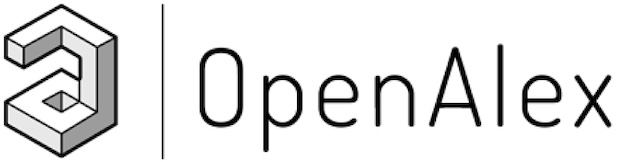
OpenAlex is a bibliographic catalogue of scientific papers, authors and institutions accessible in open access mode, named after the Library of Alexandria. It's citation coverage is excellent and I hope you will find utility in this listing of citing articles!
If you click the article title, you'll navigate to the article, as listed in CrossRef. If you click the Open Access links, you'll navigate to the "best Open Access location". Clicking the citation count will open this listing for that article. Lastly at the bottom of the page, you'll find basic pagination options.
Requested Article:
Single-cell RNA-seq denoising using a deep count autoencoder
Gökçen Eraslan, Lukas M. Simon, Maria Mircea, et al.
Nature Communications (2019) Vol. 10, Iss. 1
Open Access | Times Cited: 843
Gökçen Eraslan, Lukas M. Simon, Maria Mircea, et al.
Nature Communications (2019) Vol. 10, Iss. 1
Open Access | Times Cited: 843
Showing 1-25 of 843 citing articles:
Normalization and variance stabilization of single-cell RNA-seq data using regularized negative binomial regression
Christoph Hafemeister, Rahul Satija
Genome biology (2019) Vol. 20, Iss. 1
Open Access | Times Cited: 3337
Christoph Hafemeister, Rahul Satija
Genome biology (2019) Vol. 20, Iss. 1
Open Access | Times Cited: 3337
Deep generative modeling for single-cell transcriptomics
Romain Lopez, Jeffrey Regier, Michael B. Cole, et al.
Nature Methods (2018) Vol. 15, Iss. 12, pp. 1053-1058
Open Access | Times Cited: 1700
Romain Lopez, Jeffrey Regier, Michael B. Cole, et al.
Nature Methods (2018) Vol. 15, Iss. 12, pp. 1053-1058
Open Access | Times Cited: 1700
Current best practices in single‐cell RNA‐seq analysis: a tutorial
Malte D. Luecken, Fabian J. Theis
Molecular Systems Biology (2019) Vol. 15, Iss. 6
Open Access | Times Cited: 1672
Malte D. Luecken, Fabian J. Theis
Molecular Systems Biology (2019) Vol. 15, Iss. 6
Open Access | Times Cited: 1672
Eleven grand challenges in single-cell data science
David Lähnemann, Johannes Köster, Ewa Szczurek, et al.
Genome biology (2020) Vol. 21, Iss. 1
Open Access | Times Cited: 992
David Lähnemann, Johannes Köster, Ewa Szczurek, et al.
Genome biology (2020) Vol. 21, Iss. 1
Open Access | Times Cited: 992
Deep learning: new computational modelling techniques for genomics
Gökçen Eraslan, Žiga Avsec, Julien Gagneur, et al.
Nature Reviews Genetics (2019) Vol. 20, Iss. 7, pp. 389-403
Closed Access | Times Cited: 930
Gökçen Eraslan, Žiga Avsec, Julien Gagneur, et al.
Nature Reviews Genetics (2019) Vol. 20, Iss. 7, pp. 389-403
Closed Access | Times Cited: 930
Scientific discovery in the age of artificial intelligence
Hanchen Wang, Tianfan Fu, Yuanqi Du, et al.
Nature (2023) Vol. 620, Iss. 7972, pp. 47-60
Closed Access | Times Cited: 588
Hanchen Wang, Tianfan Fu, Yuanqi Du, et al.
Nature (2023) Vol. 620, Iss. 7972, pp. 47-60
Closed Access | Times Cited: 588
Statistics or biology: the zero-inflation controversy about scRNA-seq data
Ruochen Jiang, Tianyi Sun, Dongyuan Song, et al.
