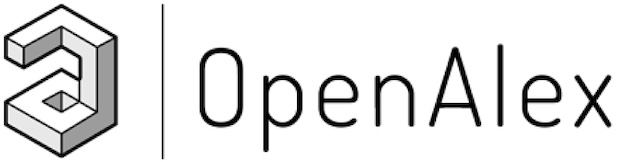
OpenAlex is a bibliographic catalogue of scientific papers, authors and institutions accessible in open access mode, named after the Library of Alexandria. It's citation coverage is excellent and I hope you will find utility in this listing of citing articles!
If you click the article title, you'll navigate to the article, as listed in CrossRef. If you click the Open Access links, you'll navigate to the "best Open Access location". Clicking the citation count will open this listing for that article. Lastly at the bottom of the page, you'll find basic pagination options.
Requested Article:
Revolutionizing Membrane Design Using Machine Learning-Bayesian Optimization
Haiping Gao, Shifa Zhong, Wenlong Zhang, et al.
Environmental Science & Technology (2021) Vol. 56, Iss. 4, pp. 2572-2581
Closed Access | Times Cited: 104
Haiping Gao, Shifa Zhong, Wenlong Zhang, et al.
Environmental Science & Technology (2021) Vol. 56, Iss. 4, pp. 2572-2581
Closed Access | Times Cited: 104
Showing 1-25 of 104 citing articles:
Machine Learning in Environmental Research: Common Pitfalls and Best Practices
Jun‐Jie Zhu, Meiqi Yang, Zhiyong Jason Ren
Environmental Science & Technology (2023) Vol. 57, Iss. 46, pp. 17671-17689
Closed Access | Times Cited: 215
Jun‐Jie Zhu, Meiqi Yang, Zhiyong Jason Ren
Environmental Science & Technology (2023) Vol. 57, Iss. 46, pp. 17671-17689
Closed Access | Times Cited: 215
Pressure-driven membrane desalination
Weifan Liu, J. Livingston, Li Wang, et al.
Nature Reviews Methods Primers (2024) Vol. 4, Iss. 1
Closed Access | Times Cited: 35
Weifan Liu, J. Livingston, Li Wang, et al.
Nature Reviews Methods Primers (2024) Vol. 4, Iss. 1
Closed Access | Times Cited: 35
Design and Machine Learning Prediction of In Situ Grown PDA-Stabilized MOF (UiO-66-NH2) Membrane for Low-Pressure Separation of Emulsified Oily Wastewater
Jamilu Usman, Sani I. Abba, Nadeem Baig, et al.
ACS Applied Materials & Interfaces (2024) Vol. 16, Iss. 13, pp. 16271-16289
Closed Access | Times Cited: 25
Jamilu Usman, Sani I. Abba, Nadeem Baig, et al.
ACS Applied Materials & Interfaces (2024) Vol. 16, Iss. 13, pp. 16271-16289
Closed Access | Times Cited: 25
Machine learning for membrane design in energy production, gas separation, and water treatment: a review
Ahmed I. Osman, Mahmoud Nasr, Mohamed Farghali, et al.
Environmental Chemistry Letters (2024) Vol. 22, Iss. 2, pp. 505-560
Open Access | Times Cited: 23
Ahmed I. Osman, Mahmoud Nasr, Mohamed Farghali, et al.
Environmental Chemistry Letters (2024) Vol. 22, Iss. 2, pp. 505-560
Open Access | Times Cited: 23
Prediction of effluent total nitrogen and energy consumption in wastewater treatment plants: Bayesian optimization machine learning methods
Gang Ye, Jinquan Wan, Zhicheng Deng, et al.
Bioresource Technology (2024) Vol. 395, pp. 130361-130361
Closed Access | Times Cited: 20
Gang Ye, Jinquan Wan, Zhicheng Deng, et al.
Bioresource Technology (2024) Vol. 395, pp. 130361-130361
Closed Access | Times Cited: 20
Machine Learning in Membrane Design: From Property Prediction to AI-Guided Optimization
Zhonglin Cao, Omid Barati Farimani, Janghoon Ock, et al.
Nano Letters (2024) Vol. 24, Iss. 10, pp. 2953-2960
Open Access | Times Cited: 16
Zhonglin Cao, Omid Barati Farimani, Janghoon Ock, et al.
Nano Letters (2024) Vol. 24, Iss. 10, pp. 2953-2960
Open Access | Times Cited: 16
Active Learning-Based Guided Synthesis of Engineered Biochar for CO2 Capture
Xiangzhou Yuan, Manu Suvarna, Juin Yau Lim, et al.
