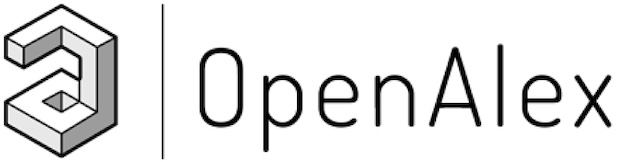
OpenAlex is a bibliographic catalogue of scientific papers, authors and institutions accessible in open access mode, named after the Library of Alexandria. It's citation coverage is excellent and I hope you will find utility in this listing of citing articles!
If you click the article title, you'll navigate to the article, as listed in CrossRef. If you click the Open Access links, you'll navigate to the "best Open Access location". Clicking the citation count will open this listing for that article. Lastly at the bottom of the page, you'll find basic pagination options.
Requested Article:
Linear Atomic Cluster Expansion Force Fields for Organic Molecules: Beyond RMSE
Dávid Péter Kovács, Cas van der Oord, Jiri Kucera, et al.
Journal of Chemical Theory and Computation (2021) Vol. 17, Iss. 12, pp. 7696-7711
Open Access | Times Cited: 75
Dávid Péter Kovács, Cas van der Oord, Jiri Kucera, et al.
Journal of Chemical Theory and Computation (2021) Vol. 17, Iss. 12, pp. 7696-7711
Open Access | Times Cited: 75
Showing 1-25 of 75 citing articles:
E(3)-equivariant graph neural networks for data-efficient and accurate interatomic potentials
Simon Batzner, Albert Musaelian, Lixin Sun, et al.
Nature Communications (2022) Vol. 13, Iss. 1
Open Access | Times Cited: 802
Simon Batzner, Albert Musaelian, Lixin Sun, et al.
Nature Communications (2022) Vol. 13, Iss. 1
Open Access | Times Cited: 802
Learning local equivariant representations for large-scale atomistic dynamics
Albert Musaelian, Simon Batzner, Anders Johansson, et al.
Nature Communications (2023) Vol. 14, Iss. 1
Open Access | Times Cited: 275
Albert Musaelian, Simon Batzner, Anders Johansson, et al.
Nature Communications (2023) Vol. 14, Iss. 1
Open Access | Times Cited: 275
DeePMD-kit v2: A software package for deep potential models
Jinzhe Zeng, Duo Zhang, Denghui Lu, et al.
The Journal of Chemical Physics (2023) Vol. 159, Iss. 5
Open Access | Times Cited: 188
Jinzhe Zeng, Duo Zhang, Denghui Lu, et al.
The Journal of Chemical Physics (2023) Vol. 159, Iss. 5
Open Access | Times Cited: 188
GPUMD: A package for constructing accurate machine-learned potentials and performing highly efficient atomistic simulations
Zheyong Fan, Yanzhou Wang, Penghua Ying, et al.
The Journal of Chemical Physics (2022) Vol. 157, Iss. 11
Open Access | Times Cited: 142
Zheyong Fan, Yanzhou Wang, Penghua Ying, et al.
The Journal of Chemical Physics (2022) Vol. 157, Iss. 11
Open Access | Times Cited: 142
How to validate machine-learned interatomic potentials
Joe D. Morrow, John L. A. Gardner, Volker L. Deringer
The Journal of Chemical Physics (2023) Vol. 158, Iss. 12
Open Access | Times Cited: 60
Joe D. Morrow, John L. A. Gardner, Volker L. Deringer
The Journal of Chemical Physics (2023) Vol. 158, Iss. 12
Open Access | Times Cited: 60
Hyperactive learning for data-driven interatomic potentials
Cas van der Oord, Matthias Sachs, Dávid Péter Kovács, et al.
npj Computational Materials (2023) Vol. 9, Iss. 1
Open Access | Times Cited: 52
Cas van der Oord, Matthias Sachs, Dávid Péter Kovács, et al.
npj Computational Materials (2023) Vol. 9, Iss. 1
Open Access | Times Cited: 52
Evaluation of the MACE force field architecture: From medicinal chemistry to materials science
Dávid Péter Kovács, Ilyes Batatia, Eszter Sára Arany, et al.
