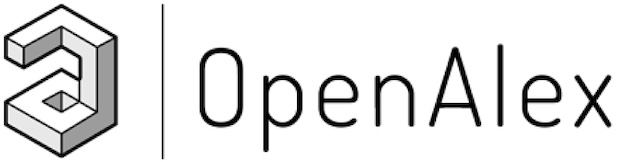
OpenAlex is a bibliographic catalogue of scientific papers, authors and institutions accessible in open access mode, named after the Library of Alexandria. It's citation coverage is excellent and I hope you will find utility in this listing of citing articles!
If you click the article title, you'll navigate to the article, as listed in CrossRef. If you click the Open Access links, you'll navigate to the "best Open Access location". Clicking the citation count will open this listing for that article. Lastly at the bottom of the page, you'll find basic pagination options.
Requested Article:
Reducing autocorrelation times in lattice simulations with generative adversarial networks
Jan M. Pawlowski, Julian M. Urban
Machine Learning Science and Technology (2020) Vol. 1, Iss. 4, pp. 045011-045011
Open Access | Times Cited: 62
Jan M. Pawlowski, Julian M. Urban
Machine Learning Science and Technology (2020) Vol. 1, Iss. 4, pp. 045011-045011
Open Access | Times Cited: 62
Showing 1-25 of 62 citing articles:
Anomaly detection with density estimation
Benjamin Nachman, David Shih
Physical review. D/Physical review. D. (2020) Vol. 101, Iss. 7
Open Access | Times Cited: 261
Benjamin Nachman, David Shih
Physical review. D/Physical review. D. (2020) Vol. 101, Iss. 7
Open Access | Times Cited: 261
Machine learning for quantum matter
Juan Carrasquilla
Advances in Physics X (2020) Vol. 5, Iss. 1, pp. 1797528-1797528
Open Access | Times Cited: 202
Juan Carrasquilla
Advances in Physics X (2020) Vol. 5, Iss. 1, pp. 1797528-1797528
Open Access | Times Cited: 202
Colloquium: Machine learning in nuclear physics
A. Boehnlein, Markus Diefenthaler, N. Sato, et al.
Reviews of Modern Physics (2022) Vol. 94, Iss. 3
Open Access | Times Cited: 143
A. Boehnlein, Markus Diefenthaler, N. Sato, et al.
Reviews of Modern Physics (2022) Vol. 94, Iss. 3
Open Access | Times Cited: 143
Fast and accurate simulations of calorimeter showers with normalizing flows
Claudius Krause, David Shih
Physical review. D/Physical review. D. (2023) Vol. 107, Iss. 11
Open Access | Times Cited: 69
Claudius Krause, David Shih
Physical review. D/Physical review. D. (2023) Vol. 107, Iss. 11
Open Access | Times Cited: 69
High-energy nuclear physics meets machine learning
W. He, Y. G., Long-Gang Pang, et al.
Nuclear Science and Techniques (2023) Vol. 34, Iss. 6
Open Access | Times Cited: 61
W. He, Y. G., Long-Gang Pang, et al.
Nuclear Science and Techniques (2023) Vol. 34, Iss. 6
Open Access | Times Cited: 61
Sampling usingSU ( N ) gauge equivariant flows
Denis Boyda, Gurtej Kanwar, Sébastien Racanière, et al.
Physical review. D/Physical review. D. (2021) Vol. 103, Iss. 7
Open Access | Times Cited: 102
Denis Boyda, Gurtej Kanwar, Sébastien Racanière, et al.
Physical review. D/Physical review. D. (2021) Vol. 103, Iss. 7
Open Access | Times Cited: 102
Estimation of Thermodynamic Observables in Lattice Field Theories with Deep Generative Models
Kim A. Nicoli, Christopher J. Anders, Lena Funcke, et al.
Physical Review Letters (2021) Vol. 126, Iss. 3
Open Access | Times Cited: 101
Kim A. Nicoli, Christopher J. Anders, Lena Funcke, et al.
