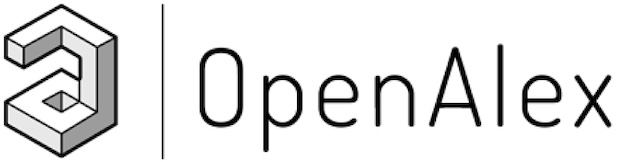
OpenAlex is a bibliographic catalogue of scientific papers, authors and institutions accessible in open access mode, named after the Library of Alexandria. It's citation coverage is excellent and I hope you will find utility in this listing of citing articles!
If you click the article title, you'll navigate to the article, as listed in CrossRef. If you click the Open Access links, you'll navigate to the "best Open Access location". Clicking the citation count will open this listing for that article. Lastly at the bottom of the page, you'll find basic pagination options.
Requested Article:
Strong error analysis for stochastic gradient descent optimization algorithms
Arnulf Jentzen, Benno Kuckuck, Ariel Neufeld, et al.
IMA Journal of Numerical Analysis (2019) Vol. 41, Iss. 1, pp. 455-492
Open Access | Times Cited: 37
Arnulf Jentzen, Benno Kuckuck, Ariel Neufeld, et al.
IMA Journal of Numerical Analysis (2019) Vol. 41, Iss. 1, pp. 455-492
Open Access | Times Cited: 37
Showing 1-25 of 37 citing articles:
Machine Learning Approximation Algorithms for High-Dimensional Fully Nonlinear Partial Differential Equations and Second-order Backward Stochastic Differential Equations
Christian Beck, E Weinan, Arnulf Jentzen
Journal of Nonlinear Science (2019) Vol. 29, Iss. 4, pp. 1563-1619
Open Access | Times Cited: 154
Christian Beck, E Weinan, Arnulf Jentzen
Journal of Nonlinear Science (2019) Vol. 29, Iss. 4, pp. 1563-1619
Open Access | Times Cited: 154
The Modern Mathematics of Deep Learning
Julius Berner, Philipp Grohs, Gitta Kutyniok, et al.
Cambridge University Press eBooks (2022), pp. 1-111
Open Access | Times Cited: 103
Julius Berner, Philipp Grohs, Gitta Kutyniok, et al.
Cambridge University Press eBooks (2022), pp. 1-111
Open Access | Times Cited: 103
Solving stochastic differential equations and Kolmogorov equations by means of deep learning
Christian Beck, S. Becker, Philipp Grohs, et al.
arXiv (Cornell University) (2018)
Closed Access | Times Cited: 101
Christian Beck, S. Becker, Philipp Grohs, et al.
arXiv (Cornell University) (2018)
Closed Access | Times Cited: 101
Deep learning volatility: a deep neural network perspective on pricing and calibration in (rough) volatility models
Blanka Horvath, Aitor Muguruza, Mehdi Tomas
Quantitative Finance (2020) Vol. 21, Iss. 1, pp. 11-27
Open Access | Times Cited: 101
Blanka Horvath, Aitor Muguruza, Mehdi Tomas
Quantitative Finance (2020) Vol. 21, Iss. 1, pp. 11-27
Open Access | Times Cited: 101
Mathematical Aspects of Deep Learning
Philipp Grohs, Philipp Grohs, Julius Berner, et al.
Cambridge University Press eBooks (2022)
Open Access | Times Cited: 49
Philipp Grohs, Philipp Grohs, Julius Berner, et al.
Cambridge University Press eBooks (2022)
Open Access | Times Cited: 49
Full error analysis for the training of deep neural networks
Christian Beck, Arnulf Jentzen, Benno Kuckuck
Infinite Dimensional Analysis Quantum Probability and Related Topics (2022) Vol. 25, Iss. 02
Open Access | Times Cited: 38
Christian Beck, Arnulf Jentzen, Benno Kuckuck
Infinite Dimensional Analysis Quantum Probability and Related Topics (2022) Vol. 25, Iss. 02
Open Access | Times Cited: 38
Stochastic Gradient Descent with Noise of Machine Learning Type Part I: Discrete Time Analysis
Stephan Wojtowytsch
Journal of Nonlinear Science (2023) Vol. 33, Iss. 3
Closed Access | Times Cited: 22
Stephan Wojtowytsch
Journal of Nonlinear Science (2023) Vol. 33, Iss. 3
Closed Access | Times Cited: 22
A Multiscale Feature Extraction Network Based on Channel-Spatial Attention for Electromyographic Signal Classification
Biao Sun, Beida Song, Jiajun Lv, et al.
