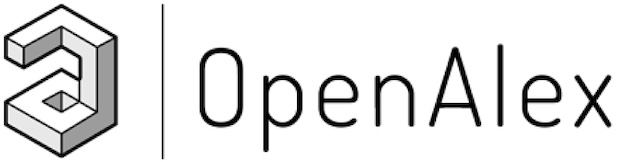
OpenAlex is a bibliographic catalogue of scientific papers, authors and institutions accessible in open access mode, named after the Library of Alexandria. It's citation coverage is excellent and I hope you will find utility in this listing of citing articles!
If you click the article title, you'll navigate to the article, as listed in CrossRef. If you click the Open Access links, you'll navigate to the "best Open Access location". Clicking the citation count will open this listing for that article. Lastly at the bottom of the page, you'll find basic pagination options.
Requested Article:
Ultra-fast interpretable machine-learning potentials
Stephen Xie, Matthias Rupp, Richard G. Hennig
npj Computational Materials (2023) Vol. 9, Iss. 1
Open Access | Times Cited: 24
Stephen Xie, Matthias Rupp, Richard G. Hennig
npj Computational Materials (2023) Vol. 9, Iss. 1
Open Access | Times Cited: 24
Showing 24 citing articles:
Machine learning heralding a new development phase in molecular dynamics simulations
Eva Prašnikar, Martin Ljubič, Andrej Perdih, et al.
Artificial Intelligence Review (2024) Vol. 57, Iss. 4
Open Access | Times Cited: 14
Eva Prašnikar, Martin Ljubič, Andrej Perdih, et al.
Artificial Intelligence Review (2024) Vol. 57, Iss. 4
Open Access | Times Cited: 14
Accelerating the prediction of inorganic surfaces with machine learning interatomic potentials
Kyle Noordhoek, Christopher J. Bartel
Nanoscale (2024) Vol. 16, Iss. 13, pp. 6365-6382
Open Access | Times Cited: 7
Kyle Noordhoek, Christopher J. Bartel
Nanoscale (2024) Vol. 16, Iss. 13, pp. 6365-6382
Open Access | Times Cited: 7
Performance Assessment of Universal Machine Learning Interatomic Potentials: Challenges and Directions for Materials’ Surfaces
Bruno Focassio, Luis Paulo Mezzina Freitas, Gabriel R. Schleder
ACS Applied Materials & Interfaces (2024)
Open Access | Times Cited: 6
Bruno Focassio, Luis Paulo Mezzina Freitas, Gabriel R. Schleder
ACS Applied Materials & Interfaces (2024)
Open Access | Times Cited: 6
Exploring parameter dependence of atomic minima with implicit differentiation
Ivan Maliyov, Petr Grigorev, Thomas D. Swinburne
npj Computational Materials (2025) Vol. 11, Iss. 1
Open Access
Ivan Maliyov, Petr Grigorev, Thomas D. Swinburne
npj Computational Materials (2025) Vol. 11, Iss. 1
Open Access
Tracking Li atoms in real-time with ultra-fast NMR simulations
Angela F. Harper, Tabea Huss, S. Kocher, et al.
(2024)
Open Access | Times Cited: 3
Angela F. Harper, Tabea Huss, S. Kocher, et al.
(2024)
Open Access | Times Cited: 3
Machine learned interatomic potentials for ternary carbides trained on the AFLOW database
Josiah Roberts, Biswas Rijal, Simon Divilov, et al.
npj Computational Materials (2024) Vol. 10, Iss. 1
Open Access | Times Cited: 3
Josiah Roberts, Biswas Rijal, Simon Divilov, et al.
npj Computational Materials (2024) Vol. 10, Iss. 1
Open Access | Times Cited: 3
Recent Advances in Machine Learning‐Assisted Multiscale Design of Energy Materials
Bohayra Mortazavi
Advanced Energy Materials (2024)
Open Access | Times Cited: 3
Bohayra Mortazavi
Advanced Energy Materials (2024)
Open Access | Times Cited: 3
High-Throughput Predictions of Accurate Enthalpies of Formation for Larger Molecules Utilizing the Bond Difference Correction Method
Huajie Xu, Bo Wang, Lingxian Liao, et al.
The Journal of Physical Chemistry Letters (2024) Vol. 15, Iss. 4, pp. 998-1005
Closed Access | Times Cited: 2
Huajie Xu, Bo Wang, Lingxian Liao, et al.
The Journal of Physical Chemistry Letters (2024) Vol. 15, Iss. 4, pp. 998-1005
Closed Access | Times Cited: 2
Machine Learning, Density Functional Theory, and Experiments to Understand the Photocatalytic Reduction of CO2 on CuPt/TiO2
Vaidish Sumaria, Takat B. Rawal, Young Feng Li, et al.
The Journal of Physical Chemistry C (2024) Vol. 128, Iss. 34, pp. 14247-14258
Closed Access | Times Cited: 2
Vaidish Sumaria, Takat B. Rawal, Young Feng Li, et al.
The Journal of Physical Chemistry C (2024) Vol. 128, Iss. 34, pp. 14247-14258
Closed Access | Times Cited: 2
DFT-Based Permutationally Invariant Polynomial Potentials Capture the Twists and Turns of C14H30
Chen Qu, Paul L. Houston, Thomas C. Allison, et al.
Journal of Chemical Theory and Computation (2024) Vol. 20, Iss. 21, pp. 9339-9353
Open Access | Times Cited: 2
Chen Qu, Paul L. Houston, Thomas C. Allison, et al.
