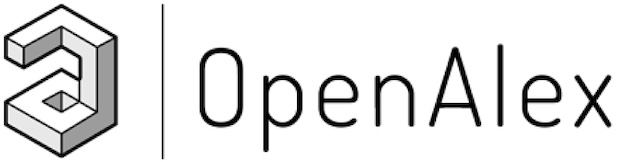
OpenAlex is a bibliographic catalogue of scientific papers, authors and institutions accessible in open access mode, named after the Library of Alexandria. It's citation coverage is excellent and I hope you will find utility in this listing of citing articles!
If you click the article title, you'll navigate to the article, as listed in CrossRef. If you click the Open Access links, you'll navigate to the "best Open Access location". Clicking the citation count will open this listing for that article. Lastly at the bottom of the page, you'll find basic pagination options.
Requested Article:
DL-m6A: Identification of N6-Methyladenosine Sites in Mammals Using Deep Learning Based on Different Encoding Schemes
Mobeen Ur Rehman, Hilal Tayara, Kil To Chong
IEEE/ACM Transactions on Computational Biology and Bioinformatics (2022) Vol. 20, Iss. 2, pp. 904-911
Closed Access | Times Cited: 30
Mobeen Ur Rehman, Hilal Tayara, Kil To Chong
IEEE/ACM Transactions on Computational Biology and Bioinformatics (2022) Vol. 20, Iss. 2, pp. 904-911
Closed Access | Times Cited: 30
Showing 1-25 of 30 citing articles:
Attention-Based Graph Neural Network for Molecular Solubility Prediction
Waqar Ahmad, Hilal Tayara, Kil To Chong
ACS Omega (2023) Vol. 8, Iss. 3, pp. 3236-3244
Open Access | Times Cited: 31
Waqar Ahmad, Hilal Tayara, Kil To Chong
ACS Omega (2023) Vol. 8, Iss. 3, pp. 3236-3244
Open Access | Times Cited: 31
IF-AIP: A machine learning method for the identification of anti-inflammatory peptides using multi-feature fusion strategy
Saima Gaffar, Mir Tanveerul Hassan, Hilal Tayara, et al.
Computers in Biology and Medicine (2023) Vol. 168, pp. 107724-107724
Closed Access | Times Cited: 21
Saima Gaffar, Mir Tanveerul Hassan, Hilal Tayara, et al.
Computers in Biology and Medicine (2023) Vol. 168, pp. 107724-107724
Closed Access | Times Cited: 21
Prediction of organic material band gaps using graph attention network
Asad Khan, Hilal Tayara, Kil To Chong
Computational Materials Science (2023) Vol. 220, pp. 112063-112063
Closed Access | Times Cited: 13
Asad Khan, Hilal Tayara, Kil To Chong
Computational Materials Science (2023) Vol. 220, pp. 112063-112063
Closed Access | Times Cited: 13
Improved Image Quality Assessment by Utilizing Pre-Trained Architecture Features with Unified Learning Mechanism
Jihyoung Ryu
Applied Sciences (2023) Vol. 13, Iss. 4, pp. 2682-2682
Open Access | Times Cited: 13
Jihyoung Ryu
Applied Sciences (2023) Vol. 13, Iss. 4, pp. 2682-2682
Open Access | Times Cited: 13
Capturing short-range and long-range dependencies of nucleotides for identifying RNA N6-methyladenosine modification sites
Guodong Li, Bo-Wei Zhao, Xiaorui Su, et al.
Computers in Biology and Medicine (2025) Vol. 186, pp. 109625-109625
Closed Access
Guodong Li, Bo-Wei Zhao, Xiaorui Su, et al.
Computers in Biology and Medicine (2025) Vol. 186, pp. 109625-109625
Closed Access
Using statistical analysis to explore the influencing factors of data imbalance for machine learning identification methods of human transcriptome m6A modification sites
Mingxin Li, Rujun Li, Yichi Zhang, et al.
Computational Biology and Chemistry (2025) Vol. 115, pp. 108351-108351
Closed Access
Mingxin Li, Rujun Li, Yichi Zhang, et al.
Computational Biology and Chemistry (2025) Vol. 115, pp. 108351-108351
Closed Access
Injecting structure-aware insights for the learning of RNA sequence representations to identify m6A modification sites
Yue Yu, Shuang Xiang, Ming‐Hao Wu
PeerJ (2025) Vol. 13, pp. e18878-e18878
Open Access
Yue Yu, Shuang Xiang, Ming‐Hao Wu
PeerJ (2025) Vol. 13, pp. e18878-e18878
Open Access
Drugs inhibition prediction in P-gp enzyme: a comparative study of machine learning and graph neural network
Maryam Maryam, Mobeen Ur Rehman, Kil To Chong, et al.
