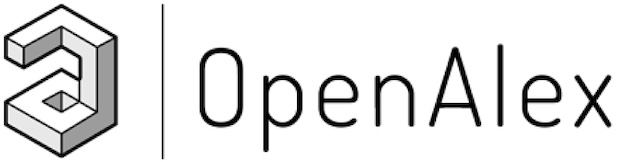
OpenAlex is a bibliographic catalogue of scientific papers, authors and institutions accessible in open access mode, named after the Library of Alexandria. It's citation coverage is excellent and I hope you will find utility in this listing of citing articles!
If you click the article title, you'll navigate to the article, as listed in CrossRef. If you click the Open Access links, you'll navigate to the "best Open Access location". Clicking the citation count will open this listing for that article. Lastly at the bottom of the page, you'll find basic pagination options.
Requested Article:
Low-cost machine learning prediction of excited state properties of iridium-centered phosphors
Gianmarco Terrones, Chenru Duan, Aditya Nandy, et al.
Chemical Science (2023) Vol. 14, Iss. 6, pp. 1419-1433
Open Access | Times Cited: 24
Gianmarco Terrones, Chenru Duan, Aditya Nandy, et al.
Chemical Science (2023) Vol. 14, Iss. 6, pp. 1419-1433
Open Access | Times Cited: 24
Showing 24 citing articles:
Combined Machine Learning, Computational, and Experimental Analysis of the Iridium(III) Complexes with Red to Near-Infrared Emission
Anas Karuth, Gerardo M. Casañola‐Martín, Levi Lystrom, et al.
The Journal of Physical Chemistry Letters (2024) Vol. 15, Iss. 2, pp. 471-480
Closed Access | Times Cited: 7
Anas Karuth, Gerardo M. Casañola‐Martín, Levi Lystrom, et al.
The Journal of Physical Chemistry Letters (2024) Vol. 15, Iss. 2, pp. 471-480
Closed Access | Times Cited: 7
David Dalmau, Juan V. Alegre‐Requena
Wiley Interdisciplinary Reviews Computational Molecular Science (2024) Vol. 14, Iss. 5
Open Access | Times Cited: 6
Dual-Level Parametrically Managed Neural Network Method for Learning a Potential Energy Surface for Efficient Dynamics
Suman Bhaumik, Dayou Zhang, Yinan Shu, et al.
Journal of Chemical Theory and Computation (2025)
Closed Access
Suman Bhaumik, Dayou Zhang, Yinan Shu, et al.
Journal of Chemical Theory and Computation (2025)
Closed Access
Stacking machine learning models for predicting photophysical properties of iridium complexes
Ding Peng, Lin Liu, Bin‐Bin Xie, et al.
Journal of Photochemistry and Photobiology A Chemistry (2025), pp. 116374-116374
Closed Access
Ding Peng, Lin Liu, Bin‐Bin Xie, et al.
Journal of Photochemistry and Photobiology A Chemistry (2025), pp. 116374-116374
Closed Access
Using experimental data in computationally guided rational design of inorganic materials with machine learning
Heather J. Kulik
Journal of materials research/Pratt's guide to venture capital sources (2025)
Open Access
Heather J. Kulik
Journal of materials research/Pratt's guide to venture capital sources (2025)
Open Access
Interpretable data-driven modeling on the excited state mechanisms of (Ni, Pt)-centered phosphorescent materials
Kaushik Soni, Omkar Chaudhari, Kalishankar Bhattacharyya
Theoretical Chemistry Accounts (2025) Vol. 144, Iss. 4
Closed Access
Kaushik Soni, Omkar Chaudhari, Kalishankar Bhattacharyya
Theoretical Chemistry Accounts (2025) Vol. 144, Iss. 4
Closed Access
Data-Driven Insights into Porphyrin Geometry: Interpretable AI for Non-Planarity and Aromaticity Analyses
Shachar Fite, Zeev Gross
Journal of Chemical Information and Modeling (2025)
Closed Access
Shachar Fite, Zeev Gross
Journal of Chemical Information and Modeling (2025)
Closed Access
Comment on ‘Physics-based representations for machine learning properties of chemical reactions’
Kevin Spiekermann, Thijs Stuyver, Lagnajit Pattanaik, et al.
