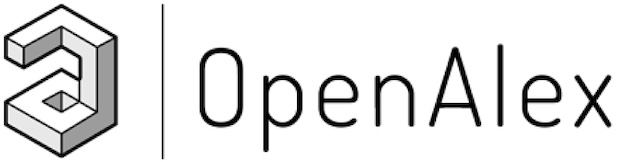
OpenAlex is a bibliographic catalogue of scientific papers, authors and institutions accessible in open access mode, named after the Library of Alexandria. It's citation coverage is excellent and I hope you will find utility in this listing of citing articles!
If you click the article title, you'll navigate to the article, as listed in CrossRef. If you click the Open Access links, you'll navigate to the "best Open Access location". Clicking the citation count will open this listing for that article. Lastly at the bottom of the page, you'll find basic pagination options.
Requested Article:
Learning the solution operator of parametric partial differential equations with physics-informed DeepONets
Sifan Wang, Hanwen Wang, Paris Perdikaris
Science Advances (2021) Vol. 7, Iss. 40
Open Access | Times Cited: 354
Sifan Wang, Hanwen Wang, Paris Perdikaris
Science Advances (2021) Vol. 7, Iss. 40
Open Access | Times Cited: 354
Showing 1-25 of 354 citing articles:
Finite basis physics-informed neural networks (FBPINNs): a scalable domain decomposition approach for solving differential equations
Ben Moseley, Andrew Markham, Tarje Nissen‐Meyer
Advances in Computational Mathematics (2023) Vol. 49, Iss. 4
Open Access | Times Cited: 132
Ben Moseley, Andrew Markham, Tarje Nissen‐Meyer
Advances in Computational Mathematics (2023) Vol. 49, Iss. 4
Open Access | Times Cited: 132
Transfer learning based physics-informed neural networks for solving inverse problems in engineering structures under different loading scenarios
Xu Chen, Ba Trung Cao, Yong Yuan, et al.
Computer Methods in Applied Mechanics and Engineering (2022) Vol. 405, pp. 115852-115852
Open Access | Times Cited: 113
Xu Chen, Ba Trung Cao, Yong Yuan, et al.
Computer Methods in Applied Mechanics and Engineering (2022) Vol. 405, pp. 115852-115852
Open Access | Times Cited: 113
Multifidelity deep neural operators for efficient learning of partial differential equations with application to fast inverse design of nanoscale heat transport
Lu Lu, Raphaël Pestourie, Steven G. Johnson, et al.
Physical Review Research (2022) Vol. 4, Iss. 2
Open Access | Times Cited: 91
Lu Lu, Raphaël Pestourie, Steven G. Johnson, et al.
Physical Review Research (2022) Vol. 4, Iss. 2
Open Access | Times Cited: 91
Learning two-phase microstructure evolution using neural operators and autoencoder architectures
Vivek Oommen, Khemraj Shukla, Somdatta Goswami, et al.
npj Computational Materials (2022) Vol. 8, Iss. 1
Open Access | Times Cited: 88
Vivek Oommen, Khemraj Shukla, Somdatta Goswami, et al.
npj Computational Materials (2022) Vol. 8, Iss. 1
Open Access | Times Cited: 88
MIONet: Learning Multiple-Input Operators via Tensor Product
Pengzhan Jin, Shuai Meng, Lu Lu
SIAM Journal on Scientific Computing (2022) Vol. 44, Iss. 6, pp. A3490-A3514
Open Access | Times Cited: 84
Pengzhan Jin, Shuai Meng, Lu Lu
SIAM Journal on Scientific Computing (2022) Vol. 44, Iss. 6, pp. A3490-A3514
Open Access | Times Cited: 84
Interfacing finite elements with deep neural operators for fast multiscale modeling of mechanics problems
Minglang Yin, Enrui Zhang, Yue Yu, et al.
Computer Methods in Applied Mechanics and Engineering (2022) Vol. 402, pp. 115027-115027
Open Access | Times Cited: 71
Minglang Yin, Enrui Zhang, Yue Yu, et al.
Computer Methods in Applied Mechanics and Engineering (2022) Vol. 402, pp. 115027-115027
Open Access | Times Cited: 71
Modeling finite-strain plasticity using physics-informed neural network and assessment of the network performance
Sijun Niu, Enrui Zhang, Yuri Bazilevs, et al.
