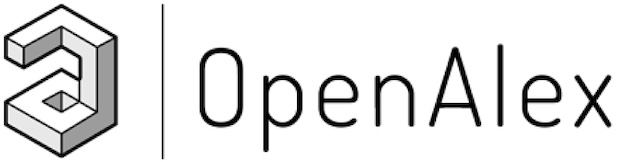
OpenAlex is a bibliographic catalogue of scientific papers, authors and institutions accessible in open access mode, named after the Library of Alexandria. It's citation coverage is excellent and I hope you will find utility in this listing of citing articles!
If you click the article title, you'll navigate to the article, as listed in CrossRef. If you click the Open Access links, you'll navigate to the "best Open Access location". Clicking the citation count will open this listing for that article. Lastly at the bottom of the page, you'll find basic pagination options.
Requested Article:
Computational analysis and prediction of lysine malonylation sites by exploiting informative features in an integrative machine-learning framework
Yanju Zhang, Ruopeng Xie, Jiawei Wang, et al.
Briefings in Bioinformatics (2018) Vol. 20, Iss. 6, pp. 2185-2199
Open Access | Times Cited: 98
Yanju Zhang, Ruopeng Xie, Jiawei Wang, et al.
Briefings in Bioinformatics (2018) Vol. 20, Iss. 6, pp. 2185-2199
Open Access | Times Cited: 98
Showing 1-25 of 98 citing articles:
Machine Learning in Enzyme Engineering
Stanislav Mazurenko, Zbyněk Prokop, Jir̆ı́ Damborský
ACS Catalysis (2019) Vol. 10, Iss. 2, pp. 1210-1223
Open Access | Times Cited: 340
Stanislav Mazurenko, Zbyněk Prokop, Jir̆ı́ Damborský
ACS Catalysis (2019) Vol. 10, Iss. 2, pp. 1210-1223
Open Access | Times Cited: 340
mAHTPred: a sequence-based meta-predictor for improving the prediction of anti-hypertensive peptides using effective feature representation
Balachandran Manavalan, Shaherin Basith, Tae Hwan Shin, et al.
Bioinformatics (2018) Vol. 35, Iss. 16, pp. 2757-2765
Closed Access | Times Cited: 223
Balachandran Manavalan, Shaherin Basith, Tae Hwan Shin, et al.
Bioinformatics (2018) Vol. 35, Iss. 16, pp. 2757-2765
Closed Access | Times Cited: 223
iRNA(m6A)-PseDNC: Identifying N6-methyladenosine sites using pseudo dinucleotide composition
Wei Chen, Hui Ding, Xu Zhou, et al.
Analytical Biochemistry (2018) Vol. 561-562, pp. 59-65
Closed Access | Times Cited: 186
Wei Chen, Hui Ding, Xu Zhou, et al.
Analytical Biochemistry (2018) Vol. 561-562, pp. 59-65
Closed Access | Times Cited: 186
SDM6A: A Web-Based Integrative Machine-Learning Framework for Predicting 6mA Sites in the Rice Genome
Shaherin Basith, Balachandran Manavalan, Tae Hwan Shin, et al.
Molecular Therapy — Nucleic Acids (2019) Vol. 18, pp. 131-141
Open Access | Times Cited: 151
Shaherin Basith, Balachandran Manavalan, Tae Hwan Shin, et al.
Molecular Therapy — Nucleic Acids (2019) Vol. 18, pp. 131-141
Open Access | Times Cited: 151
Development of machine learning model for diagnostic disease prediction based on laboratory tests
Dong Jin Park, Min Woo Park, Homin Lee, et al.
Scientific Reports (2021) Vol. 11, Iss. 1
Open Access | Times Cited: 131
Dong Jin Park, Min Woo Park, Homin Lee, et al.
Scientific Reports (2021) Vol. 11, Iss. 1
Open Access | Times Cited: 131
SPalmitoylC-PseAAC: A sequence-based model developed via Chou's 5-steps rule and general PseAAC for identifying S-palmitoylation sites in proteins
Waqar Hussain, Yaser Daanial Khan, Nouman Rasool, et al.
Analytical Biochemistry (2018) Vol. 568, pp. 14-23
Closed Access | Times Cited: 122
Waqar Hussain, Yaser Daanial Khan, Nouman Rasool, et al.
Analytical Biochemistry (2018) Vol. 568, pp. 14-23
Closed Access | Times Cited: 122
Meta-i6mA: an interspecies predictor for identifying DNAN6-methyladenine sites of plant genomes by exploiting informative features in an integrative machine-learning framework
Md Mehedi Hasan, Shaherin Basith, Mst. Shamima Khatun, et al.