Computational Toxicology (2025), pp. 100344-100344
Closed Access
Maryam Maryam, Mobeen Ur Rehman, Kil To Chong, et al.
Computational Toxicology (2025), pp. 100344-100344
Closed Access
MRM-BERT: a novel deep neural network predictor of multiple RNA modifications by fusing BERT representation and sequence features
Linshu Wang, Yuan Zhou
RNA Biology (2024) Vol. 21, Iss. 1, pp. 1-10
Open Access | Times Cited: 3
Linshu Wang, Yuan Zhou
RNA Biology (2024) Vol. 21, Iss. 1, pp. 1-10
Open Access | Times Cited: 3
m6Aexpress-enet: Predicting the regulatory expression m6A sites by an enet-regularization negative binomial regression model
Teng Zhang, Shang Gao, Shao‐Wu Zhang, et al.
Methods (2024) Vol. 226, pp. 61-70
Closed Access | Times Cited: 2
Teng Zhang, Shang Gao, Shao‐Wu Zhang, et al.
Methods (2024) Vol. 226, pp. 61-70
Closed Access | Times Cited: 2
NaII-Pred: An ensemble-learning framework for the identification and interpretation of sodium ion inhibitors by fusing multiple feature representation
Mir Tanveerul Hassan, Hilal Tayara, Kil To Chong
Computers in Biology and Medicine (2024) Vol. 178, pp. 108737-108737
Closed Access | Times Cited: 2
Mir Tanveerul Hassan, Hilal Tayara, Kil To Chong
Computers in Biology and Medicine (2024) Vol. 178, pp. 108737-108737
Closed Access | Times Cited: 2
Enhancing Taxonomic Categorization of DNA Sequences with Deep Learning: A Multi-Label Approach
Prommy Sultana Hossain, Kyungsup Kim, Jia Uddin, et al.
Bioengineering (2023) Vol. 10, Iss. 11, pp. 1293-1293
Open Access | Times Cited: 6
Prommy Sultana Hossain, Kyungsup Kim, Jia Uddin, et al.
Bioengineering (2023) Vol. 10, Iss. 11, pp. 1293-1293
Open Access | Times Cited: 6
XML-CIMT: Explainable Machine Learning (XML) Model for Predicting Chemical-Induced Mitochondrial Toxicity
Keerthana Jaganathan, Mobeen Ur Rehman, Hilal Tayara, et al.
International Journal of Molecular Sciences (2022) Vol. 23, Iss. 24, pp. 15655-15655
Open Access | Times Cited: 9
Keerthana Jaganathan, Mobeen Ur Rehman, Hilal Tayara, et al.
International Journal of Molecular Sciences (2022) Vol. 23, Iss. 24, pp. 15655-15655
Open Access | Times Cited: 9
BiPSTP: Sequence feature encoding method for identifying different RNA modifications with bidirectional position-specific trinucleotides propensities
Mingzhao Wang, Haider Ali, Yandi Xu, et al.
Journal of Biological Chemistry (2024) Vol. 300, Iss. 4, pp. 107140-107140
Open Access | Times Cited: 1
Mingzhao Wang, Haider Ali, Yandi Xu, et al.
Journal of Biological Chemistry (2024) Vol. 300, Iss. 4, pp. 107140-107140
Open Access | Times Cited: 1
SB-Net: Synergizing CNN and LSTM networks for uncovering retrosynthetic pathways in organic synthesis
Bilal Ahmad Mir, Hilal Tayara, Kil To Chong
Computational Biology and Chemistry (2024) Vol. 112, pp. 108130-108130
Closed Access | Times Cited: 1
Bilal Ahmad Mir, Hilal Tayara, Kil To Chong
Computational Biology and Chemistry (2024) Vol. 112, pp. 108130-108130
Closed Access | Times Cited: 1
m5C-Seq: Machine learning-enhanced profiling of RNA 5-methylcytosine modifications
Zeeshan Abbas, Mobeen Ur Rehman, Hilal Tayara, et al.
Computers in Biology and Medicine (2024) Vol. 182, pp. 109087-109087
Closed Access | Times Cited: 1
Zeeshan Abbas, Mobeen Ur Rehman, Hilal Tayara, et al.