Journal of the Mechanics and Physics of Solids (2022) Vol. 172, pp. 105177-105177
Open Access | Times Cited: 70
Sijun Niu, Enrui Zhang, Yuri Bazilevs, et al.
Journal of the Mechanics and Physics of Solids (2022) Vol. 172, pp. 105177-105177
Open Access | Times Cited: 70
Recent Advances and Applications of Machine Learning in Experimental Solid Mechanics: A Review
Hanxun Jin, Enrui Zhang, Horacio D. Espinosa
Applied Mechanics Reviews (2023) Vol. 75, Iss. 6
Open Access | Times Cited: 66
Hanxun Jin, Enrui Zhang, Horacio D. Espinosa
Applied Mechanics Reviews (2023) Vol. 75, Iss. 6
Open Access | Times Cited: 66
Reliable extrapolation of deep neural operators informed by physics or sparse observations
Min Zhu, Handi Zhang, Anran Jiao, et al.
Computer Methods in Applied Mechanics and Engineering (2023) Vol. 412, pp. 116064-116064
Open Access | Times Cited: 57
Min Zhu, Handi Zhang, Anran Jiao, et al.
Computer Methods in Applied Mechanics and Engineering (2023) Vol. 412, pp. 116064-116064
Open Access | Times Cited: 57
Encoding physics to learn reaction–diffusion processes
Chengping Rao, Pu Ren, Qi Wang, et al.
Nature Machine Intelligence (2023) Vol. 5, Iss. 7, pp. 765-779
Closed Access | Times Cited: 52
Chengping Rao, Pu Ren, Qi Wang, et al.
Nature Machine Intelligence (2023) Vol. 5, Iss. 7, pp. 765-779
Closed Access | Times Cited: 52
Explainable, interpretable, and trustworthy AI for an intelligent digital twin: A case study on remaining useful life
Kazuma Kobayashi, Syed Bahauddin Alam
Engineering Applications of Artificial Intelligence (2023) Vol. 129, pp. 107620-107620
Open Access | Times Cited: 52
Kazuma Kobayashi, Syed Bahauddin Alam
Engineering Applications of Artificial Intelligence (2023) Vol. 129, pp. 107620-107620
Open Access | Times Cited: 52
Physics-Informed Deep Neural Operator Networks
Somdatta Goswami, Aniruddha Bora, Yue Yu, et al.
Computational methods in engineering & the sciences (2023), pp. 219-254
Closed Access | Times Cited: 50
Somdatta Goswami, Aniruddha Bora, Yue Yu, et al.
Computational methods in engineering & the sciences (2023), pp. 219-254
Closed Access | Times Cited: 50
A deep learning energy-based method for classical elastoplasticity
Junyan He, Diab W. Abueidda, Rashid K. Abu Al‐Rub, et al.
International Journal of Plasticity (2023) Vol. 162, pp. 103531-103531
Open Access | Times Cited: 48
Junyan He, Diab W. Abueidda, Rashid K. Abu Al‐Rub, et al.
International Journal of Plasticity (2023) Vol. 162, pp. 103531-103531
Open Access | Times Cited: 48
Accelerating the design of compositionally complex materials via physics-informed artificial intelligence
Dierk Raabe, Jaber Rezaei Mianroodi, Jörg Neugebauer
Nature Computational Science (2023) Vol. 3, Iss. 3, pp. 198-209
Closed Access | Times Cited: 48
Dierk Raabe, Jaber Rezaei Mianroodi, Jörg Neugebauer
Nature Computational Science (2023) Vol. 3, Iss. 3, pp. 198-209
Closed Access | Times Cited: 48
Physics-Guided, Physics-Informed, and Physics-Encoded Neural Networks and Operators in Scientific Computing: Fluid and Solid Mechanics
Salah A. Faroughi, Nikhil M. Pawar, Célio Fernandes, et al.
Journal of Computing and Information Science in Engineering (2024) Vol. 24, Iss. 4
Closed Access | Times Cited: 45
Salah A. Faroughi, Nikhil M. Pawar, Célio Fernandes, et al.