Briefings in Bioinformatics (2020) Vol. 22, Iss. 3
Closed Access | Times Cited: 113
Md Mehedi Hasan, Shaherin Basith, Mst. Shamima Khatun, et al.
Briefings in Bioinformatics (2020) Vol. 22, Iss. 3
Closed Access | Times Cited: 113
Deep-Kcr: accurate detection of lysine crotonylation sites using deep learning method
Hao Lv, Fanny Dao, Zheng-Xing Guan, et al.
Briefings in Bioinformatics (2020) Vol. 22, Iss. 4
Closed Access | Times Cited: 112
Hao Lv, Fanny Dao, Zheng-Xing Guan, et al.
Briefings in Bioinformatics (2020) Vol. 22, Iss. 4
Closed Access | Times Cited: 112
iRNA-PseKNC(2methyl): Identify RNA 2'-O-methylation sites by convolution neural network and Chou's pseudo components
Muhammad Tahir, Hilal Tayara, Kil To Chong
Journal of Theoretical Biology (2018) Vol. 465, pp. 1-6
Open Access | Times Cited: 101
Muhammad Tahir, Hilal Tayara, Kil To Chong
Journal of Theoretical Biology (2018) Vol. 465, pp. 1-6
Open Access | Times Cited: 101
iN6-Methyl (5-step): Identifying RNA N6-methyladenosine sites using deep learning mode via Chou's 5-step rules and Chou's general PseKNC
Iman Nazari, Muhammad Tahir, Hilal Tayara, et al.
Chemometrics and Intelligent Laboratory Systems (2019) Vol. 193, pp. 103811-103811
Closed Access | Times Cited: 97
Iman Nazari, Muhammad Tahir, Hilal Tayara, et al.
Chemometrics and Intelligent Laboratory Systems (2019) Vol. 193, pp. 103811-103811
Closed Access | Times Cited: 97
iPhosH-PseAAC: Identify Phosphohistidine Sites in Proteins by Blending Statistical Moments and Position Relative Features According to the Chou's 5-Step Rule and General Pseudo Amino Acid Composition
Muhammad Awais, Waqar Hussain, Yaser Daanial Khan, et al.
IEEE/ACM Transactions on Computational Biology and Bioinformatics (2019) Vol. 18, Iss. 2, pp. 596-610
Closed Access | Times Cited: 95
Muhammad Awais, Waqar Hussain, Yaser Daanial Khan, et al.
IEEE/ACM Transactions on Computational Biology and Bioinformatics (2019) Vol. 18, Iss. 2, pp. 596-610
Closed Access | Times Cited: 95
iDNA6mA (5-step rule): Identification of DNA N6-methyladenine sites in the rice genome by intelligent computational model via Chou's 5-step rule
Muhammad Tahir, Hilal Tayara, Kil To Chong
Chemometrics and Intelligent Laboratory Systems (2019) Vol. 189, pp. 96-101
Closed Access | Times Cited: 91
Muhammad Tahir, Hilal Tayara, Kil To Chong
Chemometrics and Intelligent Laboratory Systems (2019) Vol. 189, pp. 96-101
Closed Access | Times Cited: 91
iPSW(2L)-PseKNC: A two-layer predictor for identifying promoters and their strength by hybrid features via pseudo K-tuple nucleotide composition
Xuan Xiao, Zhaochun Xu, Wang‐Ren Qiu, et al.
Genomics (2018) Vol. 111, Iss. 6, pp. 1785-1793
Open Access | Times Cited: 87
Xuan Xiao, Zhaochun Xu, Wang‐Ren Qiu, et al.
Genomics (2018) Vol. 111, Iss. 6, pp. 1785-1793
Open Access | Times Cited: 87
INTEDE: interactome of drug-metabolizing enzymes
Jiayi Yin, Fengcheng Li, Ying Zhou, et al.
Nucleic Acids Research (2020) Vol. 49, Iss. D1, pp. D1233-D1243
Open Access | Times Cited: 87
Jiayi Yin, Fengcheng Li, Ying Zhou, et al.
Nucleic Acids Research (2020) Vol. 49, Iss. D1, pp. D1233-D1243
Open Access | Times Cited: 87
Bastion3: a two-layer ensemble predictor of type III secreted effectors
Jiawei Wang, Jiahui Li, Bingjiao Yang, et al.
Bioinformatics (2018) Vol. 35, Iss. 12, pp. 2017-2028
Open Access | Times Cited: 86
Jiawei Wang, Jiahui Li, Bingjiao Yang, et al.
