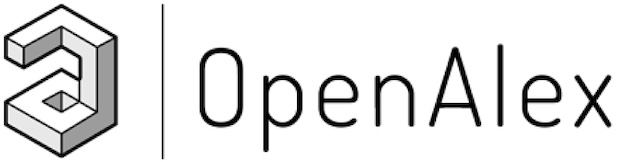
OpenAlex is a bibliographic catalogue of scientific papers, authors and institutions accessible in open access mode, named after the Library of Alexandria. It's citation coverage is excellent and I hope you will find utility in this listing of citing articles!
If you click the article title, you'll navigate to the article, as listed in CrossRef. If you click the Open Access links, you'll navigate to the "best Open Access location". Clicking the citation count will open this listing for that article. Lastly at the bottom of the page, you'll find basic pagination options.
Requested Article:
E(3)-equivariant graph neural networks for data-efficient and accurate interatomic potentials
Simon Batzner, Albert Musaelian, Lixin Sun, et al.
Nature Communications (2022) Vol. 13, Iss. 1
Open Access | Times Cited: 802
Simon Batzner, Albert Musaelian, Lixin Sun, et al.
Nature Communications (2022) Vol. 13, Iss. 1
Open Access | Times Cited: 802
Showing 1-25 of 802 citing articles:
Scaling deep learning for materials discovery
Amil Merchant, Simon Batzner, Samuel S. Schoenholz, et al.
Nature (2023) Vol. 624, Iss. 7990, pp. 80-85
Open Access | Times Cited: 370
Amil Merchant, Simon Batzner, Samuel S. Schoenholz, et al.
Nature (2023) Vol. 624, Iss. 7990, pp. 80-85
Open Access | Times Cited: 370
Graph neural networks for materials science and chemistry
Patrick Reiser, Marlen Neubert, André Eberhard, et al.
Communications Materials (2022) Vol. 3, Iss. 1
Open Access | Times Cited: 299
Patrick Reiser, Marlen Neubert, André Eberhard, et al.
Communications Materials (2022) Vol. 3, Iss. 1
Open Access | Times Cited: 299
Learning local equivariant representations for large-scale atomistic dynamics
Albert Musaelian, Simon Batzner, Anders Johansson, et al.
Nature Communications (2023) Vol. 14, Iss. 1
Open Access | Times Cited: 275
Albert Musaelian, Simon Batzner, Anders Johansson, et al.
Nature Communications (2023) Vol. 14, Iss. 1
Open Access | Times Cited: 275
SpookyNet: Learning force fields with electronic degrees of freedom and nonlocal effects
Oliver T. Unke, Stefan Chmiela, Michael Gastegger, et al.
Nature Communications (2021) Vol. 12, Iss. 1
Open Access | Times Cited: 189
Oliver T. Unke, Stefan Chmiela, Michael Gastegger, et al.
Nature Communications (2021) Vol. 12, Iss. 1
Open Access | Times Cited: 189
DeePMD-kit v2: A software package for deep potential models
Jinzhe Zeng, Duo Zhang, Denghui Lu, et al.
The Journal of Chemical Physics (2023) Vol. 159, Iss. 5
Open Access | Times Cited: 188
Jinzhe Zeng, Duo Zhang, Denghui Lu, et al.
The Journal of Chemical Physics (2023) Vol. 159, Iss. 5
Open Access | Times Cited: 188
CHGNet as a pretrained universal neural network potential for charge-informed atomistic modelling
Bowen Deng, Peichen Zhong, KyuJung Jun, et al.
Nature Machine Intelligence (2023) Vol. 5, Iss. 9, pp. 1031-1041
Open Access | Times Cited: 184
Bowen Deng, Peichen Zhong, KyuJung Jun, et al.
Nature Machine Intelligence (2023) Vol. 5, Iss. 9, pp. 1031-1041
Open Access | Times Cited: 184
Inverse design of 3d molecular structures with conditional generative neural networks
Niklas W. A. Gebauer, Michael Gastegger, Stefaan S. P. Hessmann, et al.