Physical Review Letters (2021) Vol. 126, Iss. 3
Open Access | Times Cited: 101
Lattice Gauge Equivariant Convolutional Neural Networks
Matteo Favoni, Andreas Ipp, David I. Müller, et al.
Physical Review Letters (2022) Vol. 128, Iss. 3
Open Access | Times Cited: 48
Matteo Favoni, Andreas Ipp, David I. Müller, et al.
Physical Review Letters (2022) Vol. 128, Iss. 3
Open Access | Times Cited: 48
Gauge-equivariant flow models for sampling in lattice field theories with pseudofermions
Ryan Abbott, Michael S. Albergo, Denis Boyda, et al.
Physical review. D/Physical review. D. (2022) Vol. 106, Iss. 7
Open Access | Times Cited: 39
Ryan Abbott, Michael S. Albergo, Denis Boyda, et al.
Physical review. D/Physical review. D. (2022) Vol. 106, Iss. 7
Open Access | Times Cited: 39
Exploring QCD matter in extreme conditions with Machine Learning
Kai Zhou, Lingxiao Wang, Long-Gang Pang, et al.
Progress in Particle and Nuclear Physics (2023) Vol. 135, pp. 104084-104084
Open Access | Times Cited: 37
Kai Zhou, Lingxiao Wang, Long-Gang Pang, et al.
Progress in Particle and Nuclear Physics (2023) Vol. 135, pp. 104084-104084
Open Access | Times Cited: 37
Learning lattice quantum field theories with equivariant continuous flows
Mathis Gerdes, Pim de Haan, Corrado Rainone, et al.
SciPost Physics (2023) Vol. 15, Iss. 6
Open Access | Times Cited: 24
Mathis Gerdes, Pim de Haan, Corrado Rainone, et al.
SciPost Physics (2023) Vol. 15, Iss. 6
Open Access | Times Cited: 24
Conditional normalizing flow for Markov chain Monte Carlo sampling in the critical region of lattice field theory
Ankur Singha, Dipankar Chakrabarti, Vipul Arora
Physical review. D/Physical review. D. (2023) Vol. 107, Iss. 1
Open Access | Times Cited: 23
Ankur Singha, Dipankar Chakrabarti, Vipul Arora
Physical review. D/Physical review. D. (2023) Vol. 107, Iss. 1
Open Access | Times Cited: 23
Aspects of scaling and scalability for flow-based sampling of lattice QCD
Ryan Abbott, Michael S. Albergo, Aleksandar Botev, et al.
The European Physical Journal A (2023) Vol. 59, Iss. 11
Open Access | Times Cited: 23
Ryan Abbott, Michael S. Albergo, Aleksandar Botev, et al.
The European Physical Journal A (2023) Vol. 59, Iss. 11
Open Access | Times Cited: 23
Nuclear matrix elements from lattice QCD for electroweak and beyond-Standard-Model processes
Zohreh Davoudi, William Detmold, Phiala E. Shanahan, et al.
Physics Reports (2020) Vol. 900, pp. 1-74
Open Access | Times Cited: 69
Zohreh Davoudi, William Detmold, Phiala E. Shanahan, et al.
Physics Reports (2020) Vol. 900, pp. 1-74
Open Access | Times Cited: 69
Spectral reconstruction with deep neural networks
Lukas Kades, Jan M. Pawlowski, Alexander Rothkopf, et al.
Physical review. D/Physical review. D. (2020) Vol. 102, Iss. 9
Open Access | Times Cited: 57
Lukas Kades, Jan M. Pawlowski, Alexander Rothkopf, et al.
Physical review. D/Physical review. D. (2020) Vol. 102, Iss. 9
Open Access | Times Cited: 57
Neural resampler for Monte Carlo reweighting with preserved uncertainties
Benjamin Nachman, Jesse Thaler
Physical review. D/Physical review. D. (2020) Vol. 102, Iss. 7
Open Access | Times Cited: 50
Benjamin Nachman, Jesse Thaler
Physical review. D/Physical review. D. (2020) Vol. 102, Iss. 7
Open Access | Times Cited: 50
Flow-based sampling for fermionic lattice field theories
Michael S. Albergo, Gurtej Kanwar, Sébastien Racanière, et al.