The Journal of Chemical Physics (2023) Vol. 159, Iss. 4
Open Access | Times Cited: 50
Dávid Péter Kovács, Ilyes Batatia, Eszter Sára Arany, et al.
The Journal of Chemical Physics (2023) Vol. 159, Iss. 4
Open Access | Times Cited: 50
Non-collinear magnetic atomic cluster expansion for iron
Matteo Rinaldi, Matous Mrovec, Anton Bochkarev, et al.
npj Computational Materials (2024) Vol. 10, Iss. 1
Open Access | Times Cited: 17
Matteo Rinaldi, Matous Mrovec, Anton Bochkarev, et al.
npj Computational Materials (2024) Vol. 10, Iss. 1
Open Access | Times Cited: 17
The design space of E(3)-equivariant atom-centred interatomic potentials
Ilyes Batatia, Simon Batzner, Dávid Péter Kovács, et al.
Nature Machine Intelligence (2025) Vol. 7, Iss. 1, pp. 56-67
Open Access | Times Cited: 2
Ilyes Batatia, Simon Batzner, Dávid Péter Kovács, et al.
Nature Machine Intelligence (2025) Vol. 7, Iss. 1, pp. 56-67
Open Access | Times Cited: 2
Efficient parametrization of the atomic cluster expansion
Anton Bochkarev, Yury Lysogorskiy, Sarath Menon, et al.
Physical Review Materials (2022) Vol. 6, Iss. 1
Closed Access | Times Cited: 61
Anton Bochkarev, Yury Lysogorskiy, Sarath Menon, et al.
Physical Review Materials (2022) Vol. 6, Iss. 1
Closed Access | Times Cited: 61
BIGDML—Towards accurate quantum machine learning force fields for materials
Huziel E. Sauceda, Luis E. Gálvez-González, Stefan Chmiela, et al.
Nature Communications (2022) Vol. 13, Iss. 1
Open Access | Times Cited: 47
Huziel E. Sauceda, Luis E. Gálvez-González, Stefan Chmiela, et al.
Nature Communications (2022) Vol. 13, Iss. 1
Open Access | Times Cited: 47
Permutationally invariant polynomial regression for energies and gradients, using reverse differentiation, achieves orders of magnitude speed-up with high precision compared to other machine learning methods
Paul L. Houston, Chen Qu, Apurba Nandi, et al.
The Journal of Chemical Physics (2022) Vol. 156, Iss. 4
Open Access | Times Cited: 40
Paul L. Houston, Chen Qu, Apurba Nandi, et al.
The Journal of Chemical Physics (2022) Vol. 156, Iss. 4
Open Access | Times Cited: 40
Fast uncertainty estimates in deep learning interatomic potentials
Albert Zhu, Simon Batzner, Albert Musaelian, et al.
The Journal of Chemical Physics (2023) Vol. 158, Iss. 16
Open Access | Times Cited: 30
Albert Zhu, Simon Batzner, Albert Musaelian, et al.
The Journal of Chemical Physics (2023) Vol. 158, Iss. 16
Open Access | Times Cited: 30
Single-model uncertainty quantification in neural network potentials does not consistently outperform model ensembles
Aik Rui Tan, Shingo Urata, Samuel Goldman, et al.
npj Computational Materials (2023) Vol. 9, Iss. 1
Open Access | Times Cited: 27
Aik Rui Tan, Shingo Urata, Samuel Goldman, et al.
npj Computational Materials (2023) Vol. 9, Iss. 1
Open Access | Times Cited: 27
Flow-Matching: Efficient Coarse-Graining of Molecular Dynamics without Forces
Jonas Köhler, Yaoyi Chen, Andreas Krämer, et al.
Journal of Chemical Theory and Computation (2023) Vol. 19, Iss. 3, pp. 942-952
Open Access | Times Cited: 26
Jonas Köhler, Yaoyi Chen, Andreas Krämer, et al.
