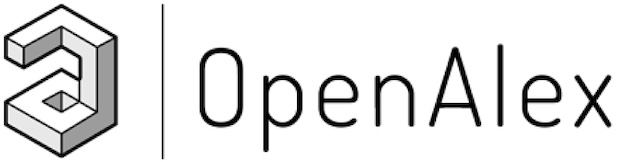
OpenAlex is a bibliographic catalogue of scientific papers, authors and institutions accessible in open access mode, named after the Library of Alexandria. It's citation coverage is excellent and I hope you will find utility in this listing of citing articles!
If you click the article title, you'll navigate to the article, as listed in CrossRef. If you click the Open Access links, you'll navigate to the "best Open Access location". Clicking the citation count will open this listing for that article. Lastly at the bottom of the page, you'll find basic pagination options.
Requested Article:
Flow-based sampling for fermionic lattice field theories
Michael S. Albergo, Gurtej Kanwar, Sébastien Racanière, et al.
Physical review. D/Physical review. D. (2021) Vol. 104, Iss. 11
Open Access | Times Cited: 49
Michael S. Albergo, Gurtej Kanwar, Sébastien Racanière, et al.
Physical review. D/Physical review. D. (2021) Vol. 104, Iss. 11
Open Access | Times Cited: 49
Showing 1-25 of 49 citing articles:
Colloquium: Machine learning in nuclear physics
A. Boehnlein, Markus Diefenthaler, N. Sato, et al.
Reviews of Modern Physics (2022) Vol. 94, Iss. 3
Open Access | Times Cited: 143
A. Boehnlein, Markus Diefenthaler, N. Sato, et al.
Reviews of Modern Physics (2022) Vol. 94, Iss. 3
Open Access | Times Cited: 143
Gauge-equivariant flow models for sampling in lattice field theories with pseudofermions
Ryan Abbott, Michael S. Albergo, Denis Boyda, et al.
Physical review. D/Physical review. D. (2022) Vol. 106, Iss. 7
Open Access | Times Cited: 39
Ryan Abbott, Michael S. Albergo, Denis Boyda, et al.
Physical review. D/Physical review. D. (2022) Vol. 106, Iss. 7
Open Access | Times Cited: 39
Matrix-Model Simulations Using Quantum Computing, Deep Learning, and Lattice Monte Carlo
Enrico Rinaldi, Xizhi Han, Mohammad Hassan, et al.
PRX Quantum (2022) Vol. 3, Iss. 1
Open Access | Times Cited: 38
Enrico Rinaldi, Xizhi Han, Mohammad Hassan, et al.
PRX Quantum (2022) Vol. 3, Iss. 1
Open Access | Times Cited: 38
Exploring QCD matter in extreme conditions with Machine Learning
Kai Zhou, Lingxiao Wang, Long-Gang Pang, et al.
Progress in Particle and Nuclear Physics (2023) Vol. 135, pp. 104084-104084
Open Access | Times Cited: 37
Kai Zhou, Lingxiao Wang, Long-Gang Pang, et al.
Progress in Particle and Nuclear Physics (2023) Vol. 135, pp. 104084-104084
Open Access | Times Cited: 37
Learning trivializing gradient flows for lattice gauge theories
Simone Bacchio, Pan Kessel, Stefan Schaefer, et al.
Physical review. D/Physical review. D. (2023) Vol. 107, Iss. 5
Open Access | Times Cited: 26
Simone Bacchio, Pan Kessel, Stefan Schaefer, et al.
Physical review. D/Physical review. D. (2023) Vol. 107, Iss. 5
Open Access | Times Cited: 26
Conditional normalizing flow for Markov chain Monte Carlo sampling in the critical region of lattice field theory
Ankur Singha, Dipankar Chakrabarti, Vipul Arora
Physical review. D/Physical review. D. (2023) Vol. 107, Iss. 1
Open Access | Times Cited: 23
Ankur Singha, Dipankar Chakrabarti, Vipul Arora
Physical review. D/Physical review. D. (2023) Vol. 107, Iss. 1
Open Access | Times Cited: 23
Aspects of scaling and scalability for flow-based sampling of lattice QCD
Ryan Abbott, Michael S. Albergo, Aleksandar Botev, et al.