Nature Communications (2022) Vol. 13, Iss. 1
Open Access | Times Cited: 121
Niklas W. A. Gebauer, Michael Gastegger, Stefaan S. P. Hessmann, et al.
Nature Communications (2022) Vol. 13, Iss. 1
Open Access | Times Cited: 121
The Open Catalyst 2022 (OC22) Dataset and Challenges for Oxide Electrocatalysts
Richard Tran, Janice Lan, Muhammed Shuaibi, et al.
ACS Catalysis (2023) Vol. 13, Iss. 5, pp. 3066-3084
Open Access | Times Cited: 112
Richard Tran, Janice Lan, Muhammed Shuaibi, et al.
ACS Catalysis (2023) Vol. 13, Iss. 5, pp. 3066-3084
Open Access | Times Cited: 112
A Comprehensive Survey on Deep Graph Representation Learning
Wei Ju, Zheng Fang, Yiyang Gu, et al.
Neural Networks (2024) Vol. 173, pp. 106207-106207
Open Access | Times Cited: 108
Wei Ju, Zheng Fang, Yiyang Gu, et al.
Neural Networks (2024) Vol. 173, pp. 106207-106207
Open Access | Times Cited: 108
14 examples of how LLMs can transform materials science and chemistry: a reflection on a large language model hackathon
Kevin Maik Jablonka, Qianxiang Ai, Alexander Al-Feghali, et al.
Digital Discovery (2023) Vol. 2, Iss. 5, pp. 1233-1250
Open Access | Times Cited: 106
Kevin Maik Jablonka, Qianxiang Ai, Alexander Al-Feghali, et al.
Digital Discovery (2023) Vol. 2, Iss. 5, pp. 1233-1250
Open Access | Times Cited: 106
Structure-based drug design with geometric deep learning
Clemens Isert, Kenneth Atz, Gisbert Schneider
Current Opinion in Structural Biology (2023) Vol. 79, pp. 102548-102548
Open Access | Times Cited: 91
Clemens Isert, Kenneth Atz, Gisbert Schneider
Current Opinion in Structural Biology (2023) Vol. 79, pp. 102548-102548
Open Access | Times Cited: 91
Accurate global machine learning force fields for molecules with hundreds of atoms
Stefan Chmiela, Valentín Vassilev-Galindo, Oliver T. Unke, et al.
Science Advances (2023) Vol. 9, Iss. 2
Open Access | Times Cited: 80
Stefan Chmiela, Valentín Vassilev-Galindo, Oliver T. Unke, et al.
Science Advances (2023) Vol. 9, Iss. 2
Open Access | Times Cited: 80
NewtonNet: a Newtonian message passing network for deep learning of interatomic potentials and forces
Mojtaba Haghighatlari, Jie Li, Xingyi Guan, et al.
Digital Discovery (2022) Vol. 1, Iss. 3, pp. 333-343
Open Access | Times Cited: 76
Mojtaba Haghighatlari, Jie Li, Xingyi Guan, et al.
Digital Discovery (2022) Vol. 1, Iss. 3, pp. 333-343
Open Access | Times Cited: 76
Machine learning potentials for metal-organic frameworks using an incremental learning approach
Sander Vandenhaute, Maarten Cools‐Ceuppens, Simon DeKeyser, et al.
npj Computational Materials (2023) Vol. 9, Iss. 1
Open Access | Times Cited: 72
Sander Vandenhaute, Maarten Cools‐Ceuppens, Simon DeKeyser, et al.
npj Computational Materials (2023) Vol. 9, Iss. 1
Open Access | Times Cited: 72
PeSTo: parameter-free geometric deep learning for accurate prediction of protein binding interfaces
Lucien F. Krapp, Luciano A. Abriata, Fabio Cortés Rodríguez, et al.
Nature Communications (2023) Vol. 14, Iss. 1
Open Access | Times Cited: 72
Lucien F. Krapp, Luciano A. Abriata, Fabio Cortés Rodríguez, et al.
