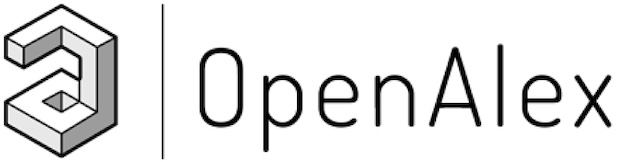
OpenAlex is a bibliographic catalogue of scientific papers, authors and institutions accessible in open access mode, named after the Library of Alexandria. It's citation coverage is excellent and I hope you will find utility in this listing of citing articles!
If you click the article title, you'll navigate to the article, as listed in CrossRef. If you click the Open Access links, you'll navigate to the "best Open Access location". Clicking the citation count will open this listing for that article. Lastly at the bottom of the page, you'll find basic pagination options.
Requested Article:
Overcoming Exploration in Reinforcement Learning with Demonstrations
Ashvin Nair, Bob McGrew, Marcin Andrychowicz, et al.
(2018), pp. 6292-6299
Open Access | Times Cited: 638
Ashvin Nair, Bob McGrew, Marcin Andrychowicz, et al.
(2018), pp. 6292-6299
Open Access | Times Cited: 638
Showing 1-25 of 638 citing articles:
Grandmaster level in StarCraft II using multi-agent reinforcement learning
Oriol Vinyals, I. Babuschkin, Wojciech Marian Czarnecki, et al.
Nature (2019) Vol. 575, Iss. 7782, pp. 350-354
Closed Access | Times Cited: 2859
Oriol Vinyals, I. Babuschkin, Wojciech Marian Czarnecki, et al.
Nature (2019) Vol. 575, Iss. 7782, pp. 350-354
Closed Access | Times Cited: 2859
Learning Complex Dexterous Manipulation with Deep Reinforcement Learning and Demonstrations
Aravind Rajeswaran, Vikash Kumar, Abhishek Gupta, et al.
(2018)
Open Access | Times Cited: 648
Aravind Rajeswaran, Vikash Kumar, Abhishek Gupta, et al.
(2018)
Open Access | Times Cited: 648
Continuous control for robot based on deep reinforcement learning
Shansi Zhang
(2019)
Open Access | Times Cited: 485
Shansi Zhang
(2019)
Open Access | Times Cited: 485
Recent Advances in Robot Learning from Demonstration
Harish Ravichandar, Athanasios Polydoros, Sonia Chernova, et al.
Annual Review of Control Robotics and Autonomous Systems (2019) Vol. 3, Iss. 1, pp. 297-330
Open Access | Times Cited: 475
Harish Ravichandar, Athanasios Polydoros, Sonia Chernova, et al.
Annual Review of Control Robotics and Autonomous Systems (2019) Vol. 3, Iss. 1, pp. 297-330
Open Access | Times Cited: 475
How to train your robot with deep reinforcement learning: lessons we have learned
Julian Ibarz, Jie Tan, Chelsea Finn, et al.
The International Journal of Robotics Research (2021) Vol. 40, Iss. 4-5, pp. 698-721
Open Access | Times Cited: 380
Julian Ibarz, Jie Tan, Chelsea Finn, et al.
The International Journal of Robotics Research (2021) Vol. 40, Iss. 4-5, pp. 698-721
Open Access | Times Cited: 380
Residual Reinforcement Learning for Robot Control
Tobias Johannink, Shikhar Bahl, Ashvin Nair, et al.
2022 International Conference on Robotics and Automation (ICRA) (2019), pp. 6023-6029
Open Access | Times Cited: 336
Tobias Johannink, Shikhar Bahl, Ashvin Nair, et al.
2022 International Conference on Robotics and Automation (ICRA) (2019), pp. 6023-6029
Open Access | Times Cited: 336
Transfer Learning in Deep Reinforcement Learning: A Survey
Zhuangdi Zhu, Kaixiang Lin, Anil K. Jain, et al.
IEEE Transactions on Pattern Analysis and Machine Intelligence (2023) Vol. 45, Iss. 11, pp. 13344-13362
Open Access | Times Cited: 293
Zhuangdi Zhu, Kaixiang Lin, Anil K. Jain, et al.