The European Physical Journal A (2023) Vol. 59, Iss. 11
Open Access | Times Cited: 23
Ryan Abbott, Michael S. Albergo, Aleksandar Botev, et al.
The European Physical Journal A (2023) Vol. 59, Iss. 11
Open Access | Times Cited: 23
Efficient modeling of trivializing maps for lattice ϕ 4 theory using normalizing flows: A first look at scalability
Luigi Del Debbio, Joe Marsh Rossney, Michael G. Wilson
Physical review. D/Physical review. D. (2021) Vol. 104, Iss. 9
Open Access | Times Cited: 43
Luigi Del Debbio, Joe Marsh Rossney, Michael G. Wilson
Physical review. D/Physical review. D. (2021) Vol. 104, Iss. 9
Open Access | Times Cited: 43
Stochastic normalizing flows as non-equilibrium transformations
Michele Caselle, Elia Cellini, Alessandro Nada, et al.
Journal of High Energy Physics (2022) Vol. 2022, Iss. 7
Open Access | Times Cited: 33
Michele Caselle, Elia Cellini, Alessandro Nada, et al.
Journal of High Energy Physics (2022) Vol. 2022, Iss. 7
Open Access | Times Cited: 33
Flow-based sampling in the lattice Schwinger model at criticality
Michael S. Albergo, Denis Boyda, K. Cranmer, et al.
Physical review. D/Physical review. D. (2022) Vol. 106, Iss. 1
Open Access | Times Cited: 33
Michael S. Albergo, Denis Boyda, K. Cranmer, et al.
Physical review. D/Physical review. D. (2022) Vol. 106, Iss. 1
Open Access | Times Cited: 33
Advances in machine-learning-based sampling motivated by lattice quantum chromodynamics
K. Cranmer, Gurtej Kanwar, Sébastien Racanière, et al.
Nature Reviews Physics (2023) Vol. 5, Iss. 9, pp. 526-535
Open Access | Times Cited: 19
K. Cranmer, Gurtej Kanwar, Sébastien Racanière, et al.
Nature Reviews Physics (2023) Vol. 5, Iss. 9, pp. 526-535
Open Access | Times Cited: 19
Sampling QCD field configurations with gauge-equivariant flow models
Phiala E. Shanahan, Ryan Abbott, Michael S. Albergo, et al.
Proceedings of The 39th International Symposium on Lattice Field Theory — PoS(LATTICE2022) (2023)
Open Access | Times Cited: 16
Phiala E. Shanahan, Ryan Abbott, Michael S. Albergo, et al.
Proceedings of The 39th International Symposium on Lattice Field Theory — PoS(LATTICE2022) (2023)
Open Access | Times Cited: 16
Sampling the lattice Nambu-Goto string using Continuous Normalizing Flows
Michele Caselle, Elia Cellini, Alessandro Nada
Journal of High Energy Physics (2024) Vol. 2024, Iss. 2
Open Access | Times Cited: 7
Michele Caselle, Elia Cellini, Alessandro Nada
Journal of High Energy Physics (2024) Vol. 2024, Iss. 2
Open Access | Times Cited: 7
Practical applications of machine-learned flows on gauge fields
Daniel C. Hackett, Ryan Abbott, Denis Boyda, et al.
Proceedings of The 39th International Symposium on Lattice Field Theory — PoS(LATTICE2022) (2024), pp. 011-011
Open Access | Times Cited: 6
Daniel C. Hackett, Ryan Abbott, Denis Boyda, et al.
Proceedings of The 39th International Symposium on Lattice Field Theory — PoS(LATTICE2022) (2024), pp. 011-011
Open Access | Times Cited: 6
Detecting and mitigating mode-collapse for flow-based sampling of lattice field theories
Kim A. Nicoli, Christopher J. Anders, Tobias Hartung, et al.
Physical review. D/Physical review. D. (2023) Vol. 108, Iss. 11
Open Access | Times Cited: 16
Kim A. Nicoli, Christopher J. Anders, Tobias Hartung, et al.
