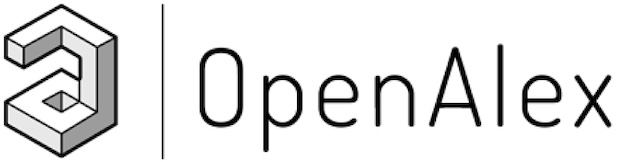
OpenAlex is a bibliographic catalogue of scientific papers, authors and institutions accessible in open access mode, named after the Library of Alexandria. It's citation coverage is excellent and I hope you will find utility in this listing of citing articles!
If you click the article title, you'll navigate to the article, as listed in CrossRef. If you click the Open Access links, you'll navigate to the "best Open Access location". Clicking the citation count will open this listing for that article. Lastly at the bottom of the page, you'll find basic pagination options.
Requested Article:
Deep learning identifies morphological patterns of homologous recombination deficiency in luminal breast cancers from whole slide images
Tristan Lazard, Guillaume Bataillon, Peter Naylor, et al.
Cell Reports Medicine (2022) Vol. 3, Iss. 12, pp. 100872-100872
Open Access | Times Cited: 47
Tristan Lazard, Guillaume Bataillon, Peter Naylor, et al.
Cell Reports Medicine (2022) Vol. 3, Iss. 12, pp. 100872-100872
Open Access | Times Cited: 47
Showing 1-25 of 47 citing articles:
Scaling Self-Supervised Learning for Histopathology with Masked Image Modeling
Alexandre Filiot, Ridouane Ghermi, Antoine Olivier, et al.
medRxiv (Cold Spring Harbor Laboratory) (2023)
Open Access | Times Cited: 56
Alexandre Filiot, Ridouane Ghermi, Antoine Olivier, et al.
medRxiv (Cold Spring Harbor Laboratory) (2023)
Open Access | Times Cited: 56
Artificial intelligence-based biomarkers for treatment decisions in oncology
Marta Ligero, Omar S.M. El Nahhas, Mihaela Aldea, et al.
Trends in cancer (2025)
Open Access | Times Cited: 1
Marta Ligero, Omar S.M. El Nahhas, Mihaela Aldea, et al.
Trends in cancer (2025)
Open Access | Times Cited: 1
Biological insights and novel biomarker discovery through deep learning approaches in breast cancer histopathology
Divneet Mandair, Jorge S. Reis‐Filho, Alan Ashworth
npj Breast Cancer (2023) Vol. 9, Iss. 1
Open Access | Times Cited: 33
Divneet Mandair, Jorge S. Reis‐Filho, Alan Ashworth
npj Breast Cancer (2023) Vol. 9, Iss. 1
Open Access | Times Cited: 33
Artificial intelligence in ovarian cancer histopathology: a systematic review
Jack Breen, Katrina J. Allen, Kieran Zucker, et al.
npj Precision Oncology (2023) Vol. 7, Iss. 1
Open Access | Times Cited: 33
Jack Breen, Katrina J. Allen, Kieran Zucker, et al.
npj Precision Oncology (2023) Vol. 7, Iss. 1
Open Access | Times Cited: 33
Computational pathology in cancer diagnosis, prognosis, and prediction – present day and prospects
Gregory Verghese, Jochen K. Lennerz, Danny Ruta, et al.
The Journal of Pathology (2023) Vol. 260, Iss. 5, pp. 551-563
Open Access | Times Cited: 32
Gregory Verghese, Jochen K. Lennerz, Danny Ruta, et al.
The Journal of Pathology (2023) Vol. 260, Iss. 5, pp. 551-563
Open Access | Times Cited: 32
Publicly available datasets of breast histopathology H&E whole-slide images: A scoping review
Masoud Tafavvoghi, Lars Ailo Bongo, Nikita Shvetsov, et al.
Journal of Pathology Informatics (2024) Vol. 15, pp. 100363-100363
Open Access | Times Cited: 10
Masoud Tafavvoghi, Lars Ailo Bongo, Nikita Shvetsov, et al.
Journal of Pathology Informatics (2024) Vol. 15, pp. 100363-100363
Open Access | Times Cited: 10
Deep Learning Artificial Intelligence Predicts Homologous Recombination Deficiency and Platinum Response From Histologic Slides
Erik N. Bergstrom, Ammal Abbasi, Marcos Díaz‐Gay, et al.