IEEE Transactions on Pattern Analysis and Machine Intelligence (2023) Vol. 45, Iss. 11, pp. 13344-13362
Open Access | Times Cited: 293
Off-Policy Deep Reinforcement Learning without Exploration
Scott Fujimoto, David Meger, Doina Precup
arXiv (Cornell University) (2018)
Open Access | Times Cited: 225
Scott Fujimoto, David Meger, Doina Precup
arXiv (Cornell University) (2018)
Open Access | Times Cited: 225
Exploration in deep reinforcement learning: A survey
Paweł Ładosz, Lilian Weng, Minwoo Kim, et al.
Information Fusion (2022) Vol. 85, pp. 1-22
Open Access | Times Cited: 223
Paweł Ładosz, Lilian Weng, Minwoo Kim, et al.
Information Fusion (2022) Vol. 85, pp. 1-22
Open Access | Times Cited: 223
First return, then explore
Adrien Ecoffet, Joost Huizinga, Joel Lehman, et al.
Nature (2021) Vol. 590, Iss. 7847, pp. 580-586
Closed Access | Times Cited: 201
Adrien Ecoffet, Joost Huizinga, Joel Lehman, et al.
Nature (2021) Vol. 590, Iss. 7847, pp. 580-586
Closed Access | Times Cited: 201
Reinforcement and Imitation Learning for Diverse Visuomotor Skills
Yuke Zhu, Ziyu Wang, Josh Merel, et al.
(2018)
Open Access | Times Cited: 199
Yuke Zhu, Ziyu Wang, Josh Merel, et al.
(2018)
Open Access | Times Cited: 199
Deep reinforcement learning in computer vision: a comprehensive survey
Ngan Le, Vidhiwar Singh Rathour, Kashu Yamazaki, et al.
Artificial Intelligence Review (2021) Vol. 55, Iss. 4, pp. 2733-2819
Open Access | Times Cited: 155
Ngan Le, Vidhiwar Singh Rathour, Kashu Yamazaki, et al.
Artificial Intelligence Review (2021) Vol. 55, Iss. 4, pp. 2733-2819
Open Access | Times Cited: 155
Parametric study on reinforcement learning optimized energy management strategy for a hybrid electric vehicle
Bin Xu, Dhruvang Rathod, Darui Zhang, et al.
Applied Energy (2019) Vol. 259, pp. 114200-114200
Closed Access | Times Cited: 154
Bin Xu, Dhruvang Rathod, Darui Zhang, et al.
Applied Energy (2019) Vol. 259, pp. 114200-114200
Closed Access | Times Cited: 154
Deep Reinforcement Learning for Industrial Insertion Tasks with Visual Inputs and Natural Rewards
Gerrit Schoettler, Ashvin Nair, Jianlan Luo, et al.
2021 IEEE/RSJ International Conference on Intelligent Robots and Systems (IROS) (2020), pp. 5548-5555
Open Access | Times Cited: 152
Gerrit Schoettler, Ashvin Nair, Jianlan Luo, et al.
2021 IEEE/RSJ International Conference on Intelligent Robots and Systems (IROS) (2020), pp. 5548-5555
Open Access | Times Cited: 152
Dexterous Manipulation with Deep Reinforcement Learning: Efficient, General, and Low-Cost
Henry Zhu, Abhishek Gupta, Aravind Rajeswaran, et al.
2022 International Conference on Robotics and Automation (ICRA) (2019), pp. 3651-3657
Open Access | Times Cited: 149
Henry Zhu, Abhishek Gupta, Aravind Rajeswaran, et al.
2022 International Conference on Robotics and Automation (ICRA) (2019), pp. 3651-3657
Open Access | Times Cited: 149
Deep reinforcement learning in medical imaging: A literature review
S. Kevin Zhou, Ngan Le, Khoa Luu, et al.
Medical Image Analysis (2021) Vol. 73, pp. 102193-102193
Open Access | Times Cited: 149
S. Kevin Zhou, Ngan Le, Khoa Luu, et al.
Medical Image Analysis (2021) Vol. 73, pp. 102193-102193
Open Access | Times Cited: 149
A Minimalist Approach to Offline Reinforcement Learning
Scott Fujimoto, Shixiang Gu
arXiv (Cornell University) (2021)
Open Access | Times Cited: 133
Scott Fujimoto, Shixiang Gu
arXiv (Cornell University) (2021)
Open Access | Times Cited: 133
A Survey on Deep Reinforcement Learning Algorithms for Robotic Manipulation
Dong Han, Beni Mulyana, Vladimir Stanković, et al.
