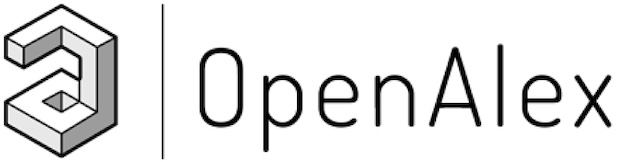
OpenAlex is a bibliographic catalogue of scientific papers, authors and institutions accessible in open access mode, named after the Library of Alexandria. It's citation coverage is excellent and I hope you will find utility in this listing of citing articles!
If you click the article title, you'll navigate to the article, as listed in CrossRef. If you click the Open Access links, you'll navigate to the "best Open Access location". Clicking the citation count will open this listing for that article. Lastly at the bottom of the page, you'll find basic pagination options.
Requested Article:
Biomolecular dynamics with machine-learned quantum-mechanical force fields trained on diverse chemical fragments
Oliver T. Unke, Martin Stöhr, Stefan Ganscha, et al.
Science Advances (2024) Vol. 10, Iss. 14
Open Access | Times Cited: 19
Oliver T. Unke, Martin Stöhr, Stefan Ganscha, et al.
Science Advances (2024) Vol. 10, Iss. 14
Open Access | Times Cited: 19
Showing 19 citing articles:
Prospective de novo drug design with deep interactome learning
Kenneth Atz, Leandro Cotos, Clemens Isert, et al.
Nature Communications (2024) Vol. 15, Iss. 1
Open Access | Times Cited: 22
Kenneth Atz, Leandro Cotos, Clemens Isert, et al.
Nature Communications (2024) Vol. 15, Iss. 1
Open Access | Times Cited: 22
Towards symbolic XAI – explanation through human understandable logical relationships between features
Thomas Schnake, Farnoush Rezaei Jafari, Jonas Lederer, et al.
Information Fusion (2025), pp. 102923-102923
Open Access | Times Cited: 1
Thomas Schnake, Farnoush Rezaei Jafari, Jonas Lederer, et al.
Information Fusion (2025), pp. 102923-102923
Open Access | Times Cited: 1
Differentiable simulation to develop molecular dynamics force fields for disordered proteins
Joe G. Greener
Chemical Science (2024) Vol. 15, Iss. 13, pp. 4897-4909
Open Access | Times Cited: 9
Joe G. Greener
Chemical Science (2024) Vol. 15, Iss. 13, pp. 4897-4909
Open Access | Times Cited: 9
A Euclidean transformer for fast and stable machine learned force fields
J. Thorben Frank, Oliver T. Unke, Klaus‐Robert Müller, et al.
Nature Communications (2024) Vol. 15, Iss. 1
Open Access | Times Cited: 9
J. Thorben Frank, Oliver T. Unke, Klaus‐Robert Müller, et al.
Nature Communications (2024) Vol. 15, Iss. 1
Open Access | Times Cited: 9
Fine-tuning molecular mechanics force fields to experimental free energy measurements
Dominic A. Rufa, Josh Fass, John D. Chodera
bioRxiv (Cold Spring Harbor Laboratory) (2025)
Open Access
Dominic A. Rufa, Josh Fass, John D. Chodera
bioRxiv (Cold Spring Harbor Laboratory) (2025)
Open Access
Grappa – a machine learned molecular mechanics force field
Leif Seute, Eric Hartmann, Jan Stühmer, et al.
Chemical Science (2025)
Open Access
Leif Seute, Eric Hartmann, Jan Stühmer, et al.
Chemical Science (2025)
Open Access
Self-assembly of architected macromolecules: Bridging a gap between experiments and simulations
Ji Woong Yu, Changsu Yoo, Suchan Cho, et al.
Chemical Physics Reviews (2025) Vol. 6, Iss. 1
Open Access
Ji Woong Yu, Changsu Yoo, Suchan Cho, et al.
Chemical Physics Reviews (2025) Vol. 6, Iss. 1
Open Access
Crash testing machine learning force fields for molecules, materials, and interfaces: molecular dynamics in the TEA challenge 2023
Igor Poltavsky, Mirela Puleva, Anton Charkin-Gorbulin, et al.
Chemical Science (2025)
Open Access
Igor Poltavsky, Mirela Puleva, Anton Charkin-Gorbulin, et al.
Chemical Science (2025)
Open Access
Modeling Boltzmann-weighted structural ensembles of proteins using artificial intelligence–based methods
Akashnathan Aranganathan, Xinyu Gu, Dedi Wang, et al.
Current Opinion in Structural Biology (2025) Vol. 91, pp. 103000-103000
Open Access
Akashnathan Aranganathan, Xinyu Gu, Dedi Wang, et al.