Machine Learning Science and Technology (2023) Vol. 4, Iss. 4, pp. 048001-048001
Open Access | Times Cited: 10
Kevin Spiekermann, Thijs Stuyver, Lagnajit Pattanaik, et al.
Machine Learning Science and Technology (2023) Vol. 4, Iss. 4, pp. 048001-048001
Open Access | Times Cited: 10
Parametrically Managed Activation Function for Fitting a Neural Network Potential with Physical Behavior Enforced by a Low-Dimensional Potential
Farideh Badichi Akher, Yinan Shu, Zoltán Varga, et al.
The Journal of Physical Chemistry A (2023) Vol. 127, Iss. 24, pp. 5287-5297
Closed Access | Times Cited: 9
Farideh Badichi Akher, Yinan Shu, Zoltán Varga, et al.
The Journal of Physical Chemistry A (2023) Vol. 127, Iss. 24, pp. 5287-5297
Closed Access | Times Cited: 9
Predicting Emission Spectra of Heteroleptic Iridium Complexes Using Artificial Chemical Intelligence
Yudhajit Pal, Tahoe A. Fiala, Wesley B. Swords, et al.
ChemPhysChem (2024) Vol. 25, Iss. 16
Open Access | Times Cited: 2
Yudhajit Pal, Tahoe A. Fiala, Wesley B. Swords, et al.
ChemPhysChem (2024) Vol. 25, Iss. 16
Open Access | Times Cited: 2
Insights into the Luminescence Quantum Yields of Cyclometalated Iridium(III) Complexes: A Density Functional Theory and Machine Learning Approach
Miho Hatanaka, Hiromoto Kato, Minami Sakai, et al.
The Journal of Physical Chemistry A (2023) Vol. 127, Iss. 36, pp. 7630-7637
Closed Access | Times Cited: 5
Miho Hatanaka, Hiromoto Kato, Minami Sakai, et al.
The Journal of Physical Chemistry A (2023) Vol. 127, Iss. 36, pp. 7630-7637
Closed Access | Times Cited: 5
Reply to Comment on ‘Physics-based representations for machine learning properties of chemical reactions’
Puck van Gerwen, Matthew D. Wodrich, Rubén Laplaza, et al.
Machine Learning Science and Technology (2023) Vol. 4, Iss. 4, pp. 048002-048002
Open Access | Times Cited: 5
Puck van Gerwen, Matthew D. Wodrich, Rubén Laplaza, et al.
Machine Learning Science and Technology (2023) Vol. 4, Iss. 4, pp. 048002-048002
Open Access | Times Cited: 5
Machine Learning Validation via Rational Dataset Sampling with astartes
Jackson Burns, Kevin Spiekermann, Himaghna Bhattacharjee, et al.
The Journal of Open Source Software (2023) Vol. 8, Iss. 91, pp. 5996-5996
Open Access | Times Cited: 5
Jackson Burns, Kevin Spiekermann, Himaghna Bhattacharjee, et al.
The Journal of Open Source Software (2023) Vol. 8, Iss. 91, pp. 5996-5996
Open Access | Times Cited: 5
ROBERT: Bridging the Gap between Machine Learning and Chemistry
David Dalmau, Juan V. Alegre‐Requena
(2023)
Open Access | Times Cited: 4
David Dalmau, Juan V. Alegre‐Requena
(2023)
Open Access | Times Cited: 4
Exploring Optimized Organic Fluorophore Search Through Simple Experimental Data-Driven VAE
Cheng‐Wei Ju, Yuzhi Xu, Yongrui Luo, et al.
(2024)
Open Access | Times Cited: 1
Cheng‐Wei Ju, Yuzhi Xu, Yongrui Luo, et al.
