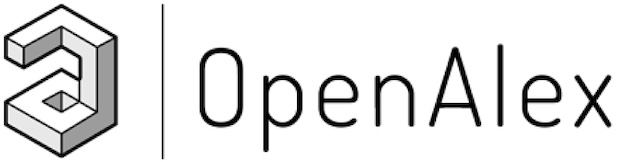
OpenAlex is a bibliographic catalogue of scientific papers, authors and institutions accessible in open access mode, named after the Library of Alexandria. It's citation coverage is excellent and I hope you will find utility in this listing of citing articles!
If you click the article title, you'll navigate to the article, as listed in CrossRef. If you click the Open Access links, you'll navigate to the "best Open Access location". Clicking the citation count will open this listing for that article. Lastly at the bottom of the page, you'll find basic pagination options.
Requested Article:
Data-Efficient Machine Learning Potentials from Transfer Learning of Periodic Correlated Electronic Structure Methods: Liquid Water at AFQMC, CCSD, and CCSD(T) Accuracy
Michael S. Chen, Joonho Lee, Hong‐Zhou Ye, et al.
Journal of Chemical Theory and Computation (2023) Vol. 19, Iss. 14, pp. 4510-4519
Closed Access | Times Cited: 44
Michael S. Chen, Joonho Lee, Hong‐Zhou Ye, et al.
Journal of Chemical Theory and Computation (2023) Vol. 19, Iss. 14, pp. 4510-4519
Closed Access | Times Cited: 44
Showing 1-25 of 44 citing articles:
How to train a neural network potential
Alea Miako Tokita, Jörg Behler
The Journal of Chemical Physics (2023) Vol. 159, Iss. 12
Open Access | Times Cited: 46
Alea Miako Tokita, Jörg Behler
The Journal of Chemical Physics (2023) Vol. 159, Iss. 12
Open Access | Times Cited: 46
Perspective: Atomistic simulations of water and aqueous systems with machine learning potentials
Amir Omranpour, Pablo Montero de Hijes, Jörg Behler, et al.
The Journal of Chemical Physics (2024) Vol. 160, Iss. 17
Open Access | Times Cited: 19
Amir Omranpour, Pablo Montero de Hijes, Jörg Behler, et al.
The Journal of Chemical Physics (2024) Vol. 160, Iss. 17
Open Access | Times Cited: 19
To Pair or not to Pair? Machine-Learned Explicitly-Correlated Electronic Structure for NaCl in Water
Niamh O’Neill, Benjamin X. Shi, Kara D. Fong, et al.
The Journal of Physical Chemistry Letters (2024) Vol. 15, Iss. 23, pp. 6081-6091
Open Access | Times Cited: 12
Niamh O’Neill, Benjamin X. Shi, Kara D. Fong, et al.
The Journal of Physical Chemistry Letters (2024) Vol. 15, Iss. 23, pp. 6081-6091
Open Access | Times Cited: 12
A personal perspective of the present status and future challenges facing thermal reaction rate theory
Eli Pollak
The Journal of Chemical Physics (2024) Vol. 160, Iss. 15
Open Access | Times Cited: 11
Eli Pollak
The Journal of Chemical Physics (2024) Vol. 160, Iss. 15
Open Access | Times Cited: 11
Data Generation for Machine Learning Interatomic Potentials and Beyond
Maksim Kulichenko, Benjamin Nebgen, Nicholas Lubbers, et al.
Chemical Reviews (2024) Vol. 124, Iss. 24, pp. 13681-13714
Closed Access | Times Cited: 10
Maksim Kulichenko, Benjamin Nebgen, Nicholas Lubbers, et al.
Chemical Reviews (2024) Vol. 124, Iss. 24, pp. 13681-13714
Closed Access | Times Cited: 10
When theory meets experiment: What does it take to accurately predict 1H NMR dipolar relaxation rates in neat liquid water from theory?
Dietmar Paschek, Johanna Busch, Angel Mary Chiramel Tony, et al.
The Journal of Chemical Physics (2025) Vol. 162, Iss. 5
Closed Access | Times Cited: 1
Dietmar Paschek, Johanna Busch, Angel Mary Chiramel Tony, et al.
