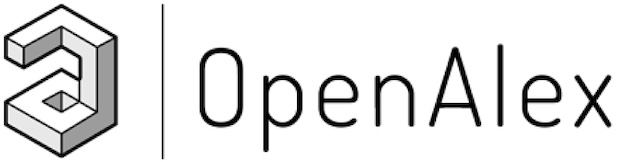
OpenAlex is a bibliographic catalogue of scientific papers, authors and institutions accessible in open access mode, named after the Library of Alexandria. It's citation coverage is excellent and I hope you will find utility in this listing of citing articles!
If you click the article title, you'll navigate to the article, as listed in CrossRef. If you click the Open Access links, you'll navigate to the "best Open Access location". Clicking the citation count will open this listing for that article. Lastly at the bottom of the page, you'll find basic pagination options.
Requested Article:
A critical evaluation of machine learning and deep learning in shield-ground interaction prediction
Pin Zhang, Huai-Na Wu, Renpeng Chen, et al.
Tunnelling and Underground Space Technology (2020) Vol. 106, pp. 103593-103593
Closed Access | Times Cited: 124
Pin Zhang, Huai-Na Wu, Renpeng Chen, et al.
Tunnelling and Underground Space Technology (2020) Vol. 106, pp. 103593-103593
Closed Access | Times Cited: 124
Showing 1-25 of 124 citing articles:
Application of deep learning algorithms in geotechnical engineering: a short critical review
Wengang Zhang, Hongrui Li, Yongqin Li, et al.
Artificial Intelligence Review (2021) Vol. 54, Iss. 8, pp. 5633-5673
Closed Access | Times Cited: 388
Wengang Zhang, Hongrui Li, Yongqin Li, et al.
Artificial Intelligence Review (2021) Vol. 54, Iss. 8, pp. 5633-5673
Closed Access | Times Cited: 388
A VMD-EWT-LSTM-based multi-step prediction approach for shield tunneling machine cutterhead torque
Gang Shi, Chengjin Qin, Jianfeng Tao, et al.
Knowledge-Based Systems (2021) Vol. 228, pp. 107213-107213
Closed Access | Times Cited: 104
Gang Shi, Chengjin Qin, Jianfeng Tao, et al.
Knowledge-Based Systems (2021) Vol. 228, pp. 107213-107213
Closed Access | Times Cited: 104
Prediction of geological characteristics from shield operational parameters by integrating grid search and K-fold cross validation into stacking classification algorithm
Tao Yan, Shui‐Long Shen, Annan Zhou, et al.
Journal of Rock Mechanics and Geotechnical Engineering (2022) Vol. 14, Iss. 4, pp. 1292-1303
Closed Access | Times Cited: 100
Tao Yan, Shui‐Long Shen, Annan Zhou, et al.
Journal of Rock Mechanics and Geotechnical Engineering (2022) Vol. 14, Iss. 4, pp. 1292-1303
Closed Access | Times Cited: 100
Investigation of feature contribution to shield tunneling-induced settlement using Shapley additive explanations method
K. K. Pabodha M. Kannangara, Wan‐Huan Zhou, Zhi Ding, et al.
Journal of Rock Mechanics and Geotechnical Engineering (2022) Vol. 14, Iss. 4, pp. 1052-1063
Open Access | Times Cited: 94
K. K. Pabodha M. Kannangara, Wan‐Huan Zhou, Zhi Ding, et al.
Journal of Rock Mechanics and Geotechnical Engineering (2022) Vol. 14, Iss. 4, pp. 1052-1063
Open Access | Times Cited: 94
Real-time prediction of shield moving trajectory during tunnelling
Shui‐Long Shen, Khalid Elbaz, Wafaa Mohamed Shaban, et al.
Acta Geotechnica (2022) Vol. 17, Iss. 4, pp. 1533-1549
Closed Access | Times Cited: 83
Shui‐Long Shen, Khalid Elbaz, Wafaa Mohamed Shaban, et al.
Acta Geotechnica (2022) Vol. 17, Iss. 4, pp. 1533-1549
Closed Access | Times Cited: 83
Deep learning analysis for energy consumption of shield tunneling machine drive system
Khalid Elbaz, Tao Yan, Annan Zhou, et al.
Tunnelling and Underground Space Technology (2022) Vol. 123, pp. 104405-104405
Closed Access | Times Cited: 79
Khalid Elbaz, Tao Yan, Annan Zhou, et al.
Tunnelling and Underground Space Technology (2022) Vol. 123, pp. 104405-104405
Closed Access | Times Cited: 79
Data-driven multi-output prediction for TBM performance during tunnel excavation: An attention-based graph convolutional network approach
Yue Pan, Xianlei Fu, Limao Zhang
Automation in Construction (2022) Vol. 141, pp. 104386-104386
Closed Access | Times Cited: 70
Yue Pan, Xianlei Fu, Limao Zhang
Automation in Construction (2022) Vol. 141, pp. 104386-104386
Closed Access | Times Cited: 70
Deep learning technologies for shield tunneling: Challenges and opportunities
Cheng Zhou, Yuyue Gao, Elton J. Chen, et al.
Automation in Construction (2023) Vol. 154, pp. 104982-104982
Closed Access | Times Cited: 54
Cheng Zhou, Yuyue Gao, Elton J. Chen, et al.
Automation in Construction (2023) Vol. 154, pp. 104982-104982
Closed Access | Times Cited: 54
Physics-informed deep learning method for predicting tunnelling-induced ground deformations
Zilong Zhang, Qiujing Pan, Zihan Yang, et al.
