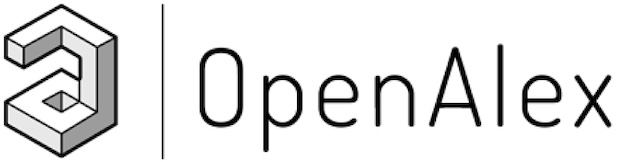
OpenAlex is a bibliographic catalogue of scientific papers, authors and institutions accessible in open access mode, named after the Library of Alexandria. It's citation coverage is excellent and I hope you will find utility in this listing of citing articles!
If you click the article title, you'll navigate to the article, as listed in CrossRef. If you click the Open Access links, you'll navigate to the "best Open Access location". Clicking the citation count will open this listing for that article. Lastly at the bottom of the page, you'll find basic pagination options.
Requested Article:
A first-principles and machine-learning investigation on the electronic, photocatalytic, mechanical and heat conduction properties of nanoporous C5N monolayers
Bohayra Mortazavi, Masoud Shahrokhi, Fazel Shojaei, et al.
Nanoscale (2022) Vol. 14, Iss. 11, pp. 4324-4333
Closed Access | Times Cited: 41
Bohayra Mortazavi, Masoud Shahrokhi, Fazel Shojaei, et al.
Nanoscale (2022) Vol. 14, Iss. 11, pp. 4324-4333
Closed Access | Times Cited: 41
Showing 1-25 of 41 citing articles:
Atomistic modeling of the mechanical properties: the rise of machine learning interatomic potentials
Bohayra Mortazavi, Xiaoying Zhuang, Timon Rabczuk, et al.
Materials Horizons (2023) Vol. 10, Iss. 6, pp. 1956-1968
Open Access | Times Cited: 61
Bohayra Mortazavi, Xiaoying Zhuang, Timon Rabczuk, et al.
Materials Horizons (2023) Vol. 10, Iss. 6, pp. 1956-1968
Open Access | Times Cited: 61
Predicting lattice thermal conductivity via machine learning: a mini review
Yufeng Luo, Mengke Li, Hongmei Yuan, et al.
npj Computational Materials (2023) Vol. 9, Iss. 1
Open Access | Times Cited: 51
Yufeng Luo, Mengke Li, Hongmei Yuan, et al.
npj Computational Materials (2023) Vol. 9, Iss. 1
Open Access | Times Cited: 51
When Machine Learning Meets 2D Materials: A Review
Bin Lu, Yuze Xia, Yuqian Ren, et al.
Advanced Science (2024) Vol. 11, Iss. 13
Open Access | Times Cited: 46
Bin Lu, Yuze Xia, Yuqian Ren, et al.
Advanced Science (2024) Vol. 11, Iss. 13
Open Access | Times Cited: 46
CxNy-based materials as gas sensors: Structure, performance, mechanism and perspective
Mengshan Chen, Haoran Zhang, Hui Li, et al.
Coordination Chemistry Reviews (2024) Vol. 503, pp. 215653-215653
Open Access | Times Cited: 37
Mengshan Chen, Haoran Zhang, Hui Li, et al.
Coordination Chemistry Reviews (2024) Vol. 503, pp. 215653-215653
Open Access | Times Cited: 37
Recent Advances in Machine Learning‐Assisted Multiscale Design of Energy Materials
Bohayra Mortazavi
Advanced Energy Materials (2024)
Open Access | Times Cited: 16
Bohayra Mortazavi
Advanced Energy Materials (2024)
Open Access | Times Cited: 16
High solar-to-hydrogen efficiency photocatalytic hydrogen evolution reaction with the HfSe2/InSe heterostructure
Rui Sun, Chuan‐Lu Yang, Meishan Wang, et al.
Journal of Power Sources (2022) Vol. 547, pp. 232008-232008
Closed Access | Times Cited: 60
Rui Sun, Chuan‐Lu Yang, Meishan Wang, et al.
