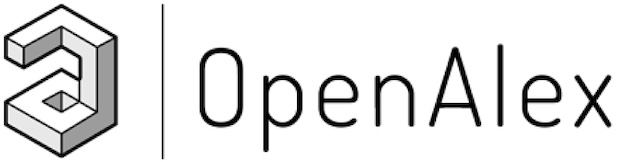
OpenAlex is a bibliographic catalogue of scientific papers, authors and institutions accessible in open access mode, named after the Library of Alexandria. It's citation coverage is excellent and I hope you will find utility in this listing of citing articles!
If you click the article title, you'll navigate to the article, as listed in CrossRef. If you click the Open Access links, you'll navigate to the "best Open Access location". Clicking the citation count will open this listing for that article. Lastly at the bottom of the page, you'll find basic pagination options.
Requested Article:
Leveraging big data for grasp planning
Daniel Kappler, Jeannette Bohg, Stefan Schaal
(2015), pp. 4304-4311
Closed Access | Times Cited: 274
Daniel Kappler, Jeannette Bohg, Stefan Schaal
(2015), pp. 4304-4311
Closed Access | Times Cited: 274
Showing 1-25 of 274 citing articles:
Learning hand-eye coordination for robotic grasping with deep learning and large-scale data collection
Sergey Levine, Peter Pástor, Alex Krizhevsky, et al.
The International Journal of Robotics Research (2017) Vol. 37, Iss. 4-5, pp. 421-436
Open Access | Times Cited: 1789
Sergey Levine, Peter Pástor, Alex Krizhevsky, et al.
The International Journal of Robotics Research (2017) Vol. 37, Iss. 4-5, pp. 421-436
Open Access | Times Cited: 1789
Supersizing self-supervision: Learning to grasp from 50K tries and 700 robot hours
Lerrel Pinto, Abhinav Gupta
(2016), pp. 3406-3413
Open Access | Times Cited: 1098
Lerrel Pinto, Abhinav Gupta
(2016), pp. 3406-3413
Open Access | Times Cited: 1098
Dex-Net 2.0: Deep Learning to Plan Robust Grasps with Synthetic Point Clouds and Analytic Grasp Metrics
Jeffrey Mahler, Jacky Liang, Sherdil Niyaz, et al.
(2017)
Open Access | Times Cited: 1056
Jeffrey Mahler, Jacky Liang, Sherdil Niyaz, et al.
(2017)
Open Access | Times Cited: 1056
Using Simulation and Domain Adaptation to Improve Efficiency of Deep Robotic Grasping
Konstantinos Bousmalis, Alex Irpan, Paul Wohlhart, et al.
(2018)
Open Access | Times Cited: 626
Konstantinos Bousmalis, Alex Irpan, Paul Wohlhart, et al.
(2018)
Open Access | Times Cited: 626
Learning ambidextrous robot grasping policies
Jeffrey Mahler, Matthew Matl, Vishal Satish, et al.
Science Robotics (2019) Vol. 4, Iss. 26
Closed Access | Times Cited: 487
Jeffrey Mahler, Matthew Matl, Vishal Satish, et al.
Science Robotics (2019) Vol. 4, Iss. 26
Closed Access | Times Cited: 487
Grasp Pose Detection in Point Clouds
Andreas ten Pas, Marcus Gualtieri, Kate Saenko, et al.
The International Journal of Robotics Research (2017) Vol. 36, Iss. 13-14, pp. 1455-1473
Open Access | Times Cited: 464
Andreas ten Pas, Marcus Gualtieri, Kate Saenko, et al.
The International Journal of Robotics Research (2017) Vol. 36, Iss. 13-14, pp. 1455-1473
Open Access | Times Cited: 464
Sim-To-Real via Sim-To-Sim: Data-Efficient Robotic Grasping via Randomized-To-Canonical Adaptation Networks
Stephen James, Paul Wohlhart, Mrinal Kalakrishnan, et al.
2022 IEEE/CVF Conference on Computer Vision and Pattern Recognition (CVPR) (2019), pp. 12619-12629
Closed Access | Times Cited: 366
Stephen James, Paul Wohlhart, Mrinal Kalakrishnan, et al.