Computers in Biology and Medicine (2024) Vol. 182, pp. 109087-109087
Closed Access | Times Cited: 1
Multi-kernel feature extraction with dynamic fusion and downsampled residual feature embedding for predicting rice RNA N6-methyladenine sites
Mengya Liu, Zhan-Li Sun, Zhigang Zeng, et al.
Briefings in Bioinformatics (2024) Vol. 26, Iss. 1
Open Access | Times Cited: 1
Mengya Liu, Zhan-Li Sun, Zhigang Zeng, et al.
Briefings in Bioinformatics (2024) Vol. 26, Iss. 1
Open Access | Times Cited: 1
GR-m6A: Prediction of N6-methyladenosine sites in mammals with molecular graph and residual network
Shi Qiu, Renxin Liu, Ying Liang
Computers in Biology and Medicine (2023) Vol. 163, pp. 107202-107202
Closed Access | Times Cited: 4
Shi Qiu, Renxin Liu, Ying Liang
Computers in Biology and Medicine (2023) Vol. 163, pp. 107202-107202
Closed Access | Times Cited: 4
iCpG-Pos: an accurate computational approach for identification of CpG sites using positional features on single-cell whole genome sequence data
Sehi Park, Mobeen Ur Rehman, Farman Ullah, et al.
Bioinformatics (2023) Vol. 39, Iss. 8
Open Access | Times Cited: 4
Sehi Park, Mobeen Ur Rehman, Farman Ullah, et al.
Bioinformatics (2023) Vol. 39, Iss. 8
Open Access | Times Cited: 4
Cancer Diagnosis through Contour Visualization of Gene Expression Leveraging Deep Learning Techniques
Vinoth Kumar Venkatesan, K. M. Karthick Raghunath, Kaladevi Amarakundhi Chandrasekaran, et al.
Diagnostics (2023) Vol. 13, Iss. 22, pp. 3452-3452
Open Access | Times Cited: 4
Vinoth Kumar Venkatesan, K. M. Karthick Raghunath, Kaladevi Amarakundhi Chandrasekaran, et al.
Diagnostics (2023) Vol. 13, Iss. 22, pp. 3452-3452
Open Access | Times Cited: 4
Dynamic regulation and key roles of ribonucleic acid methylation
Jia Zou, Hui Liu, Wei Tan, et al.
Frontiers in Cellular Neuroscience (2022) Vol. 16
Open Access | Times Cited: 7
Jia Zou, Hui Liu, Wei Tan, et al.
Frontiers in Cellular Neuroscience (2022) Vol. 16
Open Access | Times Cited: 7
Adaptive Feature Fusion and Kernel-Based Regression Modeling to Improve Blind Image Quality Assessment
Jihyoung Ryu
Applied Sciences (2023) Vol. 13, Iss. 13, pp. 7522-7522
Open Access | Times Cited: 3
Jihyoung Ryu
Applied Sciences (2023) Vol. 13, Iss. 13, pp. 7522-7522
Open Access | Times Cited: 3
EMDL_m6Am: identifying N6,2′-O-dimethyladenosine sites based on stacking ensemble deep learning
Jianhua Jia, Zhangying Wei, Mingwei Sun
BMC Bioinformatics (2023) Vol. 24, Iss. 1
Open Access | Times Cited: 2
Jianhua Jia, Zhangying Wei, Mingwei Sun
BMC Bioinformatics (2023) Vol. 24, Iss. 1
Open Access | Times Cited: 2
A Study of Neural Network Framework for Power Generation Prediction of a Solar Power Plant
Jeehong Kim, Seok-ho Lee, Kil To Chong
Energies (2022) Vol. 15, Iss. 22, pp. 8582-8582
Open Access | Times Cited: 3
Jeehong Kim, Seok-ho Lee, Kil To Chong
Energies (2022) Vol. 15, Iss. 22, pp. 8582-8582
Open Access | Times Cited: 3
TC-6mA-Pred: Prediction of DNA N6-Methyladenine Sites Using CNN with Transformer
Zeeshan Abbas, Mobeen Ur Rehman, Kil To Chong
2021 IEEE International Conference on Bioinformatics and Biomedicine (BIBM) (2022), pp. 2506-2510
Closed Access | Times Cited: 3
Zeeshan Abbas, Mobeen Ur Rehman, Kil To Chong
2021 IEEE International Conference on Bioinformatics and Biomedicine (BIBM) (2022), pp. 2506-2510
Closed Access | Times Cited: 3