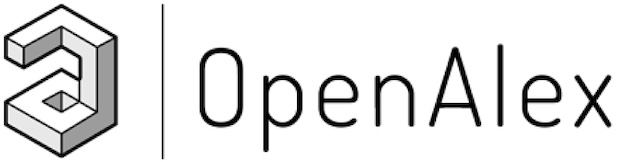
OpenAlex is a bibliographic catalogue of scientific papers, authors and institutions accessible in open access mode, named after the Library of Alexandria. It's citation coverage is excellent and I hope you will find utility in this listing of citing articles!
If you click the article title, you'll navigate to the article, as listed in CrossRef. If you click the Open Access links, you'll navigate to the "best Open Access location". Clicking the citation count will open this listing for that article. Lastly at the bottom of the page, you'll find basic pagination options.
Requested Article:
The pitfalls of negative data bias for the T-cell epitope specificity challenge
Ceder Dens, Kris Laukens, Wout Bittremieux, et al.
Nature Machine Intelligence (2023) Vol. 5, Iss. 10, pp. 1060-1062
Open Access | Times Cited: 19
Ceder Dens, Kris Laukens, Wout Bittremieux, et al.
Nature Machine Intelligence (2023) Vol. 5, Iss. 10, pp. 1060-1062
Open Access | Times Cited: 19
Showing 19 citing articles:
Adaptive immune receptor repertoire analysis
Vanessa Mhanna, Habib Bashour, Khang Lê Quý, et al.
Nature Reviews Methods Primers (2024) Vol. 4, Iss. 1
Closed Access | Times Cited: 12
Vanessa Mhanna, Habib Bashour, Khang Lê Quý, et al.
Nature Reviews Methods Primers (2024) Vol. 4, Iss. 1
Closed Access | Times Cited: 12
A unified cross-attention model for predicting antigen binding specificity to both HLA and TCR molecules
Chenpeng Yu, Xing Fang, Shiye Tian, et al.
Nature Machine Intelligence (2025)
Closed Access | Times Cited: 1
Chenpeng Yu, Xing Fang, Shiye Tian, et al.
Nature Machine Intelligence (2025)
Closed Access | Times Cited: 1
Supervised fine-tuning of pre-trained antibody language models improves antigen specificity prediction
Meng Wang, Jonathan Patsenker, Henry Li, et al.
PLoS Computational Biology (2025) Vol. 21, Iss. 3, pp. e1012153-e1012153
Open Access | Times Cited: 1
Meng Wang, Jonathan Patsenker, Henry Li, et al.
PLoS Computational Biology (2025) Vol. 21, Iss. 3, pp. e1012153-e1012153
Open Access | Times Cited: 1
Training data composition determines machine learning generalization and biological rule discovery
Eugen Ursu, Aygul R. Minnegalieva, Puneet Rawat, et al.
bioRxiv (Cold Spring Harbor Laboratory) (2024)
Open Access | Times Cited: 5
Eugen Ursu, Aygul R. Minnegalieva, Puneet Rawat, et al.
bioRxiv (Cold Spring Harbor Laboratory) (2024)
Open Access | Times Cited: 5
T-cell receptor binding prediction: A machine learning revolution
Anna Weber, Aurélien Pélissier, María Rodríguez Martínez
ImmunoInformatics (2024) Vol. 15, pp. 100040-100040
Open Access | Times Cited: 5
Anna Weber, Aurélien Pélissier, María Rodríguez Martínez
ImmunoInformatics (2024) Vol. 15, pp. 100040-100040
Open Access | Times Cited: 5
Lessons learned from the IMMREP23 TCR-epitope prediction challenge
Morten Nielsen, Anne Eugster, Mathias Fynbo Jensen, et al.
ImmunoInformatics (2024) Vol. 16, pp. 100045-100045
Open Access | Times Cited: 4
Morten Nielsen, Anne Eugster, Mathias Fynbo Jensen, et al.
ImmunoInformatics (2024) Vol. 16, pp. 100045-100045
Open Access | Times Cited: 4
Contrastive learning of T cell receptor representations
Yuta Nagano, Andrew G. T. Pyo, Martina Milighetti, et al.
Cell Systems (2025), pp. 101165-101165
Open Access
Yuta Nagano, Andrew G. T. Pyo, Martina Milighetti, et al.
Cell Systems (2025), pp. 101165-101165
Open Access
Simulation of adaptive immune receptors and repertoires with complex immune information to guide the development and benchmarking of AIRR machine learning
Maria Chernigovskaya, Milena Pavlović, Chakravarthi Kanduri, et al.
Nucleic Acids Research (2025) Vol. 53, Iss. 3
Open Access
Maria Chernigovskaya, Milena Pavlović, Chakravarthi Kanduri, et al.
