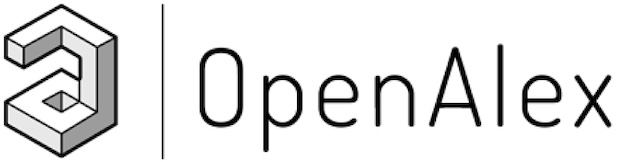
OpenAlex is a bibliographic catalogue of scientific papers, authors and institutions accessible in open access mode, named after the Library of Alexandria. It's citation coverage is excellent and I hope you will find utility in this listing of citing articles!
If you click the article title, you'll navigate to the article, as listed in CrossRef. If you click the Open Access links, you'll navigate to the "best Open Access location". Clicking the citation count will open this listing for that article. Lastly at the bottom of the page, you'll find basic pagination options.
Requested Article:
Clinical assessment of deep learning-based uncertainty maps in lung cancer segmentation
Federica Carmen Maruccio, Wietse S.C. Eppinga, Max-Heinrich Laves, et al.
Physics in Medicine and Biology (2024) Vol. 69, Iss. 3, pp. 035007-035007
Open Access | Times Cited: 8
Federica Carmen Maruccio, Wietse S.C. Eppinga, Max-Heinrich Laves, et al.
Physics in Medicine and Biology (2024) Vol. 69, Iss. 3, pp. 035007-035007
Open Access | Times Cited: 8
Showing 8 citing articles:
Explainability and uncertainty: Two sides of the same coin for enhancing the interpretability of deep learning models in healthcare
Massimo Salvi, Silvia Seoni, Andrea Campagner, et al.
International Journal of Medical Informatics (2025) Vol. 197, pp. 105846-105846
Open Access
Massimo Salvi, Silvia Seoni, Andrea Campagner, et al.
International Journal of Medical Informatics (2025) Vol. 197, pp. 105846-105846
Open Access
Histogram matching‐enhanced adversarial learning for unsupervised domain adaptation in medical image segmentation
Xiaoxue Qian, Hua‐Chieh Shao, Yunxiang Li, et al.
Medical Physics (2025)
Open Access
Xiaoxue Qian, Hua‐Chieh Shao, Yunxiang Li, et al.
Medical Physics (2025)
Open Access
Uncertainty-Aware Deep Learning for Segmentation of Primary Tumor and Pathologic Lymph Nodes in Oropharyngeal Cancer: Insights from a Multi-Center Cohort
Alessia de Biase, Nanna M. Sijtsema, Lisanne V. van Dijk, et al.
Computerized Medical Imaging and Graphics (2025), pp. 102535-102535
Open Access
Alessia de Biase, Nanna M. Sijtsema, Lisanne V. van Dijk, et al.
Computerized Medical Imaging and Graphics (2025), pp. 102535-102535
Open Access
A dynamic uncertainty-aware ensemble model: Application to lung cancer segmentation in digital pathology
Massimo Salvi, Alessandro Mogetta, U. Raghavendra, et al.
Applied Soft Computing (2024) Vol. 165, pp. 112081-112081
Open Access | Times Cited: 4
Massimo Salvi, Alessandro Mogetta, U. Raghavendra, et al.
Applied Soft Computing (2024) Vol. 165, pp. 112081-112081
Open Access | Times Cited: 4
Clinical adoption of deep learning target auto-segmentation for radiation therapy: challenges, clinical risks, and mitigation strategies
Alessia de Biase, Nanna M. Sijtsema, Tomas Janssen, et al.
Deleted Journal (2024) Vol. 1, Iss. 1
Open Access | Times Cited: 2
Alessia de Biase, Nanna M. Sijtsema, Tomas Janssen, et al.
Deleted Journal (2024) Vol. 1, Iss. 1
Open Access | Times Cited: 2
Quantifying and visualising uncertainty in deep learning-based segmentation for radiation therapy treatment planning: What do radiation oncologists and therapists want?
Margerie Huet‐Dastarac, N M C van Acht, Federica Carmen Maruccio, et al.
Radiotherapy and Oncology (2024) Vol. 201, pp. 110545-110545
Closed Access | Times Cited: 1
Margerie Huet‐Dastarac, N M C van Acht, Federica Carmen Maruccio, et al.
Radiotherapy and Oncology (2024) Vol. 201, pp. 110545-110545
Closed Access | Times Cited: 1
Uncertainty quantification in multi‐class segmentation: Comparison between Bayesian and non‐Bayesian approaches in a clinical perspective
Elisa Scalco, Silvia Pozzi, Giovanna Rizzo, et al.
Medical Physics (2024) Vol. 51, Iss. 9, pp. 6090-6102
Open Access
Elisa Scalco, Silvia Pozzi, Giovanna Rizzo, et al.
Medical Physics (2024) Vol. 51, Iss. 9, pp. 6090-6102
Open Access
Implementation of delineation error detection systems in time-critical radiotherapy: Do AI-supported optimization and human preferences meet?
Nicolas F. Chaves-de-Plaza, Prerak Mody, Klaus Hildebrandt, et al.
Cognition Technology & Work (2024)
Open Access
Nicolas F. Chaves-de-Plaza, Prerak Mody, Klaus Hildebrandt, et al.
Cognition Technology & Work (2024)
Open Access