2022 IEEE/CVF Conference on Computer Vision and Pattern Recognition (CVPR) (2019), pp. 12619-12629
Closed Access | Times Cited: 366
Dex-Net 1.0: A cloud-based network of 3D objects for robust grasp planning using a Multi-Armed Bandit model with correlated rewards
Jeffrey Mahler, Florian T. Pokorny, Brian Hou, et al.
(2016), pp. 1957-1964
Closed Access | Times Cited: 354
Jeffrey Mahler, Florian T. Pokorny, Brian Hou, et al.
(2016), pp. 1957-1964
Closed Access | Times Cited: 354
High precision grasp pose detection in dense clutter
Marcus Gualtieri, Andreas ten Pas, Kate Saenko, et al.
2021 IEEE/RSJ International Conference on Intelligent Robots and Systems (IROS) (2016), pp. 598-605
Open Access | Times Cited: 284
Marcus Gualtieri, Andreas ten Pas, Kate Saenko, et al.
2021 IEEE/RSJ International Conference on Intelligent Robots and Systems (IROS) (2016), pp. 598-605
Open Access | Times Cited: 284
Dex-Net 3.0: Computing Robust Vacuum Suction Grasp Targets in Point Clouds Using a New Analytic Model and Deep Learning
Jeffrey Mahler, Matthew Matl, Xinyu Liu, et al.
(2018), pp. 5620-5627
Closed Access | Times Cited: 284
Jeffrey Mahler, Matthew Matl, Xinyu Liu, et al.
(2018), pp. 5620-5627
Closed Access | Times Cited: 284
Shape completion enabled robotic grasping
Jacob Varley, Chad DeChant, Adam Richardson, et al.
2021 IEEE/RSJ International Conference on Intelligent Robots and Systems (IROS) (2017), pp. 2442-2447
Open Access | Times Cited: 282
Jacob Varley, Chad DeChant, Adam Richardson, et al.
2021 IEEE/RSJ International Conference on Intelligent Robots and Systems (IROS) (2017), pp. 2442-2447
Open Access | Times Cited: 282
Learning Hand-Eye Coordination for Robotic Grasping with Large-Scale Data Collection
Sergey Levine, Peter Pástor, Alex Krizhevsky, et al.
Springer proceedings in advanced robotics (2017), pp. 173-184
Closed Access | Times Cited: 278
Sergey Levine, Peter Pástor, Alex Krizhevsky, et al.
Springer proceedings in advanced robotics (2017), pp. 173-184
Closed Access | Times Cited: 278
Deep learning in robotics: a review of recent research
Harry A. Pierson, Michael S. Gashler
Advanced Robotics (2017) Vol. 31, Iss. 16, pp. 821-835
Open Access | Times Cited: 268
Harry A. Pierson, Michael S. Gashler
Advanced Robotics (2017) Vol. 31, Iss. 16, pp. 821-835
Open Access | Times Cited: 268
Meta-World: A Benchmark and Evaluation for Multi-Task and Meta Reinforcement Learning
Tianhe Yu, Deirdre Quillen, Zhanpeng He, et al.
arXiv (Cornell University) (2019)
Open Access | Times Cited: 247
Tianhe Yu, Deirdre Quillen, Zhanpeng He, et al.
arXiv (Cornell University) (2019)
Open Access | Times Cited: 247
Deep learning a grasp function for grasping under gripper pose uncertainty
Edward Johns, Stefan Leutenegger, Andrew J. Davison
2021 IEEE/RSJ International Conference on Intelligent Robots and Systems (IROS) (2016), pp. 4461-4468
Open Access | Times Cited: 231
Edward Johns, Stefan Leutenegger, Andrew J. Davison
2021 IEEE/RSJ International Conference on Intelligent Robots and Systems (IROS) (2016), pp. 4461-4468
Open Access | Times Cited: 231
Deep Reinforcement Learning for Vision-Based Robotic Grasping: A Simulated Comparative Evaluation of Off-Policy Methods
Deirdre Quillen, Eric Jang, Ofir Nachum, et al.
(2018)
Open Access | Times Cited: 208
Deirdre Quillen, Eric Jang, Ofir Nachum, et al.