Nucleic Acids Research (2025) Vol. 53, Iss. 3
Open Access
TCR-H: explainable machine learning prediction of T-cell receptor epitope binding on unseen datasets
Rajitha Rajeshwar T., Omar Demerdash, Jeremy C. Smith
Frontiers in Immunology (2024) Vol. 15
Open Access | Times Cited: 3
Rajitha Rajeshwar T., Omar Demerdash, Jeremy C. Smith
Frontiers in Immunology (2024) Vol. 15
Open Access | Times Cited: 3
Sliding-attention transformer neural architecture for predicting T cell receptor–antigen–human leucocyte antigen binding
Ziyan Feng, Jingyang Chen, Youlong Hai, et al.
Nature Machine Intelligence (2024)
Open Access | Times Cited: 3
Ziyan Feng, Jingyang Chen, Youlong Hai, et al.
Nature Machine Intelligence (2024)
Open Access | Times Cited: 3
Reading the repertoire: Progress in adaptive immune receptor analysis using machine learning
Timothy J O'Donnell, Chakravarthi Kanduri, Giulio Isacchini, et al.
Cell Systems (2024) Vol. 15, Iss. 12, pp. 1168-1189
Open Access | Times Cited: 2
Timothy J O'Donnell, Chakravarthi Kanduri, Giulio Isacchini, et al.
Cell Systems (2024) Vol. 15, Iss. 12, pp. 1168-1189
Open Access | Times Cited: 2
BATMAN: Improved T cell receptor cross-reactivity prediction benchmarked on a comprehensive mutational scan database
Amitava Banerjee, David Pattinson, Cornelia L. Wincek, et al.
bioRxiv (Cold Spring Harbor Laboratory) (2024)
Open Access | Times Cited: 2
Amitava Banerjee, David Pattinson, Cornelia L. Wincek, et al.
bioRxiv (Cold Spring Harbor Laboratory) (2024)
Open Access | Times Cited: 2
Supervised fine-tuning of pre-trained antibody language models improves antigen specificity prediction
Meng Wang, Jonathan Patsenker, Henry Li, et al.
bioRxiv (Cold Spring Harbor Laboratory) (2024)
Open Access | Times Cited: 2
Meng Wang, Jonathan Patsenker, Henry Li, et al.
bioRxiv (Cold Spring Harbor Laboratory) (2024)
Open Access | Times Cited: 2
Epitope-anchored contrastive transfer learning for paired CD8+ T cell receptor–antigen recognition
Yumeng Zhang, Zhikang Wang, Yunzhe Jiang, et al.
Nature Machine Intelligence (2024) Vol. 6, Iss. 11, pp. 1344-1358
Closed Access | Times Cited: 2
Yumeng Zhang, Zhikang Wang, Yunzhe Jiang, et al.
Nature Machine Intelligence (2024) Vol. 6, Iss. 11, pp. 1344-1358
Closed Access | Times Cited: 2
VitTCR: A deep learning method for peptide recognition prediction
Mengnan Jiang, Zilan Yu, Xun Lan
iScience (2024) Vol. 27, Iss. 5, pp. 109770-109770
Open Access | Times Cited: 1
Mengnan Jiang, Zilan Yu, Xun Lan
iScience (2024) Vol. 27, Iss. 5, pp. 109770-109770
Open Access | Times Cited: 1
Revealing the hidden sequence distribution of epitope-specific TCR repertoires and its influence on machine learning model performance
Sofie Gielis, Maria Chernigovskaya, Milena Pavlović, et al.
(2024)
Open Access | Times Cited: 1
Sofie Gielis, Maria Chernigovskaya, Milena Pavlović, et al.
(2024)
Open Access | Times Cited: 1
Reply to: The pitfalls of negative data bias for the T-cell epitope specificity challenge
Yicheng Gao, Yuli Gao, Kejing Dong, et al.
Nature Machine Intelligence (2023) Vol. 5, Iss. 10, pp. 1063-1065
Open Access | Times Cited: 3
Yicheng Gao, Yuli Gao, Kejing Dong, et al.
Nature Machine Intelligence (2023) Vol. 5, Iss. 10, pp. 1063-1065
Open Access | Times Cited: 3
TCR-H: Machine Learning Prediction of T-cell Receptor Epitope Binding on Unseen Datasets
Rajitha Rajeshwar T., Omar Demerdash, Jeremy C. Smith
bioRxiv (Cold Spring Harbor Laboratory) (2023)
Open Access | Times Cited: 3
Rajitha Rajeshwar T., Omar Demerdash, Jeremy C. Smith
bioRxiv (Cold Spring Harbor Laboratory) (2023)
Open Access | Times Cited: 3
Cracking the code of adaptive immunity: The role of computational tools
Kasi Vegesana, Paul G. Thomas
Cell Systems (2024) Vol. 15, Iss. 12, pp. 1156-1167
Closed Access
Kasi Vegesana, Paul G. Thomas
Cell Systems (2024) Vol. 15, Iss. 12, pp. 1156-1167
Closed Access