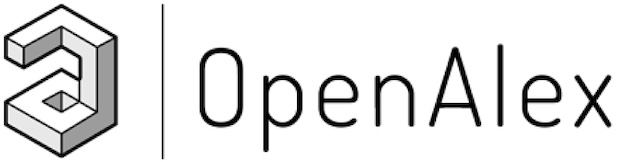
OpenAlex is a bibliographic catalogue of scientific papers, authors and institutions accessible in open access mode, named after the Library of Alexandria. It's citation coverage is excellent and I hope you will find utility in this listing of citing articles!
If you click the article title, you'll navigate to the article, as listed in CrossRef. If you click the Open Access links, you'll navigate to the "best Open Access location". Clicking the citation count will open this listing for that article. Lastly at the bottom of the page, you'll find basic pagination options.
Requested Article:
Machine learning-assisted directed protein evolution with combinatorial libraries
Zachary Wu, S. B. Jennifer Kan, Russell D. Lewis, et al.
Proceedings of the National Academy of Sciences (2019) Vol. 116, Iss. 18, pp. 8852-8858
Open Access | Times Cited: 492
Zachary Wu, S. B. Jennifer Kan, Russell D. Lewis, et al.
Proceedings of the National Academy of Sciences (2019) Vol. 116, Iss. 18, pp. 8852-8858
Open Access | Times Cited: 492
Showing 1-25 of 492 citing articles:
Machine-learning-guided directed evolution for protein engineering
Kevin Yang, Zachary Wu, Frances H. Arnold
Nature Methods (2019) Vol. 16, Iss. 8, pp. 687-694
Open Access | Times Cited: 823
Kevin Yang, Zachary Wu, Frances H. Arnold
Nature Methods (2019) Vol. 16, Iss. 8, pp. 687-694
Open Access | Times Cited: 823
Engineering new catalytic activities in enzymes
Kai Chen, Frances H. Arnold
Nature Catalysis (2020) Vol. 3, Iss. 3, pp. 203-213
Closed Access | Times Cited: 647
Kai Chen, Frances H. Arnold
Nature Catalysis (2020) Vol. 3, Iss. 3, pp. 203-213
Closed Access | Times Cited: 647
Directed Evolution: Methodologies and Applications
Yajie Wang, Pu Xue, Mingfeng Cao, et al.
Chemical Reviews (2021) Vol. 121, Iss. 20, pp. 12384-12444
Closed Access | Times Cited: 394
Yajie Wang, Pu Xue, Mingfeng Cao, et al.
Chemical Reviews (2021) Vol. 121, Iss. 20, pp. 12384-12444
Closed Access | Times Cited: 394
The Crucial Role of Methodology Development in Directed Evolution of Selective Enzymes
Ge Qu, Aitao Li, Carlos G. Acevedo‐Rocha, et al.
Angewandte Chemie International Edition (2019) Vol. 59, Iss. 32, pp. 13204-13231
Closed Access | Times Cited: 387
Ge Qu, Aitao Li, Carlos G. Acevedo‐Rocha, et al.
Angewandte Chemie International Edition (2019) Vol. 59, Iss. 32, pp. 13204-13231
Closed Access | Times Cited: 387
Machine Learning in Enzyme Engineering
Stanislav Mazurenko, Zbyněk Prokop, Jiřı́ Damborský
ACS Catalysis (2019) Vol. 10, Iss. 2, pp. 1210-1223
Open Access | Times Cited: 328
Stanislav Mazurenko, Zbyněk Prokop, Jiřı́ Damborský
ACS Catalysis (2019) Vol. 10, Iss. 2, pp. 1210-1223
Open Access | Times Cited: 328
Low-N protein engineering with data-efficient deep learning
Surojit Biswas, Grigory Khimulya, Ethan C. Alley, et al.
Nature Methods (2021) Vol. 18, Iss. 4, pp. 389-396
Open Access | Times Cited: 297
Surojit Biswas, Grigory Khimulya, Ethan C. Alley, et al.
Nature Methods (2021) Vol. 18, Iss. 4, pp. 389-396
Open Access | Times Cited: 297
Metabolic Engineering of Escherichia coli for Natural Product Biosynthesis
Dongsoo Yang, Seon Young Park, Yae Seul Park, et al.