The Journal of Chemical Physics (2025) Vol. 162, Iss. 5
Closed Access | Times Cited: 1
Learning together: Towards foundation models for machine learning interatomic potentials with meta-learning
Alice Allen, Nicholas Lubbers, Sakib Matin, et al.
npj Computational Materials (2024) Vol. 10, Iss. 1
Open Access | Times Cited: 6
Alice Allen, Nicholas Lubbers, Sakib Matin, et al.
npj Computational Materials (2024) Vol. 10, Iss. 1
Open Access | Times Cited: 6
Synthetic pre-training for neural-network interatomic potentials
John L. A. Gardner, Kathryn T. Baker, Volker L. Deringer
Machine Learning Science and Technology (2023) Vol. 5, Iss. 1, pp. 015003-015003
Open Access | Times Cited: 14
John L. A. Gardner, Kathryn T. Baker, Volker L. Deringer
Machine Learning Science and Technology (2023) Vol. 5, Iss. 1, pp. 015003-015003
Open Access | Times Cited: 14
Path Integral Simulations of Condensed-Phase Vibrational Spectroscopy
Stuart C. Althorpe
Annual Review of Physical Chemistry (2024) Vol. 75, Iss. 1, pp. 397-420
Closed Access | Times Cited: 5
Stuart C. Althorpe
Annual Review of Physical Chemistry (2024) Vol. 75, Iss. 1, pp. 397-420
Closed Access | Times Cited: 5
A Status Report on “Gold Standard” Machine-Learned Potentials for Water
Qi Yu, Chen Qu, Paul L. Houston, et al.
The Journal of Physical Chemistry Letters (2023) Vol. 14, Iss. 36, pp. 8077-8087
Open Access | Times Cited: 12
Qi Yu, Chen Qu, Paul L. Houston, et al.
The Journal of Physical Chemistry Letters (2023) Vol. 14, Iss. 36, pp. 8077-8087
Open Access | Times Cited: 12
Interfacing q-AQUA with a Polarizable Force Field: The Best of Both Worlds
Chen Qu, Qi Yu, Paul L. Houston, et al.
Journal of Chemical Theory and Computation (2023) Vol. 19, Iss. 12, pp. 3446-3459
Open Access | Times Cited: 11
Chen Qu, Qi Yu, Paul L. Houston, et al.
Journal of Chemical Theory and Computation (2023) Vol. 19, Iss. 12, pp. 3446-3459
Open Access | Times Cited: 11
Transfer-learned potential energy surfaces: Toward microsecond-scale molecular dynamics simulations in the gas phase at CCSD(T) quality
Silvan Käser, Markus Meuwly
The Journal of Chemical Physics (2023) Vol. 158, Iss. 21
Open Access | Times Cited: 11
Silvan Käser, Markus Meuwly
The Journal of Chemical Physics (2023) Vol. 158, Iss. 21
Open Access | Times Cited: 11
Historical development of a study of the structure and dynamics of liquids and solutions
Toshio Yamaguchi
Journal of Molecular Liquids (2024) Vol. 395, pp. 123919-123919
Closed Access | Times Cited: 4
Toshio Yamaguchi
Journal of Molecular Liquids (2024) Vol. 395, pp. 123919-123919
Closed Access | Times Cited: 4
Periodic Local Coupled-Cluster Theory for Insulators and Metals
Hong‐Zhou Ye, Timothy C. Berkelbach
Journal of Chemical Theory and Computation (2024) Vol. 20, Iss. 20, pp. 8948-8959
Closed Access | Times Cited: 4
Hong‐Zhou Ye, Timothy C. Berkelbach
Journal of Chemical Theory and Computation (2024) Vol. 20, Iss. 20, pp. 8948-8959
Closed Access | Times Cited: 4
Random Sampling Versus Active Learning Algorithms for Machine Learning Potentials of Quantum Liquid Water
Nore Stolte, János Daru, Harald Forbert, et al.
Journal of Chemical Theory and Computation (2025)
Open Access
Nore Stolte, János Daru, Harald Forbert, et al.
Journal of Chemical Theory and Computation (2025)
Open Access
Machine Learning Potentials for Heterogeneous Catalysis
Amir Omranpour, Jan Elsner, K. Nikolas Lausch, et al.