Journal of Computing and Information Science in Engineering (2024) Vol. 24, Iss. 4
Closed Access | Times Cited: 45
Machine learning for numerical weather and climate modelling: a review
Catherine de Burgh-Day, Tennessee Leeuwenburg
Geoscientific model development (2023) Vol. 16, Iss. 22, pp. 6433-6477
Open Access | Times Cited: 42
Catherine de Burgh-Day, Tennessee Leeuwenburg
Geoscientific model development (2023) Vol. 16, Iss. 22, pp. 6433-6477
Open Access | Times Cited: 42
Sequential Deep Operator Networks (S-DeepONet) for predicting full-field solutions under time-dependent loads
Junyan He, Shashank Kushwaha, Jaewan Park, et al.
Engineering Applications of Artificial Intelligence (2023) Vol. 127, pp. 107258-107258
Open Access | Times Cited: 41
Junyan He, Shashank Kushwaha, Jaewan Park, et al.
Engineering Applications of Artificial Intelligence (2023) Vol. 127, pp. 107258-107258
Open Access | Times Cited: 41
Neural operators for accelerating scientific simulations and design
Kamyar Azizzadenesheli, Nikola B. Kovachki, Zongyi Li, et al.
Nature Reviews Physics (2024) Vol. 6, Iss. 5, pp. 320-328
Closed Access | Times Cited: 38
Kamyar Azizzadenesheli, Nikola B. Kovachki, Zongyi Li, et al.
Nature Reviews Physics (2024) Vol. 6, Iss. 5, pp. 320-328
Closed Access | Times Cited: 38
Deep learning in computational mechanics: a review
Leon Herrmann, Stefan Kollmannsberger
Computational Mechanics (2024) Vol. 74, Iss. 2, pp. 281-331
Open Access | Times Cited: 28
Leon Herrmann, Stefan Kollmannsberger
Computational Mechanics (2024) Vol. 74, Iss. 2, pp. 281-331
Open Access | Times Cited: 28
NeuralUQ: A Comprehensive Library for Uncertainty Quantification in Neural Differential Equations and Operators
Zongren Zou, Xuhui Meng, Apostolos F. Psaros, et al.
SIAM Review (2024) Vol. 66, Iss. 1, pp. 161-190
Open Access | Times Cited: 23
Zongren Zou, Xuhui Meng, Apostolos F. Psaros, et al.
SIAM Review (2024) Vol. 66, Iss. 1, pp. 161-190
Open Access | Times Cited: 23
Weak baselines and reporting biases lead to overoptimism in machine learning for fluid-related partial differential equations
Nick McGreivy, Ammar Hakim
Nature Machine Intelligence (2024) Vol. 6, Iss. 10, pp. 1256-1269
Closed Access | Times Cited: 23
Nick McGreivy, Ammar Hakim
Nature Machine Intelligence (2024) Vol. 6, Iss. 10, pp. 1256-1269
Closed Access | Times Cited: 23
Multilevel domain decomposition-based architectures for physics-informed neural networks
Victorita Dolean, Alexander Heinlein, Siddhartha Mishra, et al.
Computer Methods in Applied Mechanics and Engineering (2024) Vol. 429, pp. 117116-117116
Open Access | Times Cited: 22
Victorita Dolean, Alexander Heinlein, Siddhartha Mishra, et al.
Computer Methods in Applied Mechanics and Engineering (2024) Vol. 429, pp. 117116-117116
Open Access | Times Cited: 22
Multi-resolution partial differential equations preserved learning framework for spatiotemporal dynamics
Xin‐Yang Liu, Min Zhu, Lu Lu, et al.
Communications Physics (2024) Vol. 7, Iss. 1
Open Access | Times Cited: 19
Xin‐Yang Liu, Min Zhu, Lu Lu, et al.
Communications Physics (2024) Vol. 7, Iss. 1
Open Access | Times Cited: 19
Towards chemical source tracking and characterization using physics-informed neural networks
Forouzan Naderi, Issac Perez-Raya, Sangeeta Yadav, et al.
Atmospheric Environment (2024) Vol. 334, pp. 120679-120679
Closed Access | Times Cited: 18
Forouzan Naderi, Issac Perez-Raya, Sangeeta Yadav, et al.
Atmospheric Environment (2024) Vol. 334, pp. 120679-120679
Closed Access | Times Cited: 18