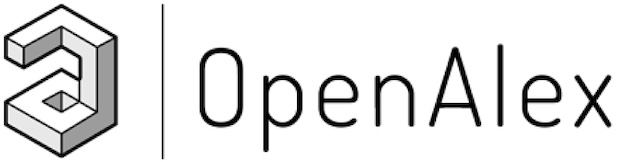
OpenAlex is a bibliographic catalogue of scientific papers, authors and institutions accessible in open access mode, named after the Library of Alexandria. It's citation coverage is excellent and I hope you will find utility in this listing of citing articles!
If you click the article title, you'll navigate to the article, as listed in CrossRef. If you click the Open Access links, you'll navigate to the "best Open Access location". Clicking the citation count will open this listing for that article. Lastly at the bottom of the page, you'll find basic pagination options.
Requested Article:
Accelerating hydrodynamic simulations of urban drainage systems with physics-guided machine learning
Rocco Palmitessa, Morten Grum, Allan P. Engsig‐Karup, et al.
Water Research (2022) Vol. 223, pp. 118972-118972
Open Access | Times Cited: 22
Rocco Palmitessa, Morten Grum, Allan P. Engsig‐Karup, et al.
Water Research (2022) Vol. 223, pp. 118972-118972
Open Access | Times Cited: 22
Showing 22 citing articles:
Generalizing rapid flood predictions to unseen urban catchments with conditional generative adversarial networks
César Ambrogi Ferreira do Lago, Marcio H. Giacomoni, Roberto Bentivoglio, et al.
Journal of Hydrology (2023) Vol. 618, pp. 129276-129276
Open Access | Times Cited: 48
César Ambrogi Ferreira do Lago, Marcio H. Giacomoni, Roberto Bentivoglio, et al.
Journal of Hydrology (2023) Vol. 618, pp. 129276-129276
Open Access | Times Cited: 48
How accurate are the machine learning models in improving monthly rainfall prediction in hyper arid environment?
Faisal Baig, Luqman Ali, Muhammad Abrar Faiz, et al.
Journal of Hydrology (2024) Vol. 633, pp. 131040-131040
Closed Access | Times Cited: 24
Faisal Baig, Luqman Ali, Muhammad Abrar Faiz, et al.
Journal of Hydrology (2024) Vol. 633, pp. 131040-131040
Closed Access | Times Cited: 24
Tool for fast assessment of stormwater flood volumes for urban catchment: A machine learning approach
Bartosz Szeląg, Dariusz Majerek, Anna Laura Eusebi, et al.
Journal of Environmental Management (2024) Vol. 355, pp. 120214-120214
Closed Access | Times Cited: 11
Bartosz Szeląg, Dariusz Majerek, Anna Laura Eusebi, et al.
Journal of Environmental Management (2024) Vol. 355, pp. 120214-120214
Closed Access | Times Cited: 11
A Temporal Fusion Transformer Model to Forecast Overflow from Sewer Manholes during Pluvial Flash Flood Events
Benjamin Burrichter, Juliana Koltermann da Silva, André Niemann, et al.
Hydrology (2024) Vol. 11, Iss. 3, pp. 41-41
Open Access | Times Cited: 5
Benjamin Burrichter, Juliana Koltermann da Silva, André Niemann, et al.
Hydrology (2024) Vol. 11, Iss. 3, pp. 41-41
Open Access | Times Cited: 5
A surrogate machine learning modeling approach for enhancing the efficiency of urban flood modeling at metropolitan scales
Fatemeh Rezaei Aderyani, Keighobad Jafarzadegan, Hamid Moradkhani
Sustainable Cities and Society (2025) Vol. 123, pp. 106277-106277
Closed Access
Fatemeh Rezaei Aderyani, Keighobad Jafarzadegan, Hamid Moradkhani
Sustainable Cities and Society (2025) Vol. 123, pp. 106277-106277
Closed Access
Operator learning for urban water clarification hydrodynamics and particulate matter transport with physics-informed neural networks
Haochen Li, Mohamed Shatarah
Water Research (2024) Vol. 251, pp. 121123-121123
Closed Access | Times Cited: 4
Haochen Li, Mohamed Shatarah
Water Research (2024) Vol. 251, pp. 121123-121123
Closed Access | Times Cited: 4
Multi-Criteria Assessment of Flood Risk on Railroads Using a Machine Learning Approach: A Case Study of Railroads in Minas Gerais
Fernanda Oliveira de Sousa, Victor Andre Ariza Flores, Christhian Santana Cunha, et al.
Infrastructures (2025) Vol. 10, Iss. 1, pp. 12-12
Open Access
Fernanda Oliveira de Sousa, Victor Andre Ariza Flores, Christhian Santana Cunha, et al.
Infrastructures (2025) Vol. 10, Iss. 1, pp. 12-12
Open Access
Multi-scale hydraulic graph neural networks for flood modelling
Roberto Bentivoglio, Elvin Isufi, Sebastiaan Nicolas Jonkman, et al.
Natural hazards and earth system sciences (2025) Vol. 25, Iss. 1, pp. 335-351
Open Access
Roberto Bentivoglio, Elvin Isufi, Sebastiaan Nicolas Jonkman, et al.
Natural hazards and earth system sciences (2025) Vol. 25, Iss. 1, pp. 335-351
Open Access
SWMManywhere: A Workflow for Generation and Sensitivity Analysis of Synthetic Urban Drainage Models, Anywhere
Barnaby Dobson, Tijana Jovanovic, Diego Alonso-Álvarez, et al.