Physical review. D/Physical review. D. (2023) Vol. 108, Iss. 11
Open Access | Times Cited: 16
Flow-based density of states for complex actions
Jan M. Pawlowski, Julian M. Urban
Physical review. D/Physical review. D. (2023) Vol. 108, Iss. 5
Open Access | Times Cited: 15
Jan M. Pawlowski, Julian M. Urban
Physical review. D/Physical review. D. (2023) Vol. 108, Iss. 5
Open Access | Times Cited: 15
Diffusion models as stochastic quantization in lattice field theory
Lili Wang, Gert Aarts, Kai Zhou
Journal of High Energy Physics (2024) Vol. 2024, Iss. 5
Open Access | Times Cited: 5
Lili Wang, Gert Aarts, Kai Zhou
Journal of High Energy Physics (2024) Vol. 2024, Iss. 5
Open Access | Times Cited: 5
Applications of flow models to the generation of correlated lattice QCD ensembles
Ryan Abbott, Aleksandar Botev, Denis Boyda, et al.
Physical review. D/Physical review. D. (2024) Vol. 109, Iss. 9
Open Access | Times Cited: 5
Ryan Abbott, Aleksandar Botev, Denis Boyda, et al.
Physical review. D/Physical review. D. (2024) Vol. 109, Iss. 9
Open Access | Times Cited: 5
Learning trivializing flows
David Albandea, Luigi Del Debbio, Pilar Hernández, et al.
The European Physical Journal C (2023) Vol. 83, Iss. 7
Open Access | Times Cited: 12
David Albandea, Luigi Del Debbio, Pilar Hernández, et al.
The European Physical Journal C (2023) Vol. 83, Iss. 7
Open Access | Times Cited: 12
Gauge covariant neural network for quarks and gluons
Yuki Nagai, Akio Tomiya
Physical review. D/Physical review. D. (2025) Vol. 111, Iss. 7
Open Access
Yuki Nagai, Akio Tomiya
Physical review. D/Physical review. D. (2025) Vol. 111, Iss. 7
Open Access
Lattice scalar field theory at complex coupling
Scott Lawrence, Hyunwoo Oh, Yukari Yamauchi
Physical review. D/Physical review. D. (2022) Vol. 106, Iss. 11
Open Access | Times Cited: 17
Scott Lawrence, Hyunwoo Oh, Yukari Yamauchi
Physical review. D/Physical review. D. (2022) Vol. 106, Iss. 11
Open Access | Times Cited: 17
Exploring QCD matter in extreme conditions with Machine Learning
Kai Zhou, Lingxiao Wang, Long-Gang Pang, et al.
arXiv (Cornell University) (2023)
Open Access | Times Cited: 10
Kai Zhou, Lingxiao Wang, Long-Gang Pang, et al.
arXiv (Cornell University) (2023)
Open Access | Times Cited: 10
Temperature steerable flows and Boltzmann generators
Manuel Dibak, Leon Klein, Andreas Krämer, et al.
Physical Review Research (2022) Vol. 4, Iss. 4
Open Access | Times Cited: 15
Manuel Dibak, Leon Klein, Andreas Krämer, et al.
Physical Review Research (2022) Vol. 4, Iss. 4
Open Access | Times Cited: 15
Training normalizing flows with computationally intensive target probability distributions
P. Białas, Piotr Korcyl, Tomasz Stebel
Computer Physics Communications (2024) Vol. 298, pp. 109094-109094
Open Access | Times Cited: 2
P. Białas, Piotr Korcyl, Tomasz Stebel
Computer Physics Communications (2024) Vol. 298, pp. 109094-109094
Open Access | Times Cited: 2
Self-Learning Monte Carlo with Equivariant Transformer
Yuki Nagai, Akio Tomiya
Journal of the Physical Society of Japan (2024) Vol. 93, Iss. 11
Closed Access | Times Cited: 2
Yuki Nagai, Akio Tomiya
Journal of the Physical Society of Japan (2024) Vol. 93, Iss. 11
Closed Access | Times Cited: 2