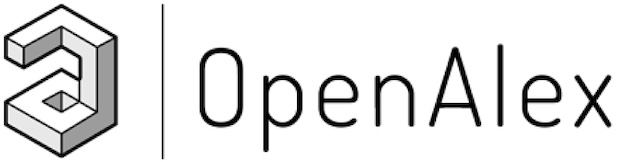
OpenAlex is a bibliographic catalogue of scientific papers, authors and institutions accessible in open access mode, named after the Library of Alexandria. It's citation coverage is excellent and I hope you will find utility in this listing of citing articles!
If you click the article title, you'll navigate to the article, as listed in CrossRef. If you click the Open Access links, you'll navigate to the "best Open Access location". Clicking the citation count will open this listing for that article. Lastly at the bottom of the page, you'll find basic pagination options.
Requested Article:
Deep learning-based defects detection of certain aero-engine blades and vanes with DDSC-YOLOv5s
Xubo Li, Wenqing Wang, Lihua Sun, et al.
Scientific Reports (2022) Vol. 12, Iss. 1
Open Access | Times Cited: 17
Xubo Li, Wenqing Wang, Lihua Sun, et al.
Scientific Reports (2022) Vol. 12, Iss. 1
Open Access | Times Cited: 17
Showing 17 citing articles:
Deep Learning and Computer Vision Techniques for Enhanced Quality Control in Manufacturing Processes
Md Raisul Islam, Md Zakir Hossain Zamil, Md. Eshmam Rayed, et al.
IEEE Access (2024) Vol. 12, pp. 121449-121479
Open Access | Times Cited: 8
Md Raisul Islam, Md Zakir Hossain Zamil, Md. Eshmam Rayed, et al.
IEEE Access (2024) Vol. 12, pp. 121449-121479
Open Access | Times Cited: 8
Damages Detection of Aeroengine Blades via Deep Learning Algorithms
Shuangbao Li, Jingyi Yu, Hao Wang
IEEE Transactions on Instrumentation and Measurement (2023) Vol. 72, pp. 1-11
Closed Access | Times Cited: 20
Shuangbao Li, Jingyi Yu, Hao Wang
IEEE Transactions on Instrumentation and Measurement (2023) Vol. 72, pp. 1-11
Closed Access | Times Cited: 20
Graphene nanoparticles as data generating digital materials in industry 4.0
Muhammad A. Ali, Muhammad Syahrizal Irfan, Tayyab Khan, et al.
Scientific Reports (2023) Vol. 13, Iss. 1
Open Access | Times Cited: 20
Muhammad A. Ali, Muhammad Syahrizal Irfan, Tayyab Khan, et al.
Scientific Reports (2023) Vol. 13, Iss. 1
Open Access | Times Cited: 20
A Deep-Learning-Based Approach for Aircraft Engine Defect Detection
Anurag Upadhyay, Jun Li, Stephen P. King, et al.
Machines (2023) Vol. 11, Iss. 2, pp. 192-192
Open Access | Times Cited: 16
Anurag Upadhyay, Jun Li, Stephen P. King, et al.
Machines (2023) Vol. 11, Iss. 2, pp. 192-192
Open Access | Times Cited: 16
Real-time detection of blade surface defects based on the improved RT-DETR
Dongbo Wu, Ruiwen Wu, Hui Wang, et al.
Journal of Intelligent Manufacturing (2025)
Closed Access
Dongbo Wu, Ruiwen Wu, Hui Wang, et al.
Journal of Intelligent Manufacturing (2025)
Closed Access
Aero-engine Blade Defect Detection: A Systematic Review of Deep Learning Models
Yusra Abdulrahman, M. A Mohammed Eltoum, Abdulla Ayyad, et al.
IEEE Access (2023), pp. 1-1
Open Access | Times Cited: 15
Yusra Abdulrahman, M. A Mohammed Eltoum, Abdulla Ayyad, et al.
IEEE Access (2023), pp. 1-1
Open Access | Times Cited: 15
Superpixel perception graph neural network for intelligent defect detection of aero-engine blade
Hongbing Shang, Qixiu Yang, Chuang Sun, et al.
Journal of Manufacturing Systems (2024) Vol. 77, pp. 112-126
Closed Access | Times Cited: 3
Hongbing Shang, Qixiu Yang, Chuang Sun, et al.
Journal of Manufacturing Systems (2024) Vol. 77, pp. 112-126
Closed Access | Times Cited: 3
Fringe Projection Profilometry for Three-Dimensional Measurement of Aerospace Blades
Ze Chen, Mulun Zhu, Chuanzhi Sun, et al.
