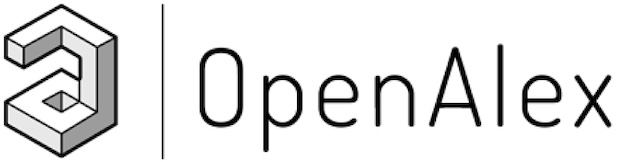
OpenAlex is a bibliographic catalogue of scientific papers, authors and institutions accessible in open access mode, named after the Library of Alexandria. It's citation coverage is excellent and I hope you will find utility in this listing of citing articles!
If you click the article title, you'll navigate to the article, as listed in CrossRef. If you click the Open Access links, you'll navigate to the "best Open Access location". Clicking the citation count will open this listing for that article. Lastly at the bottom of the page, you'll find basic pagination options.
Requested Article:
Current structure predictors are not learning the physics of protein folding
Carlos Outeiral, Daniel A. Nissley, Charlotte M. Deane
Bioinformatics (2022) Vol. 38, Iss. 7, pp. 1881-1887
Open Access | Times Cited: 70
Carlos Outeiral, Daniel A. Nissley, Charlotte M. Deane
Bioinformatics (2022) Vol. 38, Iss. 7, pp. 1881-1887
Open Access | Times Cited: 70
Showing 1-25 of 70 citing articles:
Single-sequence protein structure prediction using a language model and deep learning
Ratul Chowdhury, Nazim Bouatta, Surojit Biswas, et al.
Nature Biotechnology (2022) Vol. 40, Iss. 11, pp. 1617-1623
Open Access | Times Cited: 299
Ratul Chowdhury, Nazim Bouatta, Surojit Biswas, et al.
Nature Biotechnology (2022) Vol. 40, Iss. 11, pp. 1617-1623
Open Access | Times Cited: 299
SPEACH_AF: Sampling protein ensembles and conformational heterogeneity with Alphafold2
Richard A. Stein, Hassane S. Mchaourab
PLoS Computational Biology (2022) Vol. 18, Iss. 8, pp. e1010483-e1010483
Open Access | Times Cited: 155
Richard A. Stein, Hassane S. Mchaourab
PLoS Computational Biology (2022) Vol. 18, Iss. 8, pp. e1010483-e1010483
Open Access | Times Cited: 155
Devlina Chakravarty, Lauren L. Porter
Protein Science (2022) Vol. 31, Iss. 6
Open Access | Times Cited: 134
AlphaFold, Artificial Intelligence (AI), and Allostery
Ruth Nussinov, Mingzhen Zhang, Yonglan Liu, et al.
The Journal of Physical Chemistry B (2022) Vol. 126, Iss. 34, pp. 6372-6383
Open Access | Times Cited: 106
Ruth Nussinov, Mingzhen Zhang, Yonglan Liu, et al.
The Journal of Physical Chemistry B (2022) Vol. 126, Iss. 34, pp. 6372-6383
Open Access | Times Cited: 106
The power and pitfalls of AlphaFold2 for structure prediction beyond rigid globular proteins
Vinayak Agarwal, Andrew C. McShan
Nature Chemical Biology (2024) Vol. 20, Iss. 8, pp. 950-959
Closed Access | Times Cited: 25
Vinayak Agarwal, Andrew C. McShan
Nature Chemical Biology (2024) Vol. 20, Iss. 8, pp. 950-959
Closed Access | Times Cited: 25
AlphaFold predictions of fold-switched conformations are driven by structure memorization
Devlina Chakravarty, Joseph W. Schafer, Ethan A. Chen, et al.
Nature Communications (2024) Vol. 15, Iss. 1
Open Access | Times Cited: 25
Devlina Chakravarty, Joseph W. Schafer, Ethan A. Chen, et al.