Journal of Clinical Oncology (2024) Vol. 42, Iss. 30, pp. 3550-3560
Open Access | Times Cited: 9
Erik N. Bergstrom, Ammal Abbasi, Marcos Díaz‐Gay, et al.
Journal of Clinical Oncology (2024) Vol. 42, Iss. 30, pp. 3550-3560
Open Access | Times Cited: 9
Immune-tumor interaction dictates spatially directed evolution of esophageal squamous cell carcinoma
Yong Zhou, Shanlan Mo, Heyang Cui, et al.
National Science Review (2024) Vol. 11, Iss. 5
Open Access | Times Cited: 8
Yong Zhou, Shanlan Mo, Heyang Cui, et al.
National Science Review (2024) Vol. 11, Iss. 5
Open Access | Times Cited: 8
Artificial intelligence in digital histopathology for predicting patient prognosis and treatment efficacy in breast cancer
Christine McCaffrey, Chowdhury Arif Jahangir, Clodagh Murphy, et al.
Expert Review of Molecular Diagnostics (2024) Vol. 24, Iss. 5, pp. 363-377
Open Access | Times Cited: 8
Christine McCaffrey, Chowdhury Arif Jahangir, Clodagh Murphy, et al.
Expert Review of Molecular Diagnostics (2024) Vol. 24, Iss. 5, pp. 363-377
Open Access | Times Cited: 8
Molecular Basis of Breast Tumor Heterogeneity
Esra Dikoglu, Fresia Pareja
Advances in experimental medicine and biology (2025), pp. 237-257
Closed Access | Times Cited: 1
Esra Dikoglu, Fresia Pareja
Advances in experimental medicine and biology (2025), pp. 237-257
Closed Access | Times Cited: 1
The slow-paced digital evolution of pathology: lights and shadows from a multifaceted board
Alessandro Caputo, Vincenzo L’Imperio, Francesco Merolla, et al.
Pathologica (2023) Vol. 115, Iss. 3, pp. 127-136
Open Access | Times Cited: 17
Alessandro Caputo, Vincenzo L’Imperio, Francesco Merolla, et al.
Pathologica (2023) Vol. 115, Iss. 3, pp. 127-136
Open Access | Times Cited: 17
Deep Learning for Detecting BRCA Mutations in High-Grade Ovarian Cancer Based on an Innovative Tumor Segmentation Method From Whole Slide Images
Raphaël Bourgade, Noémie Rabilloud, Tanguy Perennec, et al.
Modern Pathology (2023) Vol. 36, Iss. 11, pp. 100304-100304
Open Access | Times Cited: 16
Raphaël Bourgade, Noémie Rabilloud, Tanguy Perennec, et al.
Modern Pathology (2023) Vol. 36, Iss. 11, pp. 100304-100304
Open Access | Times Cited: 16
DNA damage response in breast cancer and its significant role in guiding novel precise therapies
Jiayi Li, Ziqi Jia, Dong Lin, et al.
Biomarker Research (2024) Vol. 12, Iss. 1
Open Access | Times Cited: 7
Jiayi Li, Ziqi Jia, Dong Lin, et al.
Biomarker Research (2024) Vol. 12, Iss. 1
Open Access | Times Cited: 7
Microsnoop: A generalist tool for microscopy image representation
Dejin Xun, Rui Wang, Xingcai Zhang, et al.
The Innovation (2024) Vol. 5, Iss. 1, pp. 100541-100541
Open Access | Times Cited: 6
Dejin Xun, Rui Wang, Xingcai Zhang, et al.
The Innovation (2024) Vol. 5, Iss. 1, pp. 100541-100541
Open Access | Times Cited: 6
Deep learning applications in breast cancer histopathological imaging: diagnosis, treatment, and prognosis
Bitao Jiang, Lingling Bao, Songqin He, et al.
Breast Cancer Research (2024) Vol. 26, Iss. 1
Open Access | Times Cited: 5
Bitao Jiang, Lingling Bao, Songqin He, et al.
Breast Cancer Research (2024) Vol. 26, Iss. 1
Open Access | Times Cited: 5
Early Breast Cancer Risk Assessment: Integrating Histopathology with Artificial Intelligence
Mariia Ivanova, Carlo Pescia, Dario Trapani, et al.