Physical review. D/Physical review. D. (2021) Vol. 104, Iss. 11
Open Access | Times Cited: 49
Michael S. Albergo, Gurtej Kanwar, Sébastien Racanière, et al.
Physical review. D/Physical review. D. (2021) Vol. 104, Iss. 11
Open Access | Times Cited: 49
Efficient modeling of trivializing maps for lattice ϕ 4 theory using normalizing flows: A first look at scalability
Luigi Del Debbio, Joe Marsh Rossney, Michael G. Wilson
Physical review. D/Physical review. D. (2021) Vol. 104, Iss. 9
Open Access | Times Cited: 43
Luigi Del Debbio, Joe Marsh Rossney, Michael G. Wilson
Physical review. D/Physical review. D. (2021) Vol. 104, Iss. 9
Open Access | Times Cited: 43
Stochastic normalizing flows as non-equilibrium transformations
Michele Caselle, Elia Cellini, Alessandro Nada, et al.
Journal of High Energy Physics (2022) Vol. 2022, Iss. 7
Open Access | Times Cited: 33
Michele Caselle, Elia Cellini, Alessandro Nada, et al.
Journal of High Energy Physics (2022) Vol. 2022, Iss. 7
Open Access | Times Cited: 33
Advances in machine-learning-based sampling motivated by lattice quantum chromodynamics
K. Cranmer, Gurtej Kanwar, Sébastien Racanière, et al.
Nature Reviews Physics (2023) Vol. 5, Iss. 9, pp. 526-535
Open Access | Times Cited: 19
K. Cranmer, Gurtej Kanwar, Sébastien Racanière, et al.
Nature Reviews Physics (2023) Vol. 5, Iss. 9, pp. 526-535
Open Access | Times Cited: 19
Sampling QCD field configurations with gauge-equivariant flow models
Phiala E. Shanahan, Ryan Abbott, Michael S. Albergo, et al.
Proceedings of The 39th International Symposium on Lattice Field Theory — PoS(LATTICE2022) (2023)
Open Access | Times Cited: 16
Phiala E. Shanahan, Ryan Abbott, Michael S. Albergo, et al.
Proceedings of The 39th International Symposium on Lattice Field Theory — PoS(LATTICE2022) (2023)
Open Access | Times Cited: 16
Finding the deconfinement temperature in lattice Yang-Mills theories from outside the scaling window with machine learning
Denis Boyda, M. N. Chernodub, N. V. Gerasimeniuk, et al.
Physical review. D/Physical review. D. (2021) Vol. 103, Iss. 1
Open Access | Times Cited: 34
Denis Boyda, M. N. Chernodub, N. V. Gerasimeniuk, et al.
Physical review. D/Physical review. D. (2021) Vol. 103, Iss. 1
Open Access | Times Cited: 34
Fourier-flow model generating Feynman paths
Shile Chen, Oleh Savchuk, Shiqi Zheng, et al.
Physical review. D/Physical review. D. (2023) Vol. 107, Iss. 5
Open Access | Times Cited: 14
Shile Chen, Oleh Savchuk, Shiqi Zheng, et al.
Physical review. D/Physical review. D. (2023) Vol. 107, Iss. 5
Open Access | Times Cited: 14
Self-learning Monte Carlo for non-Abelian gauge theory with dynamical fermions
Yuki Nagai, Akinori Tanaka, Akio Tomiya
Physical review. D/Physical review. D. (2023) Vol. 107, Iss. 5
Open Access | Times Cited: 13
Yuki Nagai, Akinori Tanaka, Akio Tomiya
Physical review. D/Physical review. D. (2023) Vol. 107, Iss. 5
Open Access | Times Cited: 13
Diffusion models as stochastic quantization in lattice field theory
Lili Wang, Gert Aarts, Kai Zhou
Journal of High Energy Physics (2024) Vol. 2024, Iss. 5
Open Access | Times Cited: 5
Lili Wang, Gert Aarts, Kai Zhou
Journal of High Energy Physics (2024) Vol. 2024, Iss. 5
Open Access | Times Cited: 5