Trends in biotechnology (2020) Vol. 38, Iss. 7, pp. 745-765
Closed Access | Times Cited: 275
Dongsoo Yang, Seon Young Park, Yae Seul Park, et al.
Trends in biotechnology (2020) Vol. 38, Iss. 7, pp. 745-765
Closed Access | Times Cited: 275
Embracing Nature’s Catalysts: A Viewpoint on the Future of Biocatalysis
Bernhard Hauer
ACS Catalysis (2020) Vol. 10, Iss. 15, pp. 8418-8427
Closed Access | Times Cited: 231
Bernhard Hauer
ACS Catalysis (2020) Vol. 10, Iss. 15, pp. 8418-8427
Closed Access | Times Cited: 231
Navigating the Unnatural Reaction Space: Directed Evolution of Heme Proteins for Selective Carbene and Nitrene Transfer
Yang Yang, Frances H. Arnold
Accounts of Chemical Research (2021) Vol. 54, Iss. 5, pp. 1209-1225
Open Access | Times Cited: 231
Yang Yang, Frances H. Arnold
Accounts of Chemical Research (2021) Vol. 54, Iss. 5, pp. 1209-1225
Open Access | Times Cited: 231
Deep diversification of an AAV capsid protein by machine learning
Drew Bryant, Ali Bashir, Sam Sinai, et al.
Nature Biotechnology (2021) Vol. 39, Iss. 6, pp. 691-696
Closed Access | Times Cited: 224
Drew Bryant, Ali Bashir, Sam Sinai, et al.
Nature Biotechnology (2021) Vol. 39, Iss. 6, pp. 691-696
Closed Access | Times Cited: 224
Optimization of therapeutic antibodies by predicting antigen specificity from antibody sequence via deep learning
Derek M. Mason, Simon Friedensohn, Cédric R. Weber, et al.
Nature Biomedical Engineering (2021) Vol. 5, Iss. 6, pp. 600-612
Closed Access | Times Cited: 217
Derek M. Mason, Simon Friedensohn, Cédric R. Weber, et al.
Nature Biomedical Engineering (2021) Vol. 5, Iss. 6, pp. 600-612
Closed Access | Times Cited: 217
The road to fully programmable protein catalysis
Sarah L. Lovelock, Rebecca Crawshaw, Sophie Basler, et al.
Nature (2022) Vol. 606, Iss. 7912, pp. 49-58
Closed Access | Times Cited: 217
Sarah L. Lovelock, Rebecca Crawshaw, Sophie Basler, et al.
Nature (2022) Vol. 606, Iss. 7912, pp. 49-58
Closed Access | Times Cited: 217
Advances in ultrahigh-throughput screening for directed enzyme evolution
Ulrich Markel, Khalil Essani, Volkan Besirlioglu, et al.
Chemical Society Reviews (2019) Vol. 49, Iss. 1, pp. 233-262
Closed Access | Times Cited: 212
Ulrich Markel, Khalil Essani, Volkan Besirlioglu, et al.
Chemical Society Reviews (2019) Vol. 49, Iss. 1, pp. 233-262
Closed Access | Times Cited: 212
Machine learning for metabolic engineering: A review
Christopher E. Lawson, Jose Manuel Martí, Tijana Radivojević, et al.
Metabolic Engineering (2020) Vol. 63, pp. 34-60
Open Access | Times Cited: 198
Christopher E. Lawson, Jose Manuel Martí, Tijana Radivojević, et al.
Metabolic Engineering (2020) Vol. 63, pp. 34-60
Open Access | Times Cited: 198
Expanding the enzyme universe with genetically encoded unnatural amino acids
Ivana Drienovská, Gérard Roelfes
Nature Catalysis (2020) Vol. 3, Iss. 3, pp. 193-202
Closed Access | Times Cited: 193
Ivana Drienovská, Gérard Roelfes
Nature Catalysis (2020) Vol. 3, Iss. 3, pp. 193-202
Closed Access | Times Cited: 193
ProGen: Language Modeling for Protein Generation
Ali Madani, Bryan McCann, Nikhil Naik, et al.
bioRxiv (Cold Spring Harbor Laboratory) (2020)
Open Access | Times Cited: 186
Ali Madani, Bryan McCann, Nikhil Naik, et al.
bioRxiv (Cold Spring Harbor Laboratory) (2020)
Open Access | Times Cited: 186
Deep Dive into Machine Learning Models for Protein Engineering
Yuting Xu, Deeptak Verma, Robert P. Sheridan, et al.