Cancers (2024) Vol. 16, Iss. 11, pp. 1981-1981
Open Access | Times Cited: 5
Mariia Ivanova, Carlo Pescia, Dario Trapani, et al.
Cancers (2024) Vol. 16, Iss. 11, pp. 1981-1981
Open Access | Times Cited: 5
Histopathology and proteomics are synergistic for high-grade serous ovarian cancer platinum response prediction
Oz Kilim, Alex Olar, András Biricz, et al.
npj Precision Oncology (2025) Vol. 9, Iss. 1
Open Access
Oz Kilim, Alex Olar, András Biricz, et al.
npj Precision Oncology (2025) Vol. 9, Iss. 1
Open Access
Homologous recombination deficiency (HRD) testing landscape: clinical applications and technical validation for routine diagnostics
Andréa Witz, Julie Dardare, Margaux Betz, et al.
Biomarker Research (2025) Vol. 13, Iss. 1
Open Access
Andréa Witz, Julie Dardare, Margaux Betz, et al.
Biomarker Research (2025) Vol. 13, Iss. 1
Open Access
Computational pathology for breast cancer: where do we stand for prognostic applications?
Grégoire Gessain, Magali Lacroix‐Triki
The Breast (2025), pp. 104464-104464
Open Access
Grégoire Gessain, Magali Lacroix‐Triki
The Breast (2025), pp. 104464-104464
Open Access
Deep Learning-Based Prediction of Molecular Tumor Biomarkers from H&E: A Practical Review
Heather D. Couture
Journal of Personalized Medicine (2022) Vol. 12, Iss. 12, pp. 2022-2022
Open Access | Times Cited: 18
Heather D. Couture
Journal of Personalized Medicine (2022) Vol. 12, Iss. 12, pp. 2022-2022
Open Access | Times Cited: 18
Direct prediction of Homologous Recombination Deficiency from routine histology in ten different tumor types with attention-based Multiple Instance Learning: a development and validation study
Chiara Maria Lavinia Loeffler, Omar S.M. El Nahhas, Hannah Sophie Muti, et al.
medRxiv (Cold Spring Harbor Laboratory) (2023)
Open Access | Times Cited: 9
Chiara Maria Lavinia Loeffler, Omar S.M. El Nahhas, Hannah Sophie Muti, et al.
medRxiv (Cold Spring Harbor Laboratory) (2023)
Open Access | Times Cited: 9
Current HRD assays in ovarian cancer: differences, pitfalls, limitations, and novel approaches
Federica Guffanti, Ilaria Mengoli, Giovanna Damia
Frontiers in Oncology (2024) Vol. 14
Open Access | Times Cited: 3
Federica Guffanti, Ilaria Mengoli, Giovanna Damia
Frontiers in Oncology (2024) Vol. 14
Open Access | Times Cited: 3
Prediction of homologous recombination deficiency from routine histology with attention-based multiple instance learning in nine different tumor types
Chiara Maria Lavinia Loeffler, Omar S.M. El Nahhas, Hannah Sophie Muti, et al.
BMC Biology (2024) Vol. 22, Iss. 1
Open Access | Times Cited: 3
Chiara Maria Lavinia Loeffler, Omar S.M. El Nahhas, Hannah Sophie Muti, et al.
BMC Biology (2024) Vol. 22, Iss. 1
Open Access | Times Cited: 3
Generative adversarial networks accurately reconstruct pan-cancer histology from pathologic, genomic, and radiographic latent features
Frederick M. Howard, Hanna M. Hieromnimon, Siddhi Ramesh, et al.
Science Advances (2024) Vol. 10, Iss. 46
Open Access | Times Cited: 3
Frederick M. Howard, Hanna M. Hieromnimon, Siddhi Ramesh, et al.
Science Advances (2024) Vol. 10, Iss. 46
Open Access | Times Cited: 3
Deep Learning Prediction of TERT Promoter Mutation Status in Thyroid Cancer Using Histologic Images
Jinhee Kim, Seokhwan Ko, Moonsik Kim, et al.
Medicina (2023) Vol. 59, Iss. 3, pp. 536-536
Open Access | Times Cited: 7
Jinhee Kim, Seokhwan Ko, Moonsik Kim, et al.
Medicina (2023) Vol. 59, Iss. 3, pp. 536-536
Open Access | Times Cited: 7