Journal of Power Sources (2022) Vol. 547, pp. 232008-232008
Closed Access | Times Cited: 60
Structural, electronic, thermal and mechanical properties of C60-based fullerene two-dimensional networks explored by first-principles and machine learning
Bohayra Mortazavi
Carbon (2023) Vol. 213, pp. 118293-118293
Open Access | Times Cited: 34
Bohayra Mortazavi
Carbon (2023) Vol. 213, pp. 118293-118293
Open Access | Times Cited: 34
Construction of High Accuracy Machine Learning Interatomic Potential for Surface/Interface of Nanomaterials—A Review
Kaiwei Wan, Jianxin He, Xinghua Shi
Advanced Materials (2023) Vol. 36, Iss. 22
Closed Access | Times Cited: 23
Kaiwei Wan, Jianxin He, Xinghua Shi
Advanced Materials (2023) Vol. 36, Iss. 22
Closed Access | Times Cited: 23
Navigating the Evolution of Carbon Nitride Research: Integrating Machine Learning into Conventional Approaches
Deep Mondal, Sujoy Datta, Debnarayan Jana
Physical Chemistry Chemical Physics (2025)
Closed Access | Times Cited: 1
Deep Mondal, Sujoy Datta, Debnarayan Jana
Physical Chemistry Chemical Physics (2025)
Closed Access | Times Cited: 1
A combined first-principles and machine-learning investigation on the stability, electronic, optical, and mechanical properties of novel C6N7-based nanoporous carbon nitrides
Bohayra Mortazavi, Fazel Shojaei, Alexander V. Shapeev, et al.
Carbon (2022) Vol. 194, pp. 230-239
Closed Access | Times Cited: 37
Bohayra Mortazavi, Fazel Shojaei, Alexander V. Shapeev, et al.
Carbon (2022) Vol. 194, pp. 230-239
Closed Access | Times Cited: 37
A novel two-dimensional C36 fullerene network; an isotropic, auxetic semiconductor with low thermal conductivity and remarkable stiffness
Bohayra Mortazavi, Fazel Shojaei, Xiaoying Zhuang
Materials Today Nano (2022) Vol. 21, pp. 100280-100280
Closed Access | Times Cited: 35
Bohayra Mortazavi, Fazel Shojaei, Xiaoying Zhuang
Materials Today Nano (2022) Vol. 21, pp. 100280-100280
Closed Access | Times Cited: 35
Grain boundary sliding and distortion on a nanosecond timescale induce trap states in CsPbBr3: ab initio investigation with machine learning force field
Dongyu Liu, Yifan Wu, Andrey S. Vasenko, et al.
Nanoscale (2022) Vol. 15, Iss. 1, pp. 285-293
Closed Access | Times Cited: 33
Dongyu Liu, Yifan Wu, Andrey S. Vasenko, et al.
Nanoscale (2022) Vol. 15, Iss. 1, pp. 285-293
Closed Access | Times Cited: 33
Switch effect on controlled water splitting by biaxial strain regulating the promising two-dimensional Janus X2PAs (X = Si, Ge and Sn) photocatalyst
Qiwen He, Yang Wu, Chunhua Yang, et al.
Nanoscale (2023) Vol. 15, Iss. 24, pp. 10458-10464
Closed Access | Times Cited: 21
Qiwen He, Yang Wu, Chunhua Yang, et al.
Nanoscale (2023) Vol. 15, Iss. 24, pp. 10458-10464
Closed Access | Times Cited: 21
Intelligent Algorithms Enable Photocatalyst Design and Performance Prediction
Shifa Wang, Panzhong Mo, Dengfeng Li, et al.
Catalysts (2024) Vol. 14, Iss. 4, pp. 217-217
Open Access | Times Cited: 5
Shifa Wang, Panzhong Mo, Dengfeng Li, et al.
Catalysts (2024) Vol. 14, Iss. 4, pp. 217-217
Open Access | Times Cited: 5
Low and Anisotropic Tensile Strength and Thermal Conductivity in the Single-Layer Fullerene Network Predicted by Machine-Learning Interatomic Potentials
Bohayra Mortazavi, Xiaoying Zhuang
Coatings (2022) Vol. 12, Iss. 8, pp. 1171-1171
Open Access | Times Cited: 21
Bohayra Mortazavi, Xiaoying Zhuang
Coatings (2022) Vol. 12, Iss. 8, pp. 1171-1171
Open Access | Times Cited: 21
Ultrahigh strength and negative thermal expansion and low thermal conductivity in graphyne nanosheets confirmed by machine-learning interatomic potentials
Bohayra Mortazavi, Xiaoying Zhuang
FlatChem (2022) Vol. 36, pp. 100446-100446
Closed Access | Times Cited: 19
Bohayra Mortazavi, Xiaoying Zhuang
FlatChem (2022) Vol. 36, pp. 100446-100446
Closed Access | Times Cited: 19
Investigation of the mechanical and transport properties of InGeX3 (X = S, Se and Te) monolayers using density functional theory and machine learning
Yong-Bo Shi, Yuan-Yuan Chen, Hao Wang, et al.