Environmental Science & Technology (2024) Vol. 58, Iss. 15, pp. 6628-6636
Open Access | Times Cited: 15
Xiangzhou Yuan, Manu Suvarna, Juin Yau Lim, et al.
Environmental Science & Technology (2024) Vol. 58, Iss. 15, pp. 6628-6636
Open Access | Times Cited: 15
Interpretable Machine Learning Models Delivering a New Perspective for the Reaction Mechanism between Organic Pollutants and Oxidative Radicals
Yiqiu Wu, Zhixiang Wang, Guangfei Yu, et al.
Environmental Science & Technology (2025)
Closed Access | Times Cited: 1
Yiqiu Wu, Zhixiang Wang, Guangfei Yu, et al.
Environmental Science & Technology (2025)
Closed Access | Times Cited: 1
Optimization algorithms for modeling conversion and naphtha yield in the catalytic co-cracking of plastic in HVGO
A.G. Usman, Abdullah Aitani, Jamilu Usman, et al.
Process Safety and Environmental Protection (2025), pp. 106958-106958
Closed Access | Times Cited: 1
A.G. Usman, Abdullah Aitani, Jamilu Usman, et al.
Process Safety and Environmental Protection (2025), pp. 106958-106958
Closed Access | Times Cited: 1
A critical review on thin-film nanocomposite membranes enabled by nanomaterials incorporated in different positions and with diverse dimensions: Performance comparison and mechanisms
Xinxin Wei, Yanling Liu, Junfeng Zheng, et al.
Journal of Membrane Science (2022) Vol. 661, pp. 120952-120952
Closed Access | Times Cited: 64
Xinxin Wei, Yanling Liu, Junfeng Zheng, et al.
Journal of Membrane Science (2022) Vol. 661, pp. 120952-120952
Closed Access | Times Cited: 64
Machine learning for membrane design and discovery
Haoyu Yin, Muzi Xu, Zhiyao Luo, et al.
Green Energy & Environment (2022) Vol. 9, Iss. 1, pp. 54-70
Open Access | Times Cited: 49
Haoyu Yin, Muzi Xu, Zhiyao Luo, et al.
Green Energy & Environment (2022) Vol. 9, Iss. 1, pp. 54-70
Open Access | Times Cited: 49
Prediction of organic contaminant rejection by nanofiltration and reverse osmosis membranes using interpretable machine learning models
Tengyi Zhu, Yu Zhang, Cuicui Tao, et al.
The Science of The Total Environment (2022) Vol. 857, pp. 159348-159348
Closed Access | Times Cited: 44
Tengyi Zhu, Yu Zhang, Cuicui Tao, et al.
The Science of The Total Environment (2022) Vol. 857, pp. 159348-159348
Closed Access | Times Cited: 44
Understanding and Designing a High-Performance Ultrafiltration Membrane Using Machine Learning
Haiping Gao, Shifa Zhong, Raghav Dangayach, et al.
Environmental Science & Technology (2023) Vol. 57, Iss. 46, pp. 17831-17840
Open Access | Times Cited: 38
Haiping Gao, Shifa Zhong, Raghav Dangayach, et al.
Environmental Science & Technology (2023) Vol. 57, Iss. 46, pp. 17831-17840
Open Access | Times Cited: 38
Predicting Extraction Selectivity of Acetic Acid in Pervaporation by Machine Learning Models with Data Leakage Management
Meiqi Yang, Jun‐Jie Zhu, Allyson L. McGaughey, et al.
Environmental Science & Technology (2023) Vol. 57, Iss. 14, pp. 5934-5946
Closed Access | Times Cited: 31
Meiqi Yang, Jun‐Jie Zhu, Allyson L. McGaughey, et al.
Environmental Science & Technology (2023) Vol. 57, Iss. 14, pp. 5934-5946
Closed Access | Times Cited: 31
Machine learning algorithms for high-resolution prediction of spatiotemporal distribution of air pollution from meteorological and soil parameters
Tao Hai, Ali H. Jawad, A.H. Shather, et al.
Environment International (2023) Vol. 175, pp. 107931-107931
Open Access | Times Cited: 28
Tao Hai, Ali H. Jawad, A.H. Shather, et al.
Environment International (2023) Vol. 175, pp. 107931-107931
Open Access | Times Cited: 28
The Minus Approach Can Redefine the Standard of Practice of Drinking Water Treatment
Elliot Reid, Thomas Igou, Yangying Zhao, et al.