ACS Catalysis (2025) Vol. 15, Iss. 3, pp. 1616-1634
Open Access
Amir Omranpour, Jan Elsner, K. Nikolas Lausch, et al.
ACS Catalysis (2025) Vol. 15, Iss. 3, pp. 1616-1634
Open Access
Accurate Neural Network Fine-Tuning Approach for Transferable Ab Initio Energy Prediction across Varying Molecular and Crystalline Scales
Wai-Pan Ng, Zili Zhang, Jun Yang
Journal of Chemical Theory and Computation (2025)
Open Access
Wai-Pan Ng, Zili Zhang, Jun Yang
Journal of Chemical Theory and Computation (2025)
Open Access
Machine Learning Nonadiabatic Dynamics: Eliminating Phase Freedom of Nonadiabatic Couplings with the State-Interaction State-Averaged Spin-Restricted Ensemble-Referenced Kohn–Sham Approach
Sung Wook Moon, Soohaeng Yoo Willow, Tae Park, et al.
Journal of Chemical Theory and Computation (2025)
Closed Access
Sung Wook Moon, Soohaeng Yoo Willow, Tae Park, et al.
Journal of Chemical Theory and Computation (2025)
Closed Access
Application of Machine Learning Interatomic Potentials in Heterogeneous Catalysis
Gbolagade Olajide, Khagendra Baral, Sophia Ezendu, et al.
(2025)
Closed Access
Gbolagade Olajide, Khagendra Baral, Sophia Ezendu, et al.
(2025)
Closed Access
Data-efficient construction of high-fidelity graph deep learning interatomic potentials
Tsz Wai Ko, Shyue Ping Ong
npj Computational Materials (2025) Vol. 11, Iss. 1
Open Access
Tsz Wai Ko, Shyue Ping Ong
npj Computational Materials (2025) Vol. 11, Iss. 1
Open Access
On the design space between molecular mechanics and machine learning force fields
Yuanqing Wang, Kenichiro Takaba, Michael S. Chen, et al.
Applied Physics Reviews (2025) Vol. 12, Iss. 2
Open Access
Yuanqing Wang, Kenichiro Takaba, Michael S. Chen, et al.
Applied Physics Reviews (2025) Vol. 12, Iss. 2
Open Access
Machine Learning for Bridging the Gap between Density Functional Theory and Coupled Cluster Energies
Marcel Ruth, Dennis Gerbig, Peter R. Schreiner
Journal of Chemical Theory and Computation (2023) Vol. 19, Iss. 15, pp. 4912-4920
Closed Access | Times Cited: 9
Marcel Ruth, Dennis Gerbig, Peter R. Schreiner
Journal of Chemical Theory and Computation (2023) Vol. 19, Iss. 15, pp. 4912-4920
Closed Access | Times Cited: 9
Coupled cluster finite temperature simulations of periodic materials via machine learning
Basile Herzog, Alejandro Gallo, Felix Hummel, et al.
npj Computational Materials (2024) Vol. 10, Iss. 1
Open Access | Times Cited: 3
Basile Herzog, Alejandro Gallo, Felix Hummel, et al.
npj Computational Materials (2024) Vol. 10, Iss. 1
Open Access | Times Cited: 3
Active Sparse Bayesian Committee Machine Potential for Isothermal-Isobaric Molecular Dynamics Simulations
Soohaeng Yoo Willow, Dong Geon Kim, R. Sundheep, et al.
Physical Chemistry Chemical Physics (2024) Vol. 26, Iss. 33, pp. 22073-22082
Open Access | Times Cited: 3
Soohaeng Yoo Willow, Dong Geon Kim, R. Sundheep, et al.
Physical Chemistry Chemical Physics (2024) Vol. 26, Iss. 33, pp. 22073-22082
Open Access | Times Cited: 3
Nuclear Quantum Effects in Liquid Water Are Marginal for Its Average Structure but Significant for Dynamics
Nore Stolte, János Daru, Harald Forbert, et al.
The Journal of Physical Chemistry Letters (2024), pp. 12144-12150
Closed Access | Times Cited: 3
Nore Stolte, János Daru, Harald Forbert, et al.
The Journal of Physical Chemistry Letters (2024), pp. 12144-12150
Closed Access | Times Cited: 3