Nature Communications (2023) Vol. 14, Iss. 1
Open Access | Times Cited: 72
TeaNet: Universal neural network interatomic potential inspired by iterative electronic relaxations
So Takamoto, Satoshi IZUMI, Ju Li
Computational Materials Science (2022) Vol. 207, pp. 111280-111280
Open Access | Times Cited: 68
So Takamoto, Satoshi IZUMI, Ju Li
Computational Materials Science (2022) Vol. 207, pp. 111280-111280
Open Access | Times Cited: 68
OpenMM 8: Molecular Dynamics Simulation with Machine Learning Potentials
Peter Eastman, Raimondas Galvelis, Raúl P. Peláez, et al.
The Journal of Physical Chemistry B (2023) Vol. 128, Iss. 1, pp. 109-116
Open Access | Times Cited: 67
Peter Eastman, Raimondas Galvelis, Raúl P. Peláez, et al.
The Journal of Physical Chemistry B (2023) Vol. 128, Iss. 1, pp. 109-116
Open Access | Times Cited: 67
Machine Learning-Guided Protein Engineering
Petr Kouba, Pavel Kohout, Faraneh Haddadi, et al.
ACS Catalysis (2023) Vol. 13, Iss. 21, pp. 13863-13895
Open Access | Times Cited: 66
Petr Kouba, Pavel Kohout, Faraneh Haddadi, et al.
ACS Catalysis (2023) Vol. 13, Iss. 21, pp. 13863-13895
Open Access | Times Cited: 66
SPICE, A Dataset of Drug-like Molecules and Peptides for Training Machine Learning Potentials
Peter Eastman, Pavan Kumar Behara, David Dotson, et al.
Scientific Data (2023) Vol. 10, Iss. 1
Open Access | Times Cited: 62
Peter Eastman, Pavan Kumar Behara, David Dotson, et al.
Scientific Data (2023) Vol. 10, Iss. 1
Open Access | Times Cited: 62
How to validate machine-learned interatomic potentials
Joe D. Morrow, John L. A. Gardner, Volker L. Deringer
The Journal of Chemical Physics (2023) Vol. 158, Iss. 12
Open Access | Times Cited: 60
Joe D. Morrow, John L. A. Gardner, Volker L. Deringer
The Journal of Chemical Physics (2023) Vol. 158, Iss. 12
Open Access | Times Cited: 60
Deep language models for interpretative and predictive materials science
Yiwen Hu, Markus J. Buehler
APL Machine Learning (2023) Vol. 1, Iss. 1
Open Access | Times Cited: 57
Yiwen Hu, Markus J. Buehler
APL Machine Learning (2023) Vol. 1, Iss. 1
Open Access | Times Cited: 57
Uncertainty-driven dynamics for active learning of interatomic potentials
Maksim Kulichenko, Kipton Barros, Nicholas Lubbers, et al.
Nature Computational Science (2023) Vol. 3, Iss. 3, pp. 230-239
Open Access | Times Cited: 57
Maksim Kulichenko, Kipton Barros, Nicholas Lubbers, et al.
Nature Computational Science (2023) Vol. 3, Iss. 3, pp. 230-239
Open Access | Times Cited: 57
Unifying the design space and optimizing linear and nonlinear truss metamaterials by generative modeling
Li Zheng, Konstantinos Karapiperis, Siddhant Kumar, et al.
Nature Communications (2023) Vol. 14, Iss. 1
Open Access | Times Cited: 55
Li Zheng, Konstantinos Karapiperis, Siddhant Kumar, et al.
Nature Communications (2023) Vol. 14, Iss. 1
Open Access | Times Cited: 55
A “short blanket” dilemma for a state-of-the-art neural network potential for water: Reproducing experimental properties or the physics of the underlying many-body interactions?
Yaoguang Zhai, Alessandro Caruso, Sigbjørn Løland Bore, et al.
The Journal of Chemical Physics (2023) Vol. 158, Iss. 8
Open Access | Times Cited: 52
Yaoguang Zhai, Alessandro Caruso, Sigbjørn Løland Bore, et al.
The Journal of Chemical Physics (2023) Vol. 158, Iss. 8
Open Access | Times Cited: 52