Current Opinion in Structural Biology (2025) Vol. 91, pp. 103000-103000
Open Access
Machine learning in molecular biophysics: Protein allostery, multi-level free energy simulations, and lipid phase transitions
Qiang Cui
Biophysics Reviews (2025) Vol. 6, Iss. 1
Closed Access
Qiang Cui
Biophysics Reviews (2025) Vol. 6, Iss. 1
Closed Access
ArcaNN: automated enhanced sampling generation of training sets for chemically reactive machine learning interatomic potentials
Rolf David, Miguel de la Puente, Axel Gomez, et al.
Digital Discovery (2024)
Open Access | Times Cited: 5
Rolf David, Miguel de la Puente, Axel Gomez, et al.
Digital Discovery (2024)
Open Access | Times Cited: 5
Ab initio characterization of protein molecular dynamics with AI2BMD
Tong Wang, Xinheng He, Mingyu Li, et al.
Nature (2024) Vol. 635, Iss. 8040, pp. 1019-1027
Open Access | Times Cited: 5
Tong Wang, Xinheng He, Mingyu Li, et al.
Nature (2024) Vol. 635, Iss. 8040, pp. 1019-1027
Open Access | Times Cited: 5
Recent Advances in Machine Learning‐Assisted Multiscale Design of Energy Materials
Bohayra Mortazavi
Advanced Energy Materials (2024)
Open Access | Times Cited: 3
Bohayra Mortazavi
Advanced Energy Materials (2024)
Open Access | Times Cited: 3
Data Generation for Machine Learning Interatomic Potentials and Beyond
Maksim Kulichenko, Benjamin Nebgen, Nicholas Lubbers, et al.
Chemical Reviews (2024) Vol. 124, Iss. 24, pp. 13681-13714
Closed Access | Times Cited: 2
Maksim Kulichenko, Benjamin Nebgen, Nicholas Lubbers, et al.
Chemical Reviews (2024) Vol. 124, Iss. 24, pp. 13681-13714
Closed Access | Times Cited: 2
Molecular relaxation by reverse diffusion with time step prediction
Khaled Kahouli, Stefaan S. P. Hessmann, Klaus‐Robert Müller, et al.
Machine Learning Science and Technology (2024) Vol. 5, Iss. 3, pp. 035038-035038
Open Access | Times Cited: 1
Khaled Kahouli, Stefaan S. P. Hessmann, Klaus‐Robert Müller, et al.
Machine Learning Science and Technology (2024) Vol. 5, Iss. 3, pp. 035038-035038
Open Access | Times Cited: 1
COSMOPharm: Drug–Polymer Compatibility of Pharmaceutical Amorphous Solid Dispersions from COSMO-SAC
Ivan Antolović, Jadran Vrabec, Martin Klajmon
Molecular Pharmaceutics (2024) Vol. 21, Iss. 9, pp. 4395-4415
Open Access | Times Cited: 1
Ivan Antolović, Jadran Vrabec, Martin Klajmon
Molecular Pharmaceutics (2024) Vol. 21, Iss. 9, pp. 4395-4415
Open Access | Times Cited: 1
Ensemble machine learning to accelerate industrial decarbonization: Prediction of Hansen solubility parameters for streamlined chemical solvent selection
Eslam G. Al-Sakkari, Ahmed Ragab, Mostafa Amer, et al.
Digital Chemical Engineering (2024), pp. 100207-100207
Open Access | Times Cited: 1
Eslam G. Al-Sakkari, Ahmed Ragab, Mostafa Amer, et al.
Digital Chemical Engineering (2024), pp. 100207-100207
Open Access | Times Cited: 1
Development of Machine Learning Models withFragment Molecular Orbital Calculation Data
Koichiro Kato, Hiromu Matsumoto, Ryosuke KITA
Journal of Computer Chemistry Japan (2024) Vol. 23, Iss. 4, pp. 98-104
Open Access
Koichiro Kato, Hiromu Matsumoto, Ryosuke KITA
Journal of Computer Chemistry Japan (2024) Vol. 23, Iss. 4, pp. 98-104
Open Access
On machine learnability of local contributions to interatomic potentials from density functional theory calculations
Mahboobeh Babaei, Ali Sadeghi
Scientific Reports (2024) Vol. 14, Iss. 1
Open Access
Mahboobeh Babaei, Ali Sadeghi
Scientific Reports (2024) Vol. 14, Iss. 1
Open Access
Δ-Machine Learning to Elevate DFT-Based Potentials and a Force Field to the CCSD(T) Level Illustrated for Ethanol
Apurba Nandi, Priyanka Pandey, Paul L. Houston, et al.
Journal of Chemical Theory and Computation (2024)
Open Access
Apurba Nandi, Priyanka Pandey, Paul L. Houston, et al.
Journal of Chemical Theory and Computation (2024)
Open Access