Journal of Chemical Theory and Computation (2024) Vol. 20, Iss. 21, pp. 9339-9353
Open Access | Times Cited: 2
Delocalization error poisons the density-functional many-body expansion
Dustin Broderick, John M. Herbert
Chemical Science (2024) Vol. 15, Iss. 47, pp. 19893-19906
Open Access | Times Cited: 2
Dustin Broderick, John M. Herbert
Chemical Science (2024) Vol. 15, Iss. 47, pp. 19893-19906
Open Access | Times Cited: 2
A Genetic Algorithm Trained Machine-Learned Interatomic Potential for the Silicon–Carbon System
Michael MacIsaac, Salil Bavdekar, Douglas E. Spearot, et al.
The Journal of Physical Chemistry C (2024)
Open Access | Times Cited: 1
Michael MacIsaac, Salil Bavdekar, Douglas E. Spearot, et al.
The Journal of Physical Chemistry C (2024)
Open Access | Times Cited: 1
High-Throughput Screening of Li Solid-State Electrolytes with Bond Valence Methods and Machine Learning
Stephen Xie, Shreyas Honrao, John W. Lawson
Chemistry of Materials (2024) Vol. 36, Iss. 19, pp. 9320-9329
Closed Access | Times Cited: 1
Stephen Xie, Shreyas Honrao, John W. Lawson
Chemistry of Materials (2024) Vol. 36, Iss. 19, pp. 9320-9329
Closed Access | Times Cited: 1
Rediscovering the Particle-in-a-Box: Machine Learning Regression Analysis for Hypothesis Generation in Physical Chemistry Lab
Elizabeth S. Thrall, Fernando Martinez Lopez, Thomas Egg, et al.
Journal of Chemical Education (2023) Vol. 100, Iss. 12, pp. 4933-4940
Closed Access | Times Cited: 2
Elizabeth S. Thrall, Fernando Martinez Lopez, Thomas Egg, et al.
Journal of Chemical Education (2023) Vol. 100, Iss. 12, pp. 4933-4940
Closed Access | Times Cited: 2
Machine Learned Interatomic Potentials for Ternary Carbides trained on the AFLOW Database
Eva Zurek, Josiah Roberts, Biswas Rijal, et al.
Research Square (Research Square) (2024)
Open Access
Eva Zurek, Josiah Roberts, Biswas Rijal, et al.
Research Square (Research Square) (2024)
Open Access
Polymers Simulation using Machine Learning Interatomic Potentials
Teng Long, Li Jia, Chenlu Wang, et al.
Polymer (2024) Vol. 308, pp. 127416-127416
Closed Access
Teng Long, Li Jia, Chenlu Wang, et al.
Polymer (2024) Vol. 308, pp. 127416-127416
Closed Access
Thermodynamic integration by statistically sampling ensembles of dynamically unstable solids without molecular dynamics
Junsoo Park, Zhigang Wu, John W. Lawson
Physical review. B./Physical review. B (2024) Vol. 110, Iss. 14
Closed Access
Junsoo Park, Zhigang Wu, John W. Lawson
Physical review. B./Physical review. B (2024) Vol. 110, Iss. 14
Closed Access
Diamond under extremes
Alex C. Li, Boya Li, Felipe González‐Cataldo, et al.
Materials Science and Engineering R Reports (2024) Vol. 161, pp. 100857-100857
Closed Access
Alex C. Li, Boya Li, Felipe González‐Cataldo, et al.
Materials Science and Engineering R Reports (2024) Vol. 161, pp. 100857-100857
Closed Access
Generation and correction of machine learning interatomic potential for simulation of liquid metal corrosion with near experimental accuracy: a study for iron corrosion in liquid lead
Sujin Bak, Takuji Oda
Corrosion Science (2024), pp. 112541-112541
Closed Access
Sujin Bak, Takuji Oda
Corrosion Science (2024), pp. 112541-112541
Closed Access
Uncertainty Based Machine Learning-DFT Hybrid Framework for Accelerating Geometry Optimization
Akksay Singh, Jiaqi Wang, Graeme Henkelman, et al.
Journal of Chemical Theory and Computation (2024) Vol. 20, Iss. 22, pp. 10022-10033
Closed Access
Akksay Singh, Jiaqi Wang, Graeme Henkelman, et al.
Journal of Chemical Theory and Computation (2024) Vol. 20, Iss. 22, pp. 10022-10033
Closed Access
A data-driven method for optimization of classical interatomic potentials
Benjamin A. Jasperson, Harley T. Johnson
MRS Advances (2024) Vol. 9, Iss. 11, pp. 863-869
Closed Access
Benjamin A. Jasperson, Harley T. Johnson
MRS Advances (2024) Vol. 9, Iss. 11, pp. 863-869
Closed Access
Tracking Li atoms in real-time with ultra-fast NMR simulations
Angela F. Harper, Tabea Huss, S. Kocher, et al.
Faraday Discussions (2024)
Open Access
Angela F. Harper, Tabea Huss, S. Kocher, et al.
Faraday Discussions (2024)
Open Access
Active Learning of Boltzmann Samplers and Potential Energies with Quantum Mechanical Accuracy
Ana Molina-Taborda, Pilar Cossio, Olga Lopez‐Acevedo, et al.
Journal of Chemical Theory and Computation (2024) Vol. 20, Iss. 20, pp. 8833-8843
Open Access
Ana Molina-Taborda, Pilar Cossio, Olga Lopez‐Acevedo, et al.
Journal of Chemical Theory and Computation (2024) Vol. 20, Iss. 20, pp. 8833-8843
Open Access
Rediscovering the particle in a box: Machine learning regression analysis for hypothesis generation in physical chemistry lab
Elizabeth S. Thrall, Fernando Martinez Lopez, Thomas Egg, et al.
(2023)
Open Access | Times Cited: 1
Elizabeth S. Thrall, Fernando Martinez Lopez, Thomas Egg, et al.
(2023)
Open Access | Times Cited: 1