Bioinformatics (2018) Vol. 35, Iss. 12, pp. 2017-2028
Open Access | Times Cited: 86
Progresses in Predicting Post-translational Modification
Kuo‐Chen Chou
International Journal of Peptide Research and Therapeutics (2019) Vol. 26, Iss. 2, pp. 873-888
Closed Access | Times Cited: 82
Kuo‐Chen Chou
International Journal of Peptide Research and Therapeutics (2019) Vol. 26, Iss. 2, pp. 873-888
Closed Access | Times Cited: 82
Prediction of bio-sequence modifications and the associations with diseases
Chunyan Ao, Liang Yu, Quan Zou
Briefings in Functional Genomics (2020) Vol. 20, Iss. 1, pp. 1-18
Closed Access | Times Cited: 72
Chunyan Ao, Liang Yu, Quan Zou
Briefings in Functional Genomics (2020) Vol. 20, Iss. 1, pp. 1-18
Closed Access | Times Cited: 72
STALLION: a stacking-based ensemble learning framework for prokaryotic lysine acetylation site prediction
Shaherin Basith, Gwang Lee, Balachandran Manavalan
Briefings in Bioinformatics (2021) Vol. 23, Iss. 1
Open Access | Times Cited: 72
Shaherin Basith, Gwang Lee, Balachandran Manavalan
Briefings in Bioinformatics (2021) Vol. 23, Iss. 1
Open Access | Times Cited: 72
Beyond metabolic waste: lysine lactylation and its potential roles in cancer progression and cell fate determination
Jun‐Han Wang, Ling Mao, Jun Wang, et al.
Cellular Oncology (2023) Vol. 46, Iss. 3, pp. 465-480
Closed Access | Times Cited: 34
Jun‐Han Wang, Ling Mao, Jun Wang, et al.
Cellular Oncology (2023) Vol. 46, Iss. 3, pp. 465-480
Closed Access | Times Cited: 34
Systematic analysis and prediction of type IV secreted effector proteins by machine learning approaches
Jiawei Wang, Bingjiao Yang, Yi An, et al.
Briefings in Bioinformatics (2017) Vol. 20, Iss. 3, pp. 931-951
Open Access | Times Cited: 72
Jiawei Wang, Bingjiao Yang, Yi An, et al.
Briefings in Bioinformatics (2017) Vol. 20, Iss. 3, pp. 931-951
Open Access | Times Cited: 72
iSulfoTyr-PseAAC: Identify Tyrosine Sulfation Sites by Incorporating Statistical Moments via Chou’s 5-steps Rule and Pseudo Components
Omar Barukab, Yaser Daanial Khan, Sher Afzal Khan, et al.
Current Genomics (2019) Vol. 20, Iss. 4, pp. 306-320
Open Access | Times Cited: 65
Omar Barukab, Yaser Daanial Khan, Sher Afzal Khan, et al.
Current Genomics (2019) Vol. 20, Iss. 4, pp. 306-320
Open Access | Times Cited: 65
iMethylK-PseAAC: Improving Accuracy of Lysine Methylation Sites Identification by Incorporating Statistical Moments and Position Relative Features into General PseAAC via Chou’s 5-steps Rule
Sarah Ilyas, Waqar Hussain, Adeel Ashraf, et al.
Current Genomics (2019) Vol. 20, Iss. 4, pp. 275-292
Open Access | Times Cited: 64
Sarah Ilyas, Waqar Hussain, Adeel Ashraf, et al.
Current Genomics (2019) Vol. 20, Iss. 4, pp. 275-292
Open Access | Times Cited: 64
4mCCNN: Identification of N4-Methylcytosine Sites in Prokaryotes Using Convolutional Neural Network
Jhabindra Khanal, Iman Nazari, Hilal Tayara, et al.
IEEE Access (2019) Vol. 7, pp. 145455-145461
Open Access | Times Cited: 63
Jhabindra Khanal, Iman Nazari, Hilal Tayara, et al.
IEEE Access (2019) Vol. 7, pp. 145455-145461
Open Access | Times Cited: 63
A comprehensive comparison and analysis of computational predictors for RNA N6-methyladenosine sites of Saccharomyces cerevisiae
Xiaolei Zhu, Jingjing He, Shihao Zhao, et al.
Briefings in Functional Genomics (2019)
Closed Access | Times Cited: 60
Xiaolei Zhu, Jingjing He, Shihao Zhao, et al.
Briefings in Functional Genomics (2019)
Closed Access | Times Cited: 60
DeepVF: a deep learning-based hybrid framework for identifying virulence factors using the stacking strategy
Ruopeng Xie, Jiahui Li, Jiawei Wang, et al.
Briefings in Bioinformatics (2020) Vol. 22, Iss. 3
Open Access | Times Cited: 59
Ruopeng Xie, Jiahui Li, Jiawei Wang, et al.
Briefings in Bioinformatics (2020) Vol. 22, Iss. 3
Open Access | Times Cited: 59