Genome biology (2022) Vol. 23, Iss. 1
Open Access | Times Cited: 425
Ruochen Jiang, Tianyi Sun, Dongyuan Song, et al.
Genome biology (2022) Vol. 23, Iss. 1
Open Access | Times Cited: 425
scGen predicts single-cell perturbation responses
Mohammad Lotfollahi, F. Alexander Wolf, Fabian J. Theis
Nature Methods (2019) Vol. 16, Iss. 8, pp. 715-721
Closed Access | Times Cited: 416
Mohammad Lotfollahi, F. Alexander Wolf, Fabian J. Theis
Nature Methods (2019) Vol. 16, Iss. 8, pp. 715-721
Closed Access | Times Cited: 416
Probabilistic harmonization and annotation of single‐cell transcriptomics data with deep generative models
Chenling Xu, Romain Lopez, Edouard Mehlman, et al.
Molecular Systems Biology (2021) Vol. 17, Iss. 1
Open Access | Times Cited: 362
Chenling Xu, Romain Lopez, Edouard Mehlman, et al.
Molecular Systems Biology (2021) Vol. 17, Iss. 1
Open Access | Times Cited: 362
Comparison and evaluation of statistical error models for scRNA-seq
Saket Choudhary, Rahul Satija
Genome biology (2022) Vol. 23, Iss. 1
Open Access | Times Cited: 315
Saket Choudhary, Rahul Satija
Genome biology (2022) Vol. 23, Iss. 1
Open Access | Times Cited: 315
Droplet scRNA-seq is not zero-inflated
Valentine Svensson
Nature Biotechnology (2020) Vol. 38, Iss. 2, pp. 147-150
Open Access | Times Cited: 300
Valentine Svensson
Nature Biotechnology (2020) Vol. 38, Iss. 2, pp. 147-150
Open Access | Times Cited: 300
Clustering single-cell RNA-seq data with a model-based deep learning approach
Tian Tian, Ji Wan, Qi Song, et al.
Nature Machine Intelligence (2019) Vol. 1, Iss. 4, pp. 191-198
Closed Access | Times Cited: 277
Tian Tian, Ji Wan, Qi Song, et al.
Nature Machine Intelligence (2019) Vol. 1, Iss. 4, pp. 191-198
Closed Access | Times Cited: 277
scGNN is a novel graph neural network framework for single-cell RNA-Seq analyses
Juexin Wang, Anjun Ma, Yuzhou Chang, et al.
Nature Communications (2021) Vol. 12, Iss. 1
Open Access | Times Cited: 270
Juexin Wang, Anjun Ma, Yuzhou Chang, et al.
Nature Communications (2021) Vol. 12, Iss. 1
Open Access | Times Cited: 270
Unsupervised removal of systematic background noise from droplet-based single-cell experiments using CellBender
Stephen J. Fleming, Mark Chaffin, Alessandro Arduini, et al.
Nature Methods (2023) Vol. 20, Iss. 9, pp. 1323-1335
Open Access | Times Cited: 268
Stephen J. Fleming, Mark Chaffin, Alessandro Arduini, et al.
Nature Methods (2023) Vol. 20, Iss. 9, pp. 1323-1335
Open Access | Times Cited: 268
Deep learning-based clustering approaches for bioinformatics
Md. Rezaul Karim, Oya Beyan, Achille Zappa, et al.
Briefings in Bioinformatics (2019) Vol. 22, Iss. 1, pp. 393-415
Open Access | Times Cited: 236
Md. Rezaul Karim, Oya Beyan, Achille Zappa, et al.
Briefings in Bioinformatics (2019) Vol. 22, Iss. 1, pp. 393-415
Open Access | Times Cited: 236
A systematic evaluation of single-cell RNA-sequencing imputation methods
Wenpin Hou, Zhicheng Ji, Hongkai Ji, et al.
Genome biology (2020) Vol. 21, Iss. 1
Open Access | Times Cited: 233
Wenpin Hou, Zhicheng Ji, Hongkai Ji, et al.