Nature Communications (2024) Vol. 15, Iss. 1
Open Access | Times Cited: 25
Codon language embeddings provide strong signals for use in protein engineering
Carlos Outeiral, Charlotte M. Deane
Nature Machine Intelligence (2024) Vol. 6, Iss. 2, pp. 170-179
Open Access | Times Cited: 24
Carlos Outeiral, Charlotte M. Deane
Nature Machine Intelligence (2024) Vol. 6, Iss. 2, pp. 170-179
Open Access | Times Cited: 24
Machine-designed biotherapeutics: opportunities, feasibility and advantages of deep learning in computational antibody discovery
Wiktoria Wilman, Sonia Wróbel, Weronika Bielska, et al.
Briefings in Bioinformatics (2022) Vol. 23, Iss. 4
Open Access | Times Cited: 59
Wiktoria Wilman, Sonia Wróbel, Weronika Bielska, et al.
Briefings in Bioinformatics (2022) Vol. 23, Iss. 4
Open Access | Times Cited: 59
Protein structure and folding pathway prediction based on remote homologs recognition using PAthreader
Kailong Zhao, Yuhao Xia, Fujin Zhang, et al.
Communications Biology (2023) Vol. 6, Iss. 1
Open Access | Times Cited: 30
Kailong Zhao, Yuhao Xia, Fujin Zhang, et al.
Communications Biology (2023) Vol. 6, Iss. 1
Open Access | Times Cited: 30
Evolutionary selection of proteins with two folds
Joseph W. Schafer, Lauren L. Porter
Nature Communications (2023) Vol. 14, Iss. 1
Open Access | Times Cited: 30
Joseph W. Schafer, Lauren L. Porter
Nature Communications (2023) Vol. 14, Iss. 1
Open Access | Times Cited: 30
Generative artificial intelligence for de novo protein design
Adam Winnifrith, Carlos Outeiral, Brian Hie
Current Opinion in Structural Biology (2024) Vol. 86, pp. 102794-102794
Open Access | Times Cited: 14
Adam Winnifrith, Carlos Outeiral, Brian Hie
Current Opinion in Structural Biology (2024) Vol. 86, pp. 102794-102794
Open Access | Times Cited: 14
Deep learning for protein structure prediction and design—progress and applications
Jürgen Jänes, Pedro Beltrão
Molecular Systems Biology (2024) Vol. 20, Iss. 3, pp. 162-169
Open Access | Times Cited: 13
Jürgen Jänes, Pedro Beltrão
Molecular Systems Biology (2024) Vol. 20, Iss. 3, pp. 162-169
Open Access | Times Cited: 13
A Perspective on Protein Structure Prediction Using Quantum Computers
Hakan Doğa, Bryan Raubenolt, Fabio Cumbo, et al.
Journal of Chemical Theory and Computation (2024) Vol. 20, Iss. 9, pp. 3359-3378
Open Access | Times Cited: 10
Hakan Doğa, Bryan Raubenolt, Fabio Cumbo, et al.
Journal of Chemical Theory and Computation (2024) Vol. 20, Iss. 9, pp. 3359-3378
Open Access | Times Cited: 10
Membranome 3.0: Database of single‐pass membrane proteins with AlphaFold models
Andrei L. Lomize, Kevin A. Schnitzer, Spencer C. Todd, et al.
Protein Science (2022) Vol. 31, Iss. 5
Open Access | Times Cited: 29
Andrei L. Lomize, Kevin A. Schnitzer, Spencer C. Todd, et al.
Protein Science (2022) Vol. 31, Iss. 5
Open Access | Times Cited: 29
AlphaFold2 has more to learn about protein energy landscapes
Devlina Chakravarty, Joseph W. Schafer, Ethan A. Chen, et al.
bioRxiv (Cold Spring Harbor Laboratory) (2023)
Open Access | Times Cited: 19
Devlina Chakravarty, Joseph W. Schafer, Ethan A. Chen, et al.
