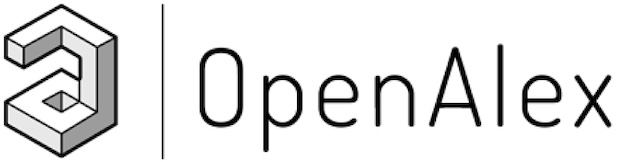
OpenAlex is a bibliographic catalogue of scientific papers, authors and institutions accessible in open access mode, named after the Library of Alexandria. It's citation coverage is excellent and I hope you will find utility in this listing of citing articles!
If you click the article title, you'll navigate to the article, as listed in CrossRef. If you click the Open Access links, you'll navigate to the "best Open Access location". Clicking the citation count will open this listing for that article. Lastly at the bottom of the page, you'll find basic pagination options.
Requested Article:
Deep learning metal complex properties with natural quantum graphs
Hannes Kneiding, Ruslan Lukin, Lucas Lang, et al.
Digital Discovery (2023) Vol. 2, Iss. 3, pp. 618-633
Open Access | Times Cited: 21
Hannes Kneiding, Ruslan Lukin, Lucas Lang, et al.
Digital Discovery (2023) Vol. 2, Iss. 3, pp. 618-633
Open Access | Times Cited: 21
Showing 21 citing articles:
Directional multiobjective optimization of metal complexes at the billion-system scale
Hannes Kneiding, Ainara Nova, David Balcells
Nature Computational Science (2024) Vol. 4, Iss. 4, pp. 263-273
Open Access | Times Cited: 11
Hannes Kneiding, Ainara Nova, David Balcells
Nature Computational Science (2024) Vol. 4, Iss. 4, pp. 263-273
Open Access | Times Cited: 11
Automated Transition Metal Catalysts Discovery and Optimisation with AI and Machine Learning
S. Macé, Yingjian Xu, Bao N. Nguyen
ChemCatChem (2024) Vol. 16, Iss. 10
Open Access | Times Cited: 7
S. Macé, Yingjian Xu, Bao N. Nguyen
ChemCatChem (2024) Vol. 16, Iss. 10
Open Access | Times Cited: 7
Hybrid DFT Geometries and Properties for 17k Lanthanoid Complexes─The LnQM Data Set
Christian Hölzer, Igor Gordiy, Stefan Grimme, et al.
Journal of Chemical Information and Modeling (2024) Vol. 64, Iss. 3, pp. 825-836
Closed Access | Times Cited: 5
Christian Hölzer, Igor Gordiy, Stefan Grimme, et al.
Journal of Chemical Information and Modeling (2024) Vol. 64, Iss. 3, pp. 825-836
Closed Access | Times Cited: 5
Zinc metal complexes synthesized by a green method as a new approach to alter the structural and optical characteristics of PVA: new field for polymer composite fabrication with controlled optical band gap
Dana S. Muhammad, Dara Muhammed Aziz, Shujahadeen B. Aziz
RSC Advances (2024) Vol. 14, Iss. 36, pp. 26362-26387
Open Access | Times Cited: 5
Dana S. Muhammad, Dara Muhammed Aziz, Shujahadeen B. Aziz
RSC Advances (2024) Vol. 14, Iss. 36, pp. 26362-26387
Open Access | Times Cited: 5
Using Machine Learning to Predict the Antibacterial Activity of Ruthenium Complexes**
Markus Orsi, Boon Shing Loh, Cheng Weng, et al.
Angewandte Chemie International Edition (2023) Vol. 63, Iss. 10
Closed Access | Times Cited: 13
Markus Orsi, Boon Shing Loh, Cheng Weng, et al.
Angewandte Chemie International Edition (2023) Vol. 63, Iss. 10
Closed Access | Times Cited: 13
ReaLigands: A Ligand Library Cultivated from Experiment and Intended for Molecular Computational Catalyst Design
Shusen Chen, Zack Meyer, Brendan Jensen, et al.
Journal of Chemical Information and Modeling (2023) Vol. 63, Iss. 23, pp. 7412-7422
Closed Access | Times Cited: 8
Shusen Chen, Zack Meyer, Brendan Jensen, et al.
Journal of Chemical Information and Modeling (2023) Vol. 63, Iss. 23, pp. 7412-7422
Closed Access | Times Cited: 8
Deep Generative Model for the Dual-Objective Inverse Design of Metal Complexes
Magnus Strandgaard, Trond Linjordet, Hannes Kneiding, et al.
(2024)
Open Access | Times Cited: 2
Magnus Strandgaard, Trond Linjordet, Hannes Kneiding, et al.
(2024)
Open Access | Times Cited: 2
Unsupervised manifold embedding to encode molecular quantum information for supervised learning of chemical data
Tonglei Li, Nicholas J. Huls, Shan Lu, et al.
Communications Chemistry (2024) Vol. 7, Iss. 1
Open Access | Times Cited: 2
Tonglei Li, Nicholas J. Huls, Shan Lu, et al.
Communications Chemistry (2024) Vol. 7, Iss. 1
Open Access | Times Cited: 2
Molecular representations in bio-cheminformatics
Thanh‐Hoang Nguyen‐Vo, Paul Teesdale‐Spittle, Joanne E. Harvey, et al.