(2024)
Open Access | Times Cited: 1
Exploring Optimized Organic Fluorophore Search Through Simple Experimental Data-Driven VAE
Cheng‐Wei Ju, Yuzhi Xu, Yongrui Luo, et al.
(2024)
Open Access | Times Cited: 1
Cheng‐Wei Ju, Yuzhi Xu, Yongrui Luo, et al.
(2024)
Open Access | Times Cited: 1
Deep Learning Prediction of Triplet–Triplet Annihilation Parameters in Blue Fluorescent Organic Light‐Emitting Diodes
Junseop Lim, Jae‐Min Kim, Jun Yeob Lee
Advanced Materials (2024) Vol. 36, Iss. 28
Closed Access | Times Cited: 1
Junseop Lim, Jae‐Min Kim, Jun Yeob Lee
Advanced Materials (2024) Vol. 36, Iss. 28
Closed Access | Times Cited: 1
Parametrically Managed Activation Function for a Fitting a Neural Network Potential with Physical Behavior Enforced by a Low-Dimensional Potential
Farideh Badichi Akher, Yinan Shu, Zoltán M. Varga, et al.
(2023)
Open Access | Times Cited: 2
Farideh Badichi Akher, Yinan Shu, Zoltán M. Varga, et al.
(2023)
Open Access | Times Cited: 2
Accurately Predicting Barrier Heights for Radical Reactions in Solution Using Deep Graph Networks
Kevin Spiekermann, Xiaorui Dong, Angiras Menon, et al.
The Journal of Physical Chemistry A (2024)
Closed Access
Kevin Spiekermann, Xiaorui Dong, Angiras Menon, et al.
The Journal of Physical Chemistry A (2024)
Closed Access
Chemical Feature-Based Machine Learning Model for Predicting Photophysical Properties of BODIPY Compounds: Density Functional Theory and Quantitative Structure–Property Relationship Modeling
Gerardo M. Casañola‐Martín, Jing Wang, Jian‐Ge Zhou, et al.
Research Square (Research Square) (2024)
Open Access
Gerardo M. Casañola‐Martín, Jing Wang, Jian‐Ge Zhou, et al.
Research Square (Research Square) (2024)
Open Access
Discovery of Novel Iridium(III) Complex‐Based Photofunctional Materials Using Excited‐State Descriptors
Chu Wang, Lin Liu, Wei Sun, et al.
Advanced Optical Materials (2024)
Closed Access
Chu Wang, Lin Liu, Wei Sun, et al.
Advanced Optical Materials (2024)
Closed Access
Chemical feature-based machine learning model for predicting photophysical properties of BODIPY compounds: density functional theory and quantitative structure–property relationship modeling
Gerardo M. Casañola‐Martín, Jing Wang, Jian‐Ge Zhou, et al.
Journal of Molecular Modeling (2024) Vol. 31, Iss. 1
Closed Access
Gerardo M. Casañola‐Martín, Jing Wang, Jian‐Ge Zhou, et al.
Journal of Molecular Modeling (2024) Vol. 31, Iss. 1
Closed Access
Simulation-Assisted Deep Learning Techniques for Commercially Applicable OLED Phosphorescent Materials
Kisoo Kwon, Kuhwan Jeong, Sanghyun Yoo, et al.
Chemistry of Materials (2024) Vol. 37, Iss. 1, pp. 387-399
Closed Access
Kisoo Kwon, Kuhwan Jeong, Sanghyun Yoo, et al.
Chemistry of Materials (2024) Vol. 37, Iss. 1, pp. 387-399
Closed Access
Post-Activation Function for Managing the Behavior of a Neural Network Potential with a Low-Dimensional Potential
Farideh Badichi Akher, Yinan Shu, Zoltán M. Varga, et al.
(2023)
Open Access
Farideh Badichi Akher, Yinan Shu, Zoltán M. Varga, et al.
(2023)
Open Access