Genome biology (2020) Vol. 21, Iss. 1
Open Access | Times Cited: 233
DeepImpute: an accurate, fast, and scalable deep neural network method to impute single-cell RNA-seq data
Cédric Arisdakessian, Olivier Poirion, Breck Yunits, et al.
Genome biology (2019) Vol. 20, Iss. 1
Open Access | Times Cited: 232
Cédric Arisdakessian, Olivier Poirion, Breck Yunits, et al.
Genome biology (2019) Vol. 20, Iss. 1
Open Access | Times Cited: 232
Functional interpretation of single cell similarity maps
David DeTomaso, Matthew G. Jones, Meena Subramaniam, et al.
Nature Communications (2019) Vol. 10, Iss. 1
Open Access | Times Cited: 220
David DeTomaso, Matthew G. Jones, Meena Subramaniam, et al.
Nature Communications (2019) Vol. 10, Iss. 1
Open Access | Times Cited: 220
The epigenetic basis of cellular heterogeneity
Benjamin C. Carter, Keji Zhao
Nature Reviews Genetics (2020) Vol. 22, Iss. 4, pp. 235-250
Open Access | Times Cited: 220
Benjamin C. Carter, Keji Zhao
Nature Reviews Genetics (2020) Vol. 22, Iss. 4, pp. 235-250
Open Access | Times Cited: 220
Spatial components of molecular tissue biology
Giovanni Palla, David S. Fischer, Aviv Regev, et al.
Nature Biotechnology (2022) Vol. 40, Iss. 3, pp. 308-318
Closed Access | Times Cited: 218
Giovanni Palla, David S. Fischer, Aviv Regev, et al.
Nature Biotechnology (2022) Vol. 40, Iss. 3, pp. 308-318
Closed Access | Times Cited: 218
The Human Lung Cell Atlas: A High-Resolution Reference Map of the Human Lung in Health and Disease
Herbert B. Schiller, Daniel T. Montoro, Lukas M. Simon, et al.
American Journal of Respiratory Cell and Molecular Biology (2019) Vol. 61, Iss. 1, pp. 31-41
Open Access | Times Cited: 215
Herbert B. Schiller, Daniel T. Montoro, Lukas M. Simon, et al.
American Journal of Respiratory Cell and Molecular Biology (2019) Vol. 61, Iss. 1, pp. 31-41
Open Access | Times Cited: 215
The triumphs and limitations of computational methods for scRNA-seq
Peter V. Kharchenko
Nature Methods (2021) Vol. 18, Iss. 7, pp. 723-732
Closed Access | Times Cited: 214
Peter V. Kharchenko
Nature Methods (2021) Vol. 18, Iss. 7, pp. 723-732
Closed Access | Times Cited: 214
Normalization and variance stabilization of single-cell RNA-seq data using regularized negative binomial regression
Christoph Hafemeister, Rahul Satija
bioRxiv (Cold Spring Harbor Laboratory) (2019)
Open Access | Times Cited: 207
Christoph Hafemeister, Rahul Satija
bioRxiv (Cold Spring Harbor Laboratory) (2019)
Open Access | Times Cited: 207
Current progress and open challenges for applying deep learning across the biosciences
Nicolae Sapoval, Amirali Aghazadeh, Michael Nute, et al.
Nature Communications (2022) Vol. 13, Iss. 1
Open Access | Times Cited: 207
Nicolae Sapoval, Amirali Aghazadeh, Michael Nute, et al.
Nature Communications (2022) Vol. 13, Iss. 1
Open Access | Times Cited: 207
Concepts and limitations for learning developmental trajectories from single cell genomics
Sophie Tritschler, Maren Büttner, David S. Fischer, et al.
Development (2019) Vol. 146, Iss. 12
Open Access | Times Cited: 204
Sophie Tritschler, Maren Büttner, David S. Fischer, et al.
Development (2019) Vol. 146, Iss. 12
Open Access | Times Cited: 204