Physical Chemistry Chemical Physics (2023) Vol. 25, Iss. 20, pp. 13864-13876
Closed Access | Times Cited: 12
Yong-Bo Shi, Yuan-Yuan Chen, Hao Wang, et al.
Physical Chemistry Chemical Physics (2023) Vol. 25, Iss. 20, pp. 13864-13876
Closed Access | Times Cited: 12
Machine learning aided multiscale magnetostatics
Fadi Aldakheel, Celal Soyarslan, Hari Subramani Palanisamy, et al.
Mechanics of Materials (2023) Vol. 184, pp. 104726-104726
Open Access | Times Cited: 12
Fadi Aldakheel, Celal Soyarslan, Hari Subramani Palanisamy, et al.
Mechanics of Materials (2023) Vol. 184, pp. 104726-104726
Open Access | Times Cited: 12
Carbon Emission Optimization of Ultra-High-Performance Concrete Using Machine Learning Methods
Min Wang, Mingfeng Du, Yue Jia, et al.
Materials (2024) Vol. 17, Iss. 7, pp. 1670-1670
Open Access | Times Cited: 4
Min Wang, Mingfeng Du, Yue Jia, et al.
Materials (2024) Vol. 17, Iss. 7, pp. 1670-1670
Open Access | Times Cited: 4
Biaxila strain modulated high anisotropic gas-sensing performance of C5N-based two-dimensional devices: A first-principles study
H. B. Li, Zhengfang Liu, Guogang Liu, et al.
Surfaces and Interfaces (2024) Vol. 48, pp. 104277-104277
Closed Access | Times Cited: 4
H. B. Li, Zhengfang Liu, Guogang Liu, et al.
Surfaces and Interfaces (2024) Vol. 48, pp. 104277-104277
Closed Access | Times Cited: 4
Applications of machine learning in surfaces and interfaces
Shaofeng Xu, Jing‐Yuan Wu, Ying Guo, et al.
Chemical Physics Reviews (2025) Vol. 6, Iss. 1
Open Access
Shaofeng Xu, Jing‐Yuan Wu, Ying Guo, et al.
Chemical Physics Reviews (2025) Vol. 6, Iss. 1
Open Access
Machine learning for semiconductors
Duanyang Liu, Liming Xu, Xu-Min Lin, et al.
Chip (2022) Vol. 1, Iss. 4, pp. 100033-100033
Open Access | Times Cited: 17
Duanyang Liu, Liming Xu, Xu-Min Lin, et al.
Chip (2022) Vol. 1, Iss. 4, pp. 100033-100033
Open Access | Times Cited: 17
Numerical study on the static bending and forced vibration of triclinic plate with arbitrary boundary conditions
Behrouz Karami, Maziar Janghorban
Archives of Civil and Mechanical Engineering (2023) Vol. 23, Iss. 4
Closed Access | Times Cited: 10
Behrouz Karami, Maziar Janghorban
Archives of Civil and Mechanical Engineering (2023) Vol. 23, Iss. 4
Closed Access | Times Cited: 10
S-C3N6 monolayer by atomic doping serving as solar cells and photocatalyst
Yaqi She, Yutong Zou, Yuhuan Li, et al.
Colloids and Surfaces A Physicochemical and Engineering Aspects (2024) Vol. 688, pp. 133616-133616
Closed Access | Times Cited: 3
Yaqi She, Yutong Zou, Yuhuan Li, et al.
Colloids and Surfaces A Physicochemical and Engineering Aspects (2024) Vol. 688, pp. 133616-133616
Closed Access | Times Cited: 3
Exploring the structural stability, thermal and mechanical properties of nanoporous carbon nitride nanosheets using a transferrable machine learning interatomic potential
Bohayra Mortazavi, Timon Rabczuk, Xiaoying Zhuang
Machine learning for computational science and engineering (2024) Vol. 1, Iss. 1
Open Access | Times Cited: 3
Bohayra Mortazavi, Timon Rabczuk, Xiaoying Zhuang
Machine learning for computational science and engineering (2024) Vol. 1, Iss. 1
Open Access | Times Cited: 3