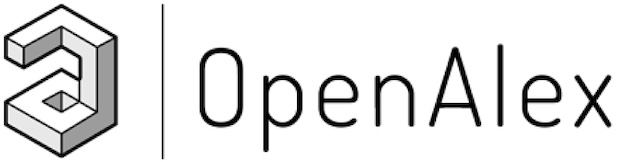
OpenAlex is a bibliographic catalogue of scientific papers, authors and institutions accessible in open access mode, named after the Library of Alexandria. It's citation coverage is excellent and I hope you will find utility in this listing of citing articles!
If you click the article title, you'll navigate to the article, as listed in CrossRef. If you click the Open Access links, you'll navigate to the "best Open Access location". Clicking the citation count will open this listing for that article. Lastly at the bottom of the page, you'll find basic pagination options.
Requested Article:
Universal fragment descriptors for predicting properties of inorganic crystals
Olexandr Isayev, Corey Oses, Cormac Toher, et al.
Nature Communications (2017) Vol. 8, Iss. 1
Open Access | Times Cited: 580
Olexandr Isayev, Corey Oses, Cormac Toher, et al.
Nature Communications (2017) Vol. 8, Iss. 1
Open Access | Times Cited: 580
Showing 1-25 of 580 citing articles:
Machine learning for molecular and materials science
Keith T. Butler, Daniel W. Davies, Hugh M. Cartwright, et al.
Nature (2018) Vol. 559, Iss. 7715, pp. 547-555
Open Access | Times Cited: 3463
Keith T. Butler, Daniel W. Davies, Hugh M. Cartwright, et al.
Nature (2018) Vol. 559, Iss. 7715, pp. 547-555
Open Access | Times Cited: 3463
Crystal Graph Convolutional Neural Networks for an Accurate and Interpretable Prediction of Material Properties
Tian Xie, Jeffrey C. Grossman
Physical Review Letters (2018) Vol. 120, Iss. 14
Open Access | Times Cited: 1911
Tian Xie, Jeffrey C. Grossman
Physical Review Letters (2018) Vol. 120, Iss. 14
Open Access | Times Cited: 1911
Recent advances and applications of machine learning in solid-state materials science
Jonathan Schmidt, Mário R. G. Marques, Silvana Botti, et al.
npj Computational Materials (2019) Vol. 5, Iss. 1
Open Access | Times Cited: 1894
Jonathan Schmidt, Mário R. G. Marques, Silvana Botti, et al.
npj Computational Materials (2019) Vol. 5, Iss. 1
Open Access | Times Cited: 1894
ANI-1: an extensible neural network potential with DFT accuracy at force field computational cost
Justin S. Smith, Olexandr Isayev, Adrián E. Roitberg
Chemical Science (2017) Vol. 8, Iss. 4, pp. 3192-3203
Open Access | Times Cited: 1699
Justin S. Smith, Olexandr Isayev, Adrián E. Roitberg
Chemical Science (2017) Vol. 8, Iss. 4, pp. 3192-3203
Open Access | Times Cited: 1699
Graph Networks as a Universal Machine Learning Framework for Molecules and Crystals
Chi Chen, Weike Ye, Yunxing Zuo, et al.
Chemistry of Materials (2019) Vol. 31, Iss. 9, pp. 3564-3572
Open Access | Times Cited: 1047
Chi Chen, Weike Ye, Yunxing Zuo, et al.
Chemistry of Materials (2019) Vol. 31, Iss. 9, pp. 3564-3572
Open Access | Times Cited: 1047
Materials discovery and design using machine learning
Yue Liu, Tianlu Zhao, Wangwei Ju, et al.
Journal of Materiomics (2017) Vol. 3, Iss. 3, pp. 159-177
Open Access | Times Cited: 1019
Yue Liu, Tianlu Zhao, Wangwei Ju, et al.
Journal of Materiomics (2017) Vol. 3, Iss. 3, pp. 159-177
Open Access | Times Cited: 1019
SchNet – A deep learning architecture for molecules and materials
Kristof T. Schütt, Huziel E. Sauceda, Pieter-Jan Kindermans, et al.