(2018)
Open Access | Times Cited: 208
Object Recognition Using Tactile Measurements: Kernel Sparse Coding Methods
Huaping Liu, Di Guo, Fuchun Sun
IEEE Transactions on Instrumentation and Measurement (2016) Vol. 65, Iss. 3, pp. 656-665
Closed Access | Times Cited: 180
Huaping Liu, Di Guo, Fuchun Sun
IEEE Transactions on Instrumentation and Measurement (2016) Vol. 65, Iss. 3, pp. 656-665
Closed Access | Times Cited: 180
6-DOF Grasping for Target-driven Object Manipulation in Clutter
Adithyavairavan Murali, Arsalan Mousavian, Clemens Eppner, et al.
(2020), pp. 6232-6238
Open Access | Times Cited: 173
Adithyavairavan Murali, Arsalan Mousavian, Clemens Eppner, et al.
(2020), pp. 6232-6238
Open Access | Times Cited: 173
Learning task-oriented grasping for tool manipulation from simulated self-supervision
Kuan Fang, Yuke Zhu, Animesh Garg, et al.
The International Journal of Robotics Research (2019) Vol. 39, Iss. 2-3, pp. 202-216
Open Access | Times Cited: 156
Kuan Fang, Yuke Zhu, Animesh Garg, et al.
The International Journal of Robotics Research (2019) Vol. 39, Iss. 2-3, pp. 202-216
Open Access | Times Cited: 156
Deep Learning Approaches to Grasp Synthesis: A Review
R. Newbury, Morris Gu, Lachlan Chumbley, et al.
IEEE Transactions on Robotics (2023) Vol. 39, Iss. 5, pp. 3994-4015
Open Access | Times Cited: 91
R. Newbury, Morris Gu, Lachlan Chumbley, et al.
IEEE Transactions on Robotics (2023) Vol. 39, Iss. 5, pp. 3994-4015
Open Access | Times Cited: 91
Learning high-DOF reaching-and-grasping via dynamic representation of gripper-object interaction
Qijin She, Ruizhen Hu, Juzhan Xu, et al.
ACM Transactions on Graphics (2022) Vol. 41, Iss. 4, pp. 1-14
Closed Access | Times Cited: 76
Qijin She, Ruizhen Hu, Juzhan Xu, et al.
ACM Transactions on Graphics (2022) Vol. 41, Iss. 4, pp. 1-14
Closed Access | Times Cited: 76
Learning Object Grasping for Soft Robot Hands
Changhyun Choi, Wilko Schwarting, Joseph DelPreto, et al.
IEEE Robotics and Automation Letters (2018) Vol. 3, Iss. 3, pp. 2370-2377
Open Access | Times Cited: 158
Changhyun Choi, Wilko Schwarting, Joseph DelPreto, et al.
IEEE Robotics and Automation Letters (2018) Vol. 3, Iss. 3, pp. 2370-2377
Open Access | Times Cited: 158
More Than a Feeling: Learning to Grasp and Regrasp Using Vision and Touch
Roberto Calandra, Andrew Owens, Dinesh Jayaraman, et al.
IEEE Robotics and Automation Letters (2018) Vol. 3, Iss. 4, pp. 3300-3307
Open Access | Times Cited: 157
Roberto Calandra, Andrew Owens, Dinesh Jayaraman, et al.
IEEE Robotics and Automation Letters (2018) Vol. 3, Iss. 4, pp. 3300-3307
Open Access | Times Cited: 157
Learning Deep Policies for Robot Bin Picking by Simulating Robust Grasping Sequences
Jeffrey Mahler, Ken Goldberg
Conference on Robot Learning (2017), pp. 515-524
Closed Access | Times Cited: 147
Jeffrey Mahler, Ken Goldberg
Conference on Robot Learning (2017), pp. 515-524
Closed Access | Times Cited: 147
Learning Hand-Eye Coordination for Robotic Grasping with Deep Learning and Large-Scale Data Collection
Sergey Levine, Peter Pástor, Alex Krizhevsky, et al.
arXiv (Cornell University) (2016)
Closed Access | Times Cited: 146
Sergey Levine, Peter Pástor, Alex Krizhevsky, et al.
arXiv (Cornell University) (2016)
Closed Access | Times Cited: 146