bioRxiv (Cold Spring Harbor Laboratory) (2023)
Open Access | Times Cited: 19
Distinguishing features of fold‐switching proteins
Devlina Chakravarty, Joseph W. Schafer, Lauren L. Porter
Protein Science (2023) Vol. 32, Iss. 3
Open Access | Times Cited: 16
Devlina Chakravarty, Joseph W. Schafer, Lauren L. Porter
Protein Science (2023) Vol. 32, Iss. 3
Open Access | Times Cited: 16
Protein structure prediction in the era of AI: Challenges and limitations when applying to in silico force spectroscopy
Priscila da Silva Figueiredo Celestino Gomes, Diego E. B. Gomes, Rafael C. Bernardi
Frontiers in Bioinformatics (2022) Vol. 2
Open Access | Times Cited: 24
Priscila da Silva Figueiredo Celestino Gomes, Diego E. B. Gomes, Rafael C. Bernardi
Frontiers in Bioinformatics (2022) Vol. 2
Open Access | Times Cited: 24
Understanding and controlling the molecular mechanisms of protein aggregation in mAb therapeutics
Kuin Tian Pang, Yuansheng Yang, Wei Zhang, et al.
Biotechnology Advances (2023) Vol. 67, pp. 108192-108192
Open Access | Times Cited: 14
Kuin Tian Pang, Yuansheng Yang, Wei Zhang, et al.
Biotechnology Advances (2023) Vol. 67, pp. 108192-108192
Open Access | Times Cited: 14
AlphaFold and what is next: bridging functional, systems and structural biology
Kacper Szczepski, Łukasz Jaremko
Expert Review of Proteomics (2025)
Closed Access
Kacper Szczepski, Łukasz Jaremko
Expert Review of Proteomics (2025)
Closed Access
Advances in bioinformatics and multi-omics integration: transforming viral infectious disease research in veterinary medicine
Alyaa Elrashedy, Walid Mousa, Mohamed Nayel, et al.
Virology Journal (2025) Vol. 22, Iss. 1
Open Access
Alyaa Elrashedy, Walid Mousa, Mohamed Nayel, et al.
Virology Journal (2025) Vol. 22, Iss. 1
Open Access
Protein structure prediction in the deep learning era
Zhenling Peng, Wenkai Wang, Renmin Han, et al.
Current Opinion in Structural Biology (2022) Vol. 77, pp. 102495-102495
Closed Access | Times Cited: 21
Zhenling Peng, Wenkai Wang, Renmin Han, et al.
Current Opinion in Structural Biology (2022) Vol. 77, pp. 102495-102495
Closed Access | Times Cited: 21
Integrating comparative modeling and accelerated simulations reveals conformational and energetic basis of actomyosin force generation
Wen Ma, Shengjun You, Michael Regnier, et al.
Proceedings of the National Academy of Sciences (2023) Vol. 120, Iss. 9
Open Access | Times Cited: 11
Wen Ma, Shengjun You, Michael Regnier, et al.
Proceedings of the National Academy of Sciences (2023) Vol. 120, Iss. 9
Open Access | Times Cited: 11
Accurate prediction of protein folding mechanisms by simple structure-based statistical mechanical models
Koji Ooka, Munehito Arai
Nature Communications (2023) Vol. 14, Iss. 1
Open Access | Times Cited: 11
Koji Ooka, Munehito Arai
Nature Communications (2023) Vol. 14, Iss. 1
Open Access | Times Cited: 11
Natural Language Processing Methods for the Study of Protein–Ligand Interactions
J.H. Michels, Ramya Bandarupalli, Ahmad Akbari, et al.
Journal of Chemical Information and Modeling (2025)
Open Access
J.H. Michels, Ramya Bandarupalli, Ahmad Akbari, et al.
Journal of Chemical Information and Modeling (2025)
Open Access
The Physics-AI Dialogue in Drug Design
Pablo Andrés Vargas-Rosales, Amedeo Caflisch
RSC Medicinal Chemistry (2025)
Open Access
Pablo Andrés Vargas-Rosales, Amedeo Caflisch
RSC Medicinal Chemistry (2025)
Open Access