Journal of Chemical Information and Modeling (2020) Vol. 60, Iss. 6, pp. 2773-2790
Open Access | Times Cited: 182
Yuting Xu, Deeptak Verma, Robert P. Sheridan, et al.
Journal of Chemical Information and Modeling (2020) Vol. 60, Iss. 6, pp. 2773-2790
Open Access | Times Cited: 182
From nature to industry: Harnessing enzymes for biocatalysis
Rebecca Buller, Stefan Lutz, Romas J. Kazlauskas, et al.
Science (2023) Vol. 382, Iss. 6673
Open Access | Times Cited: 168
Rebecca Buller, Stefan Lutz, Romas J. Kazlauskas, et al.
Science (2023) Vol. 382, Iss. 6673
Open Access | Times Cited: 168
Biocatalysis making waves in organic chemistry
Ulf Hanefeld, Frank Hollmann, Caroline E. Paul
Chemical Society Reviews (2021) Vol. 51, Iss. 2, pp. 594-627
Open Access | Times Cited: 160
Ulf Hanefeld, Frank Hollmann, Caroline E. Paul
Chemical Society Reviews (2021) Vol. 51, Iss. 2, pp. 594-627
Open Access | Times Cited: 160
Protein Engineering for Improving and Diversifying Natural Product Biosynthesis
Chenyi Li, Ruihua Zhang, Jian Wang, et al.
Trends in biotechnology (2020) Vol. 38, Iss. 7, pp. 729-744
Open Access | Times Cited: 155
Chenyi Li, Ruihua Zhang, Jian Wang, et al.
Trends in biotechnology (2020) Vol. 38, Iss. 7, pp. 729-744
Open Access | Times Cited: 155
Informed training set design enables efficient machine learning-assisted directed protein evolution
Bruce J. Wittmann, Yisong Yue, Frances H. Arnold
Cell Systems (2021) Vol. 12, Iss. 11, pp. 1026-1045.e7
Open Access | Times Cited: 152
Bruce J. Wittmann, Yisong Yue, Frances H. Arnold
Cell Systems (2021) Vol. 12, Iss. 11, pp. 1026-1045.e7
Open Access | Times Cited: 152
Machine learning applications in systems metabolic engineering
Gi Bae Kim, Won Jun Kim, Hyun Uk Kim, et al.
Current Opinion in Biotechnology (2019) Vol. 64, pp. 1-9
Closed Access | Times Cited: 142
Gi Bae Kim, Won Jun Kim, Hyun Uk Kim, et al.
Current Opinion in Biotechnology (2019) Vol. 64, pp. 1-9
Closed Access | Times Cited: 142
Directed Evolution of a Selective and Sensitive Serotonin Sensor via Machine Learning
Elizabeth K. Unger, Jacob P. Keller, Michael Altermatt, et al.
Cell (2020) Vol. 183, Iss. 7, pp. 1986-2002.e26
Open Access | Times Cited: 140
Elizabeth K. Unger, Jacob P. Keller, Michael Altermatt, et al.
Cell (2020) Vol. 183, Iss. 7, pp. 1986-2002.e26
Open Access | Times Cited: 140
Combining chemistry and protein engineering for new-to-nature biocatalysis
David C. Miller, Soumitra V. Athavale, Frances H. Arnold
Nature Synthesis (2022) Vol. 1, Iss. 1, pp. 18-23
Open Access | Times Cited: 129
David C. Miller, Soumitra V. Athavale, Frances H. Arnold
Nature Synthesis (2022) Vol. 1, Iss. 1, pp. 18-23
Open Access | Times Cited: 129
ECNet is an evolutionary context-integrated deep learning framework for protein engineering
Yunan Luo, Guangde Jiang, Tianhao Yu, et al.
Nature Communications (2021) Vol. 12, Iss. 1
Open Access | Times Cited: 122
Yunan Luo, Guangde Jiang, Tianhao Yu, et al.
Nature Communications (2021) Vol. 12, Iss. 1
Open Access | Times Cited: 122