Symmetry (2024) Vol. 16, Iss. 3, pp. 350-350
Open Access | Times Cited: 3
Ze Chen, Mulun Zhu, Chuanzhi Sun, et al.
Symmetry (2024) Vol. 16, Iss. 3, pp. 350-350
Open Access | Times Cited: 3
Automatic Defect Detection of Jet Engine Turbine and Compressor Blade Surface Coatings Using a Deep Learning-Based Algorithm
Md Hasib Zubayer, Chaoqun Zhang, Wen Liu, et al.
Coatings (2024) Vol. 14, Iss. 4, pp. 501-501
Open Access | Times Cited: 2
Md Hasib Zubayer, Chaoqun Zhang, Wen Liu, et al.
Coatings (2024) Vol. 14, Iss. 4, pp. 501-501
Open Access | Times Cited: 2
SAIT: Harnessing Sparse Annotations and Intrinsic Tasks for Semisupervised Aeroengine Defect Segmentation
Haochen Qi, Xiangwei Kong, Zhitong Liu, et al.
IEEE Transactions on Industrial Informatics (2024) Vol. 20, Iss. 8, pp. 10463-10472
Closed Access | Times Cited: 1
Haochen Qi, Xiangwei Kong, Zhitong Liu, et al.
IEEE Transactions on Industrial Informatics (2024) Vol. 20, Iss. 8, pp. 10463-10472
Closed Access | Times Cited: 1
AeroClick: An advanced single-click interactive framework for aeroengine defect segmentation
Haochen Qi, Xiangwei Kong, Zinan Wang, et al.
Expert Systems with Applications (2024) Vol. 257, pp. 125093-125093
Closed Access | Times Cited: 1
Haochen Qi, Xiangwei Kong, Zinan Wang, et al.
Expert Systems with Applications (2024) Vol. 257, pp. 125093-125093
Closed Access | Times Cited: 1
Multi-Object Detection Algorithm in Wind Turbine Nacelles Based on Improved YOLOX-Nano
Chunsheng Hu, Yong Zhao, Fangjuan Cheng, et al.
Energies (2023) Vol. 16, Iss. 3, pp. 1082-1082
Open Access | Times Cited: 4
Chunsheng Hu, Yong Zhao, Fangjuan Cheng, et al.
Energies (2023) Vol. 16, Iss. 3, pp. 1082-1082
Open Access | Times Cited: 4
Identification of Location and Geometry of Invisible Internal Defects in Structures using Deep Learning and Surface Deformation Field
Suman Timilsina, Seong Min Jang, Cheol Woo Jo, et al.
Advanced Intelligent Systems (2023) Vol. 5, Iss. 12
Open Access | Times Cited: 2
Suman Timilsina, Seong Min Jang, Cheol Woo Jo, et al.
Advanced Intelligent Systems (2023) Vol. 5, Iss. 12
Open Access | Times Cited: 2
MobGSim-YOLO: Mobile Device Terminal-Based Crack Hole Detection Model for Aero-Engine Blades
Xinyao Hou, Hao Zeng, Lu Jia, et al.
Aerospace (2024) Vol. 11, Iss. 8, pp. 676-676
Open Access
Xinyao Hou, Hao Zeng, Lu Jia, et al.
Aerospace (2024) Vol. 11, Iss. 8, pp. 676-676
Open Access
Intelligent Texture Feature-Based Defects Classification of Aircraft Engine Blades
Soham Joshi, Animesh Kumar, Mokshit Lodha, et al.
Lecture notes in networks and systems (2024), pp. 234-242
Closed Access
Soham Joshi, Animesh Kumar, Mokshit Lodha, et al.
Lecture notes in networks and systems (2024), pp. 234-242
Closed Access
MT-CrackNet:A multi-task deep learning framework for automatic in-situ fatigue micro-crack detection and quantification
Xiangyun Long, Hongyu Ji, Jinkang Liu, et al.
International Journal of Fatigue (2024), pp. 108667-108667
Closed Access
Xiangyun Long, Hongyu Ji, Jinkang Liu, et al.
International Journal of Fatigue (2024), pp. 108667-108667
Closed Access
Revolutionizing Military Surveillance: Advanced Deep Learning Techniques for Aircraft Detection
Patchigolla Sampath, Shaik Haseeb, Are Jaideep, et al.
(2023) Vol. 10, pp. 12-19
Closed Access | Times Cited: 1
Patchigolla Sampath, Shaik Haseeb, Are Jaideep, et al.
(2023) Vol. 10, pp. 12-19
Closed Access | Times Cited: 1