IEEE Transactions on Cognitive and Developmental Systems (2022) Vol. 15, Iss. 2, pp. 591-601
Closed Access | Times Cited: 22
Biao Sun, Beida Song, Jiajun Lv, et al.
IEEE Transactions on Cognitive and Developmental Systems (2022) Vol. 15, Iss. 2, pp. 591-601
Closed Access | Times Cited: 22
Overall error analysis for the training of deep neural networks via stochastic gradient descent with random initialisation
Arnulf Jentzen, Timo Welti
Applied Mathematics and Computation (2023) Vol. 455, pp. 127907-127907
Open Access | Times Cited: 15
Arnulf Jentzen, Timo Welti
Applied Mathematics and Computation (2023) Vol. 455, pp. 127907-127907
Open Access | Times Cited: 15
Deep Learning Volatility
Blanka Horvath, Aitor Muguruza, Mehdi Tomas
SSRN Electronic Journal (2019)
Open Access | Times Cited: 39
Blanka Horvath, Aitor Muguruza, Mehdi Tomas
SSRN Electronic Journal (2019)
Open Access | Times Cited: 39
Non-convergence of stochastic gradient descent in the training of deep neural networks
Patrick Cheridito, Arnulf Jentzen, Florian Rossmannek
Journal of Complexity (2020) Vol. 64, pp. 101540-101540
Open Access | Times Cited: 35
Patrick Cheridito, Arnulf Jentzen, Florian Rossmannek
Journal of Complexity (2020) Vol. 64, pp. 101540-101540
Open Access | Times Cited: 35
A proof of convergence for gradient descent in the training of artificial neural networks for constant target functions
Patrick Cheridito, Arnulf Jentzen, Adrian Riekert, et al.
Journal of Complexity (2022) Vol. 72, pp. 101646-101646
Open Access | Times Cited: 19
Patrick Cheridito, Arnulf Jentzen, Adrian Riekert, et al.
Journal of Complexity (2022) Vol. 72, pp. 101646-101646
Open Access | Times Cited: 19
An Improved Adagrad Gradient Descent Optimization Algorithm
Nana ZHANG, Deming Lei, Jinghui Zhao
(2018), pp. 2359-2362
Closed Access | Times Cited: 36
Nana ZHANG, Deming Lei, Jinghui Zhao
(2018), pp. 2359-2362
Closed Access | Times Cited: 36
An Efficient Hybrid Model Based on Modified Whale Optimization Algorithm and Multilayer Perceptron Neural Network for Medical Classification Problems
Saeid Raziani, Sajad Ahmadian, Seyed Mohammad Jafar Jalali, et al.
Journal of Bionic Engineering (2022) Vol. 19, Iss. 5, pp. 1504-1521
Closed Access | Times Cited: 16
Saeid Raziani, Sajad Ahmadian, Seyed Mohammad Jafar Jalali, et al.
Journal of Bionic Engineering (2022) Vol. 19, Iss. 5, pp. 1504-1521
Closed Access | Times Cited: 16
Deep Reinforcement Learning for the Agile Earth Observation Satellite Scheduling Problem
Jie Chun, Wenyuan Yang, Xiaolu Liu, et al.
Mathematics (2023) Vol. 11, Iss. 19, pp. 4059-4059
Open Access | Times Cited: 10
Jie Chun, Wenyuan Yang, Xiaolu Liu, et al.