Journal of Chemical Theory and Computation (2023) Vol. 19, Iss. 3, pp. 942-952
Open Access | Times Cited: 26
Ultra-fast interpretable machine-learning potentials
Stephen Xie, Matthias Rupp, Richard G. Hennig
npj Computational Materials (2023) Vol. 9, Iss. 1
Open Access | Times Cited: 24
Stephen Xie, Matthias Rupp, Richard G. Hennig
npj Computational Materials (2023) Vol. 9, Iss. 1
Open Access | Times Cited: 24
Machine Learning Potentials with the Iterative Boltzmann Inversion: Training to Experiment
Sakib Matin, Alice Allen, Justin S. Smith, et al.
Journal of Chemical Theory and Computation (2024) Vol. 20, Iss. 3, pp. 1274-1281
Open Access | Times Cited: 11
Sakib Matin, Alice Allen, Justin S. Smith, et al.
Journal of Chemical Theory and Computation (2024) Vol. 20, Iss. 3, pp. 1274-1281
Open Access | Times Cited: 11
Complexity of many-body interactions in transition metals via machine-learned force fields from the TM23 data set
Cameron J. Owen, Steven B. Torrisi, Yu Xie, et al.
npj Computational Materials (2024) Vol. 10, Iss. 1
Open Access | Times Cited: 11
Cameron J. Owen, Steven B. Torrisi, Yu Xie, et al.
npj Computational Materials (2024) Vol. 10, Iss. 1
Open Access | Times Cited: 11
Modelling chemical processes in explicit solvents with machine learning potentials
Hanwen Zhang, Veronika Jurásková, Fernanda Duarte
Nature Communications (2024) Vol. 15, Iss. 1
Open Access | Times Cited: 8
Hanwen Zhang, Veronika Jurásková, Fernanda Duarte
Nature Communications (2024) Vol. 15, Iss. 1
Open Access | Times Cited: 8
No Headache for PIPs: A PIP Potential for Aspirin Runs Much Faster and with Similar Precision Than Other Machine-Learned Potentials
Paul L. Houston, Chen Qu, Qi Yu, et al.
Journal of Chemical Theory and Computation (2024) Vol. 20, Iss. 8, pp. 3008-3018
Open Access | Times Cited: 6
Paul L. Houston, Chen Qu, Qi Yu, et al.
Journal of Chemical Theory and Computation (2024) Vol. 20, Iss. 8, pp. 3008-3018
Open Access | Times Cited: 6
Low-index mesoscopic surface reconstructions of Au surfaces using Bayesian force fields
Cameron J. Owen, Yu Xie, Anders Johansson, et al.
Nature Communications (2024) Vol. 15, Iss. 1
Open Access | Times Cited: 6
Cameron J. Owen, Yu Xie, Anders Johansson, et al.
Nature Communications (2024) Vol. 15, Iss. 1
Open Access | Times Cited: 6
Mapping the Binary Covalent Alloy Space to Pursue Superior Nitrogen Reduction Reaction Catalysts
Cooper Tezak, Sophie Gerits, Benjamin Rich, et al.
Advanced Energy Materials (2024) Vol. 14, Iss. 28
Closed Access | Times Cited: 6
Cooper Tezak, Sophie Gerits, Benjamin Rich, et al.
Advanced Energy Materials (2024) Vol. 14, Iss. 28
Closed Access | Times Cited: 6
Creation of an Fe3P Schreibersite Density Functional Tight Binding Model for Astrobiological Simulations
Riccardo Dettori, Nir Goldman
The Journal of Physical Chemistry A (2025)
Closed Access
Riccardo Dettori, Nir Goldman
The Journal of Physical Chemistry A (2025)
Closed Access
Grappa – a machine learned molecular mechanics force field
Leif Seute, Eric Hartmann, Jan Stühmer, et al.
Chemical Science (2025)
Open Access
Leif Seute, Eric Hartmann, Jan Stühmer, et al.
Chemical Science (2025)
Open Access
Computationally efficient machine-learned model for GST phase change materials via direct and indirect learning
Owen R. Dunton, Tom Arbaugh, Francis W. Starr
The Journal of Chemical Physics (2025) Vol. 162, Iss. 3
Open Access
Owen R. Dunton, Tom Arbaugh, Francis W. Starr
The Journal of Chemical Physics (2025) Vol. 162, Iss. 3
Open Access