Environmental Modelling & Software (2025) Vol. 186, pp. 106358-106358
Closed Access
Barnaby Dobson, Tijana Jovanovic, Diego Alonso-Álvarez, et al.
Environmental Modelling & Software (2025) Vol. 186, pp. 106358-106358
Closed Access
On the accuracy requirement of surrogate models for adequate global sensitivity analysis of urban low-impact development model
Yi Ke, Pan Yang, Siyuan Yang, et al.
Journal of Hydrology (2025), pp. 133102-133102
Closed Access
Yi Ke, Pan Yang, Siyuan Yang, et al.
Journal of Hydrology (2025), pp. 133102-133102
Closed Access
Interpretable Time Series Models for Wastewater Modeling in Combined Sewer Overflows
Teodor Chiaburu, Felix Bießmann
Procedia Computer Science (2024) Vol. 237, pp. 155-162
Open Access | Times Cited: 2
Teodor Chiaburu, Felix Bießmann
Procedia Computer Science (2024) Vol. 237, pp. 155-162
Open Access | Times Cited: 2
Transferable and data efficient metamodeling of storm water system nodal depths using auto-regressive graph neural networks
Alexander Garzón, Zoran Kapelan, Jeroen Langeveld, et al.
Water Research (2024) Vol. 266, pp. 122396-122396
Open Access | Times Cited: 2
Alexander Garzón, Zoran Kapelan, Jeroen Langeveld, et al.
Water Research (2024) Vol. 266, pp. 122396-122396
Open Access | Times Cited: 2
Predicting the Urban Stormwater Drainage System State using the Graph-WaveNet
Mengru Li, Xiaoming Shi, Zhongming Lu, et al.
Sustainable Cities and Society (2024), pp. 105877-105877
Closed Access | Times Cited: 2
Mengru Li, Xiaoming Shi, Zhongming Lu, et al.
Sustainable Cities and Society (2024), pp. 105877-105877
Closed Access | Times Cited: 2
Concepts and evolution of urban hydrology
Tim D. Fletcher, Matthew J. Burns, Kathryn Russell, et al.
Nature Reviews Earth & Environment (2024) Vol. 5, Iss. 11, pp. 789-801
Closed Access | Times Cited: 2
Tim D. Fletcher, Matthew J. Burns, Kathryn Russell, et al.
Nature Reviews Earth & Environment (2024) Vol. 5, Iss. 11, pp. 789-801
Closed Access | Times Cited: 2
Graph neural network-based surrogate modelling for real-time hydraulic prediction of urban drainage networks
Zhiyu Zhang, Wenchong Tian, Chenkaixiang Lu, et al.
Water Research (2024) Vol. 263, pp. 122142-122142
Open Access | Times Cited: 1
Zhiyu Zhang, Wenchong Tian, Chenkaixiang Lu, et al.
Water Research (2024) Vol. 263, pp. 122142-122142
Open Access | Times Cited: 1
Efficient Hydrodynamic Modelling of Urban Stormwater Systems for Real-Time Applications
Henry Baumann, Nanna Høegh Ravn, Alexander Schaum
Modelling—International Open Access Journal of Modelling in Engineering Science (2022) Vol. 3, Iss. 4, pp. 464-480
Open Access | Times Cited: 7
Henry Baumann, Nanna Høegh Ravn, Alexander Schaum
Modelling—International Open Access Journal of Modelling in Engineering Science (2022) Vol. 3, Iss. 4, pp. 464-480
Open Access | Times Cited: 7
A novel safe-fail framework for the design of urban stormwater drainage infrastructures with minimal failure and flood severity
A. N. Rohith, K. P. Sudheer
Journal of Hydrology (2023) Vol. 627, pp. 130393-130393
Closed Access | Times Cited: 3
A. N. Rohith, K. P. Sudheer
Journal of Hydrology (2023) Vol. 627, pp. 130393-130393
Closed Access | Times Cited: 3
ELM-Based Approach for Analyzing One-Dimensional Electro-osmotic Consolidation
Shaohong Li, Shiguo Xiao
Environmental science and engineering (2024), pp. 653-667
Closed Access
Shaohong Li, Shiguo Xiao
Environmental science and engineering (2024), pp. 653-667
Closed Access
Accelerating Urban Drainage Simulations: A Data-Efficient GNN Metamodel for SWMM Flowrates
Alexander Garzón, Zoran Kapelan, Jeroen Langeveld, et al.
(2024) Vol. 58, pp. 137-137
Open Access
Alexander Garzón, Zoran Kapelan, Jeroen Langeveld, et al.
(2024) Vol. 58, pp. 137-137
Open Access
Fully automated simplification of urban drainage models on a city scale
Markus Pichler, A. Konig, Stefan Reinstaller, et al.
Water Science & Technology (2024) Vol. 90, Iss. 9, pp. 2673-2695
Open Access
Markus Pichler, A. Konig, Stefan Reinstaller, et al.
Water Science & Technology (2024) Vol. 90, Iss. 9, pp. 2673-2695
Open Access
Enhancing Real-Time Urban Drainage Network Modeling through Crossformer Algorithm and Online Continual Learning
Siyi Wang, Jiaying Wang, Kunlun Xin, et al.
Water Research (2024) Vol. 268, pp. 122614-122614
Closed Access
Siyi Wang, Jiaying Wang, Kunlun Xin, et al.
Water Research (2024) Vol. 268, pp. 122614-122614
Closed Access