The Journal of Chemical Physics (2018) Vol. 148, Iss. 24
Open Access | Times Cited: 966
Kristof T. Schütt, Huziel E. Sauceda, Pieter-Jan Kindermans, et al.
The Journal of Chemical Physics (2018) Vol. 148, Iss. 24
Open Access | Times Cited: 966
Matminer: An open source toolkit for materials data mining
Logan Ward, Alexander Dunn, Alireza Faghaninia, et al.
Computational Materials Science (2018) Vol. 152, pp. 60-69
Open Access | Times Cited: 712
Logan Ward, Alexander Dunn, Alireza Faghaninia, et al.
Computational Materials Science (2018) Vol. 152, pp. 60-69
Open Access | Times Cited: 712
Machine Learning Interatomic Potentials as Emerging Tools for Materials Science
Volker L. Deringer, A. Miguel, Gábor Cśanyi
Advanced Materials (2019) Vol. 31, Iss. 46
Open Access | Times Cited: 647
Volker L. Deringer, A. Miguel, Gábor Cśanyi
Advanced Materials (2019) Vol. 31, Iss. 46
Open Access | Times Cited: 647
From DFT to machine learning: recent approaches to materials science–a review
Gabriel R. Schleder, A. C. M. Padilha, Carlos Mera Acosta, et al.
Journal of Physics Materials (2019) Vol. 2, Iss. 3, pp. 032001-032001
Open Access | Times Cited: 646
Gabriel R. Schleder, A. C. M. Padilha, Carlos Mera Acosta, et al.
Journal of Physics Materials (2019) Vol. 2, Iss. 3, pp. 032001-032001
Open Access | Times Cited: 646
QSAR without borders
Eugene Muratov, Jürgen Bajorath, Robert P. Sheridan, et al.
Chemical Society Reviews (2020) Vol. 49, Iss. 11, pp. 3525-3564
Open Access | Times Cited: 617
Eugene Muratov, Jürgen Bajorath, Robert P. Sheridan, et al.
Chemical Society Reviews (2020) Vol. 49, Iss. 11, pp. 3525-3564
Open Access | Times Cited: 617
DScribe: Library of descriptors for machine learning in materials science
Lauri Himanen, Marc O. J. Jäger, Eiaki V. Morooka, et al.
Computer Physics Communications (2019) Vol. 247, pp. 106949-106949
Open Access | Times Cited: 605
Lauri Himanen, Marc O. J. Jäger, Eiaki V. Morooka, et al.
Computer Physics Communications (2019) Vol. 247, pp. 106949-106949
Open Access | Times Cited: 605
Recent advances and applications of deep learning methods in materials science
Kamal Choudhary, Brian DeCost, Chi Chen, et al.
npj Computational Materials (2022) Vol. 8, Iss. 1
Open Access | Times Cited: 592
Kamal Choudhary, Brian DeCost, Chi Chen, et al.
npj Computational Materials (2022) Vol. 8, Iss. 1
Open Access | Times Cited: 592
Accelerated discovery of stable lead-free hybrid organic-inorganic perovskites via machine learning
Shuaihua Lu, Qionghua Zhou, Yixin Ouyang, et al.
Nature Communications (2018) Vol. 9, Iss. 1
Open Access | Times Cited: 587
Shuaihua Lu, Qionghua Zhou, Yixin Ouyang, et al.
Nature Communications (2018) Vol. 9, Iss. 1
Open Access | Times Cited: 587
Oxide perovskites, double perovskites and derivatives for electrocatalysis, photocatalysis, and photovoltaics
Wan‐Jian Yin, Baicheng Weng, Jie Ge, et al.
Energy & Environmental Science (2018) Vol. 12, Iss. 2, pp. 442-462
Closed Access | Times Cited: 581
Wan‐Jian Yin, Baicheng Weng, Jie Ge, et al.
Energy & Environmental Science (2018) Vol. 12, Iss. 2, pp. 442-462
Closed Access | Times Cited: 581
Structure prediction drives materials discovery
Artem R. Oganov, Chris J. Pickard, Qiang Zhu, et al.
Nature Reviews Materials (2019) Vol. 4, Iss. 5, pp. 331-348
Closed Access | Times Cited: 558
Artem R. Oganov, Chris J. Pickard, Qiang Zhu, et al.