Mathematics (2023) Vol. 11, Iss. 19, pp. 4059-4059
Open Access | Times Cited: 10
Analysis of stochastic gradient descent in continuous time
Jonas Latz
Statistics and Computing (2021) Vol. 31, Iss. 4
Open Access | Times Cited: 23
Jonas Latz
Statistics and Computing (2021) Vol. 31, Iss. 4
Open Access | Times Cited: 23
A Change Detection Method for Remote Sensing Images Based on Coupled Dictionary and Deep Learning
Weiwei Yang, Haifeng Song, Lei Du, et al.
Computational Intelligence and Neuroscience (2022) Vol. 2022, pp. 1-14
Open Access | Times Cited: 15
Weiwei Yang, Haifeng Song, Lei Du, et al.
Computational Intelligence and Neuroscience (2022) Vol. 2022, pp. 1-14
Open Access | Times Cited: 15
The One Step Malliavin scheme: new discretization of BSDEs implemented with deep learning regressions
Bálint Négyesi, Kristoffer Andersson, Cornelis W. Oosterlee
IMA Journal of Numerical Analysis (2024)
Open Access | Times Cited: 2
Bálint Négyesi, Kristoffer Andersson, Cornelis W. Oosterlee
IMA Journal of Numerical Analysis (2024)
Open Access | Times Cited: 2
Lower error bounds for the stochastic gradient descent optimization algorithm: Sharp convergence rates for slowly and fast decaying learning rates
Arnulf Jentzen, Philippe von Wurstemberger
Journal of Complexity (2019) Vol. 57, pp. 101438-101438
Open Access | Times Cited: 20
Arnulf Jentzen, Philippe von Wurstemberger
Journal of Complexity (2019) Vol. 57, pp. 101438-101438
Open Access | Times Cited: 20
Deep Learning Volatility
Blanka Horvath, Aitor Muguruza, Mehdi Tomas
arXiv (Cornell University) (2019)
Closed Access | Times Cited: 20
Blanka Horvath, Aitor Muguruza, Mehdi Tomas
arXiv (Cornell University) (2019)
Closed Access | Times Cited: 20
A proof of convergence for stochastic gradient descent in the training of artificial neural networks with ReLU activation for constant target functions
Arnulf Jentzen, Adrian Riekert
Zeitschrift für angewandte Mathematik und Physik (2022) Vol. 73, Iss. 5
Open Access | Times Cited: 10
Arnulf Jentzen, Adrian Riekert
Zeitschrift für angewandte Mathematik und Physik (2022) Vol. 73, Iss. 5
Open Access | Times Cited: 10
A Global-in-Time Neural Network Approach to Dynamic Portfolio Optimization
Pieter M. van Staden, Peter Forsyth, Yuying Li
Applied Mathematical Finance (2024), pp. 1-33
Open Access | Times Cited: 1
Pieter M. van Staden, Peter Forsyth, Yuying Li
Applied Mathematical Finance (2024), pp. 1-33
Open Access | Times Cited: 1
On the Existence of Global Minima and Convergence Analyses for Gradient Descent Methods in the Training of Deep Neural Networks
Arnulf Jentzen, Adrian Riekert
Journal of Machine Learning (2022) Vol. 1, Iss. 2, pp. 141-246
Open Access | Times Cited: 6
Arnulf Jentzen, Adrian Riekert
Journal of Machine Learning (2022) Vol. 1, Iss. 2, pp. 141-246
Open Access | Times Cited: 6
Uniform-in-Time Weak Error Analysis for Stochastic Gradient Descent Algorithms via Diffusion Approximation
Yuanyuan Feng, Tingran Gao, Lei Li, et al.
arXiv (Cornell University) (2019)
Open Access | Times Cited: 9
Yuanyuan Feng, Tingran Gao, Lei Li, et al.
arXiv (Cornell University) (2019)
Open Access | Times Cited: 9
Convergence rates for the stochastic gradient descent method for non-convex objective functions
Benjamin Fehrman, Benjamin Gess, Arnulf Jentzen
(2019)
Closed Access | Times Cited: 7
Benjamin Fehrman, Benjamin Gess, Arnulf Jentzen
(2019)
Closed Access | Times Cited: 7