Memetic Computing (2024) Vol. 16, Iss. 3, pp. 519-536
Open Access | Times Cited: 2
Thanh‐Hoang Nguyen‐Vo, Paul Teesdale‐Spittle, Joanne E. Harvey, et al.
Memetic Computing (2024) Vol. 16, Iss. 3, pp. 519-536
Open Access | Times Cited: 2
Data Generation for Machine Learning Interatomic Potentials and Beyond
Maksim Kulichenko, Benjamin Nebgen, Nicholas Lubbers, et al.
Chemical Reviews (2024) Vol. 124, Iss. 24, pp. 13681-13714
Closed Access | Times Cited: 2
Maksim Kulichenko, Benjamin Nebgen, Nicholas Lubbers, et al.
Chemical Reviews (2024) Vol. 124, Iss. 24, pp. 13681-13714
Closed Access | Times Cited: 2
Applying Large Graph Neural Networks to Predict Transition Metal Complex Energies Using the tmQM_wB97MV Data Set
Aaron Garrison, Javier Heras‐Domingo, John R. Kitchin, et al.
Journal of Chemical Information and Modeling (2023) Vol. 63, Iss. 24, pp. 7642-7654
Open Access | Times Cited: 7
Aaron Garrison, Javier Heras‐Domingo, John R. Kitchin, et al.
Journal of Chemical Information and Modeling (2023) Vol. 63, Iss. 24, pp. 7642-7654
Open Access | Times Cited: 7
Directional Multiobjective Optimization of Metal Complexes at the Billion-Scale with the tmQMg-L Dataset and PL-MOGA Algorithm
Hannes Kneiding, Ainara Nova, David Balcells
(2023)
Open Access | Times Cited: 6
Hannes Kneiding, Ainara Nova, David Balcells
(2023)
Open Access | Times Cited: 6
AABBA Graph Kernel: Atom-Atom, Bond-Bond, and Bond-Atom Autocorrelations for Machine Learning
Lucía Morán‐González, Jørn Eirik Betten, Hannes Kneiding, et al.
Journal of Chemical Information and Modeling (2024)
Open Access | Times Cited: 1
Lucía Morán‐González, Jørn Eirik Betten, Hannes Kneiding, et al.
Journal of Chemical Information and Modeling (2024)
Open Access | Times Cited: 1
Deep Graph Learning for Molecules and Materials
Hannes Kneiding, David Balcells
Nordic Machine Intelligence (2023) Vol. 3, Iss. 2, pp. 8-14
Open Access | Times Cited: 2
Hannes Kneiding, David Balcells
Nordic Machine Intelligence (2023) Vol. 3, Iss. 2, pp. 8-14
Open Access | Times Cited: 2
Toward AI/ML-assisted discovery of transition metal complexes
H.-Q. Jin, Kenneth M. Merz
Annual reports in computational chemistry (2024)
Closed Access
H.-Q. Jin, Kenneth M. Merz
Annual reports in computational chemistry (2024)
Closed Access
Hidden descriptors: Using statistical treatments to generate better descriptor sets
Lucía Morán‐González, Feliu Maseras
Artificial Intelligence Chemistry (2024) Vol. 2, Iss. 1, pp. 100061-100061
Open Access
Lucía Morán‐González, Feliu Maseras
Artificial Intelligence Chemistry (2024) Vol. 2, Iss. 1, pp. 100061-100061
Open Access
Discovering metal complexes in vast chemical spaces
Nature Computational Science (2024) Vol. 4, Iss. 4, pp. 259-260
Closed Access
Nature Computational Science (2024) Vol. 4, Iss. 4, pp. 259-260
Closed Access
Molecular quantum chemical data sets and databases for machine learning potentials
Arif Ullah, Yuxinxin Chen, Pavlo O. Dral
Machine Learning Science and Technology (2024) Vol. 5, Iss. 4, pp. 041001-041001
Open Access
Arif Ullah, Yuxinxin Chen, Pavlo O. Dral
Machine Learning Science and Technology (2024) Vol. 5, Iss. 4, pp. 041001-041001
Open Access
Beyond Chemical Structures: Lessons and Guiding Principles for the Next Generation of Molecular Databases
Timo Sommer, Cian Clarke, Max Garcı́a-Melchor
Chemical Science (2024)
Open Access
Timo Sommer, Cian Clarke, Max Garcı́a-Melchor
Chemical Science (2024)
Open Access
AABBA: Atom–Atom Bond–Bond Bond–Atom Graph Kernel for Machine Learning on Molecules and Materials
Lucía Morán‐González, Jørn Eirik Betten, Hannes Kneiding, et al.
(2023)
Open Access
Lucía Morán‐González, Jørn Eirik Betten, Hannes Kneiding, et al.
(2023)
Open Access
Maschinelles Lernen zur Vorhersage antibakterieller Aktivität von Ruthenium‐Komplexen**
Markus Orsi, Boon Shing Loh, Cheng Weng, et al.
Angewandte Chemie (2023) Vol. 136, Iss. 10
Closed Access
Markus Orsi, Boon Shing Loh, Cheng Weng, et al.
Angewandte Chemie (2023) Vol. 136, Iss. 10
Closed Access