solid materials: promises and challenges
Juhwan Noh, Geun Ho Gu, Sungwon Kim, et al.
Chemical Science (2020) Vol. 11, Iss. 19, pp. 4871-4881
Open Access | Times Cited: 147
Juhwan Noh, Geun Ho Gu, Sungwon Kim, et al.
Chemical Science (2020) Vol. 11, Iss. 19, pp. 4871-4881
Open Access | Times Cited: 147
Autonomous Chemical Experiments: Challenges and Perspectives on Establishing a Self-Driving Lab
Martin Seifrid, Robert Pollice, Andrés Aguilar-Gránda, et al.
Accounts of Chemical Research (2022) Vol. 55, Iss. 17, pp. 2454-2466
Open Access | Times Cited: 147
Martin Seifrid, Robert Pollice, Andrés Aguilar-Gránda, et al.
Accounts of Chemical Research (2022) Vol. 55, Iss. 17, pp. 2454-2466
Open Access | Times Cited: 147
A Roadmap for Transforming Research to Invent the Batteries of the Future Designed within the European Large Scale Research Initiative BATTERY 2030+
Julia Amici, Pietro Asinari, Elixabete Ayerbe, et al.
Advanced Energy Materials (2022) Vol. 12, Iss. 17
Open Access | Times Cited: 139
Julia Amici, Pietro Asinari, Elixabete Ayerbe, et al.
Advanced Energy Materials (2022) Vol. 12, Iss. 17
Open Access | Times Cited: 139
Toward autonomous design and synthesis of novel inorganic materials
Nathan J. Szymanski, Yan Zeng, Haoyan Huo, et al.
Materials Horizons (2021) Vol. 8, Iss. 8, pp. 2169-2198
Open Access | Times Cited: 107
Nathan J. Szymanski, Yan Zeng, Haoyan Huo, et al.
Materials Horizons (2021) Vol. 8, Iss. 8, pp. 2169-2198
Open Access | Times Cited: 107
Gryffin : An algorithm for Bayesian optimization of categorical variables informed by expert knowledge
Florian Häse, Matteo Aldeghi, Riley J. Hickman, et al.
Applied Physics Reviews (2021) Vol. 8, Iss. 3
Open Access | Times Cited: 104
Florian Häse, Matteo Aldeghi, Riley J. Hickman, et al.
Applied Physics Reviews (2021) Vol. 8, Iss. 3
Open Access | Times Cited: 104