Environmental Science & Technology (2023) Vol. 57, Iss. 18, pp. 7150-7161
Open Access | Times Cited: 27
Elliot Reid, Thomas Igou, Yangying Zhao, et al.
Environmental Science & Technology (2023) Vol. 57, Iss. 18, pp. 7150-7161
Open Access | Times Cited: 27
MatGPT: A Vane of Materials Informatics from Past, Present, to Future
Zhilong Wang, An Chen, Kehao Tao, et al.
Advanced Materials (2023) Vol. 36, Iss. 6
Closed Access | Times Cited: 26
Zhilong Wang, An Chen, Kehao Tao, et al.
Advanced Materials (2023) Vol. 36, Iss. 6
Closed Access | Times Cited: 26
Tree-structured parzen estimator optimized-automated machine learning assisted by meta–analysis for predicting biochar–driven N2O mitigation effect in constructed wetlands
Bi–Ni Jiang, Yingying Zhang, Zhiyong Zhang, et al.
Journal of Environmental Management (2024) Vol. 354, pp. 120335-120335
Closed Access | Times Cited: 13
Bi–Ni Jiang, Yingying Zhang, Zhiyong Zhang, et al.
Journal of Environmental Management (2024) Vol. 354, pp. 120335-120335
Closed Access | Times Cited: 13
Enhancing biomass conversion to bioenergy with machine learning: Gains and problems
Rupeng Wang, Zixiang He, Honglin Chen, et al.
The Science of The Total Environment (2024) Vol. 927, pp. 172310-172310
Closed Access | Times Cited: 11
Rupeng Wang, Zixiang He, Honglin Chen, et al.
The Science of The Total Environment (2024) Vol. 927, pp. 172310-172310
Closed Access | Times Cited: 11
Different applications of machine learning approaches in materials science and engineering: Comprehensive review
Yan Cao, Ali Taghvaie Nakhjiri, Mahdi Ghadiri
Engineering Applications of Artificial Intelligence (2024) Vol. 135, pp. 108783-108783
Closed Access | Times Cited: 11
Yan Cao, Ali Taghvaie Nakhjiri, Mahdi Ghadiri
Engineering Applications of Artificial Intelligence (2024) Vol. 135, pp. 108783-108783
Closed Access | Times Cited: 11
Exploring chemistry and additive manufacturing design spaces: a perspective on computationally-guided design of printable alloys
Sofia Sheikh, Brent Vela, Vahid Attari, et al.
Materials Research Letters (2024) Vol. 12, Iss. 4, pp. 235-263
Open Access | Times Cited: 10
Sofia Sheikh, Brent Vela, Vahid Attari, et al.
Materials Research Letters (2024) Vol. 12, Iss. 4, pp. 235-263
Open Access | Times Cited: 10
Understanding Rejection Mechanisms of Trace Organic Contaminants by Polyamide Membranes via Data-Knowledge Codriven Machine Learning
Hejia Wang, Jin Zeng, Ruobin Dai, et al.
Environmental Science & Technology (2024) Vol. 58, Iss. 13, pp. 5878-5888
Closed Access | Times Cited: 10
Hejia Wang, Jin Zeng, Ruobin Dai, et al.
Environmental Science & Technology (2024) Vol. 58, Iss. 13, pp. 5878-5888
Closed Access | Times Cited: 10
The impact of Bayesian optimization on feature selection
Kaixin Yang, Long Liu, Yalu Wen
Scientific Reports (2024) Vol. 14, Iss. 1
Open Access | Times Cited: 10
Kaixin Yang, Long Liu, Yalu Wen
Scientific Reports (2024) Vol. 14, Iss. 1
Open Access | Times Cited: 10
Machine learning for the advancement of membrane science and technology: A critical review
Gergő Ignácz, Lana Bader, Aron K. Beke, et al.
Journal of Membrane Science (2024) Vol. 713, pp. 123256-123256
Open Access | Times Cited: 8
Gergő Ignácz, Lana Bader, Aron K. Beke, et al.
Journal of Membrane Science (2024) Vol. 713, pp. 123256-123256
Open Access | Times Cited: 8
MOF or COF membranes for olefin/paraffin separation: Current status and future research directions
Ruicong Wei, Xiaowei Liu, Zhiping Lai
Advanced Membranes (2022) Vol. 2, pp. 100035-100035
Open Access | Times Cited: 33
Ruicong Wei, Xiaowei Liu, Zhiping Lai
Advanced Membranes (2022) Vol. 2, pp. 100035-100035
Open Access | Times Cited: 33