Nature Reviews Materials (2019) Vol. 4, Iss. 5, pp. 331-348
Closed Access | Times Cited: 558
Approaching coupled cluster accuracy with a general-purpose neural network potential through transfer learning
Justin S. Smith, Benjamin Nebgen, R.I. Zubatyuk, et al.
Nature Communications (2019) Vol. 10, Iss. 1
Open Access | Times Cited: 538
Justin S. Smith, Benjamin Nebgen, R.I. Zubatyuk, et al.
Nature Communications (2019) Vol. 10, Iss. 1
Open Access | Times Cited: 538
The TensorMol-0.1 model chemistry: a neural network augmented with long-range physics
Kun Yao, John E. Herr, David W. Toth, et al.
Chemical Science (2018) Vol. 9, Iss. 8, pp. 2261-2269
Open Access | Times Cited: 465
Kun Yao, John E. Herr, David W. Toth, et al.
Chemical Science (2018) Vol. 9, Iss. 8, pp. 2261-2269
Open Access | Times Cited: 465
Big-Data Science in Porous Materials: Materials Genomics and Machine Learning
Kevin Maik Jablonka, Daniele Ongari, Seyed Mohamad Moosavi, et al.
Chemical Reviews (2020) Vol. 120, Iss. 16, pp. 8066-8129
Open Access | Times Cited: 460
Kevin Maik Jablonka, Daniele Ongari, Seyed Mohamad Moosavi, et al.
Chemical Reviews (2020) Vol. 120, Iss. 16, pp. 8066-8129
Open Access | Times Cited: 460
Machine learning for alloys
Gus L. W. Hart, Tim Mueller, Cormac Toher, et al.
Nature Reviews Materials (2021) Vol. 6, Iss. 8, pp. 730-755
Closed Access | Times Cited: 446
Gus L. W. Hart, Tim Mueller, Cormac Toher, et al.
Nature Reviews Materials (2021) Vol. 6, Iss. 8, pp. 730-755
Closed Access | Times Cited: 446
A perspective on conventional high-temperature superconductors at high pressure: Methods and materials
José A. Flores‐Livas, Lilia Boeri, Antonio Sanna, et al.
Physics Reports (2020) Vol. 856, pp. 1-78
Open Access | Times Cited: 440
José A. Flores‐Livas, Lilia Boeri, Antonio Sanna, et al.
Physics Reports (2020) Vol. 856, pp. 1-78
Open Access | Times Cited: 440
Less is more: Sampling chemical space with active learning
Justin S. Smith, Benjamin Nebgen, Nicholas Lubbers, et al.
The Journal of Chemical Physics (2018) Vol. 148, Iss. 24
Open Access | Times Cited: 425
Justin S. Smith, Benjamin Nebgen, Nicholas Lubbers, et al.
The Journal of Chemical Physics (2018) Vol. 148, Iss. 24
Open Access | Times Cited: 425
Atomistic Line Graph Neural Network for improved materials property predictions
Kamal Choudhary, Brian DeCost
npj Computational Materials (2021) Vol. 7, Iss. 1
Open Access | Times Cited: 406
Kamal Choudhary, Brian DeCost
npj Computational Materials (2021) Vol. 7, Iss. 1
Open Access | Times Cited: 406
Machine learning modeling of superconducting critical temperature
Valentin Stanev, Corey Oses, A. Gilad Kusne, et al.
npj Computational Materials (2018) Vol. 4, Iss. 1
Open Access | Times Cited: 397
Valentin Stanev, Corey Oses, A. Gilad Kusne, et al.
npj Computational Materials (2018) Vol. 4, Iss. 1
Open Access | Times Cited: 397
Predicting the Band Gaps of Inorganic Solids by Machine Learning
Ya Zhuo, Aria Mansouri Tehrani, Jakoah Brgoch
The Journal of Physical Chemistry Letters (2018) Vol. 9, Iss. 7, pp. 1668-1673
Closed Access | Times Cited: 380
Ya Zhuo, Aria Mansouri Tehrani, Jakoah Brgoch
The Journal of Physical Chemistry Letters (2018) Vol. 9, Iss. 7, pp. 1668-1673
Closed Access | Times Cited: 380