Sensors (2023) Vol. 23, Iss. 7, pp. 3762-3762
Open Access | Times Cited: 84
Dong Han, Beni Mulyana, Vladimir Stanković, et al.
Sensors (2023) Vol. 23, Iss. 7, pp. 3762-3762
Open Access | Times Cited: 84
Human-in-the-Loop Reinforcement Learning: A Survey and Position on Requirements, Challenges, and Opportunities
Carl Orge Retzlaff, Srijita Das, Christabel Wayllace, et al.
Journal of Artificial Intelligence Research (2024) Vol. 79, pp. 359-415
Open Access | Times Cited: 28
Carl Orge Retzlaff, Srijita Das, Christabel Wayllace, et al.
Journal of Artificial Intelligence Research (2024) Vol. 79, pp. 359-415
Open Access | Times Cited: 28
Variable Compliance Control for Robotic Peg-in-Hole Assembly: A Deep-Reinforcement-Learning Approach
Cristian C. Beltran-Hernandez, Damien Petit, Ixchel G. Ramirez-Alpizar, et al.
Applied Sciences (2020) Vol. 10, Iss. 19, pp. 6923-6923
Open Access | Times Cited: 134
Cristian C. Beltran-Hernandez, Damien Petit, Ixchel G. Ramirez-Alpizar, et al.
Applied Sciences (2020) Vol. 10, Iss. 19, pp. 6923-6923
Open Access | Times Cited: 134
Reinforcement and Imitation Learning for Diverse Visuomotor Skills
Yuke Zhu, Ziyu Wang, Josh Merel, et al.
arXiv (Cornell University) (2018)
Open Access | Times Cited: 113
Yuke Zhu, Ziyu Wang, Josh Merel, et al.
arXiv (Cornell University) (2018)
Open Access | Times Cited: 113
Adaptive Quantitative Trading: An Imitative Deep Reinforcement Learning Approach
Yang Liu, Qi Liu, Hongke Zhao, et al.
Proceedings of the AAAI Conference on Artificial Intelligence (2020) Vol. 34, Iss. 02, pp. 2128-2135
Open Access | Times Cited: 110
Yang Liu, Qi Liu, Hongke Zhao, et al.
Proceedings of the AAAI Conference on Artificial Intelligence (2020) Vol. 34, Iss. 02, pp. 2128-2135
Open Access | Times Cited: 110
Transfer Learning in Deep Reinforcement Learning: A Survey
Zhuangdi Zhu, Kaixiang Lin, Jiayu Zhou
arXiv (Cornell University) (2020)
Open Access | Times Cited: 108
Zhuangdi Zhu, Kaixiang Lin, Jiayu Zhou
arXiv (Cornell University) (2020)
Open Access | Times Cited: 108
Deep Learning in Robotics: Survey on Model Structures and Training Strategies
Artúr I. Károly, Péter Galambos, József Kuti, et al.
IEEE Transactions on Systems Man and Cybernetics Systems (2020) Vol. 51, Iss. 1, pp. 266-279
Open Access | Times Cited: 106
Artúr I. Károly, Péter Galambos, József Kuti, et al.
IEEE Transactions on Systems Man and Cybernetics Systems (2020) Vol. 51, Iss. 1, pp. 266-279
Open Access | Times Cited: 106
A Practical Approach to Insertion with Variable Socket Position Using Deep Reinforcement Learning
Mel Vecerík, Oleg Sushkov, David Barker, et al.
2022 International Conference on Robotics and Automation (ICRA) (2019), pp. 754-760
Open Access | Times Cited: 104
Mel Vecerík, Oleg Sushkov, David Barker, et al.
2022 International Conference on Robotics and Automation (ICRA) (2019), pp. 754-760
Open Access | Times Cited: 104