Acta Geotechnica (2023) Vol. 18, Iss. 9, pp. 4957-4972
Closed Access | Times Cited: 50
Zilong Zhang, Qiujing Pan, Zihan Yang, et al.
Acta Geotechnica (2023) Vol. 18, Iss. 9, pp. 4957-4972
Closed Access | Times Cited: 50
Deep learning-based prediction of steady surface settlement due to shield tunnelling
Wang Gan, Qian Fang, Jianming Du, et al.
Automation in Construction (2023) Vol. 154, pp. 105006-105006
Closed Access | Times Cited: 41
Wang Gan, Qian Fang, Jianming Du, et al.
Automation in Construction (2023) Vol. 154, pp. 105006-105006
Closed Access | Times Cited: 41
Modelling the performance of EPB shield tunnelling using machine and deep learning algorithms
Song-Shun Lin, Shui‐Long Shen, Ning Zhang, et al.
Geoscience Frontiers (2021) Vol. 12, Iss. 5, pp. 101177-101177
Open Access | Times Cited: 78
Song-Shun Lin, Shui‐Long Shen, Ning Zhang, et al.
Geoscience Frontiers (2021) Vol. 12, Iss. 5, pp. 101177-101177
Open Access | Times Cited: 78
Tunnel boring machine vibration-based deep learning for the ground identification of working faces
Mengbo Liu, Shaoming Liao, Yifeng Yang, et al.
Journal of Rock Mechanics and Geotechnical Engineering (2021) Vol. 13, Iss. 6, pp. 1340-1357
Open Access | Times Cited: 71
Mengbo Liu, Shaoming Liao, Yifeng Yang, et al.
Journal of Rock Mechanics and Geotechnical Engineering (2021) Vol. 13, Iss. 6, pp. 1340-1357
Open Access | Times Cited: 71
Spatio-temporal feature fusion for real-time prediction of TBM operating parameters: A deep learning approach
Xianlei Fu, Limao Zhang
Automation in Construction (2021) Vol. 132, pp. 103937-103937
Closed Access | Times Cited: 67
Xianlei Fu, Limao Zhang
Automation in Construction (2021) Vol. 132, pp. 103937-103937
Closed Access | Times Cited: 67
Success and challenges in predicting TBM penetration rate using recurrent neural networks
Feng Shan, Xuzhen He, Danial Jahed Armaghani, et al.
Tunnelling and Underground Space Technology (2022) Vol. 130, pp. 104728-104728
Closed Access | Times Cited: 66
Feng Shan, Xuzhen He, Danial Jahed Armaghani, et al.
Tunnelling and Underground Space Technology (2022) Vol. 130, pp. 104728-104728
Closed Access | Times Cited: 66
Time-series prediction of shield movement performance during tunneling based on hybrid model
Song-Shun Lin, Ning Zhang, Annan Zhou, et al.
Tunnelling and Underground Space Technology (2021) Vol. 119, pp. 104245-104245
Closed Access | Times Cited: 62
Song-Shun Lin, Ning Zhang, Annan Zhou, et al.
Tunnelling and Underground Space Technology (2021) Vol. 119, pp. 104245-104245
Closed Access | Times Cited: 62
Significance and methodology: Preprocessing the big data for machine learning on TBM performance
Haohan Xiao, Wen‐Kun Yang, Jing Hu, et al.
Underground Space (2022) Vol. 7, Iss. 4, pp. 680-701
Open Access | Times Cited: 52
Haohan Xiao, Wen‐Kun Yang, Jing Hu, et al.
Underground Space (2022) Vol. 7, Iss. 4, pp. 680-701
Open Access | Times Cited: 52
A new index for cutter life evaluation and ensemble model for prediction of cutter wear
Nan Zhang, Shui‐Long Shen, Annan Zhou
Tunnelling and Underground Space Technology (2022) Vol. 131, pp. 104830-104830
Closed Access | Times Cited: 50
Nan Zhang, Shui‐Long Shen, Annan Zhou
Tunnelling and Underground Space Technology (2022) Vol. 131, pp. 104830-104830
Closed Access | Times Cited: 50
Optimization of high-performance concrete mix ratio design using machine learning
Bin Chen, Lei Wang, Zongbao Feng, et al.
Engineering Applications of Artificial Intelligence (2023) Vol. 122, pp. 106047-106047
Closed Access | Times Cited: 33
Bin Chen, Lei Wang, Zongbao Feng, et al.
Engineering Applications of Artificial Intelligence (2023) Vol. 122, pp. 106047-106047
Closed Access | Times Cited: 33
Integrated parameter optimization approach: Just-in-time (JIT) operational control strategy for TBM tunnelling
Xiaojun Li, Sicheng Zhao, Yi Shen, et al.
Tunnelling and Underground Space Technology (2023) Vol. 135, pp. 105040-105040
Closed Access | Times Cited: 30
Xiaojun Li, Sicheng Zhao, Yi Shen, et al.
Tunnelling and Underground Space Technology (2023) Vol. 135, pp. 105040-105040
Closed Access | Times Cited: 30
Dynamic and explainable deep learning-based risk prediction on adjacent building induced by deep excavation
Xuyang Li, Yue Pan, Limao Zhang, et al.
Tunnelling and Underground Space Technology (2023) Vol. 140, pp. 105243-105243
Closed Access | Times Cited: 29
Xuyang Li, Yue Pan, Limao Zhang, et al.
Tunnelling and Underground Space Technology (2023) Vol. 140, pp. 105243-105243
Closed Access | Times Cited: 29
Dynamic prediction for attitude and position of shield machine in tunneling: A hybrid deep learning method considering dual attention
Zeyu Dai, Peinan Li, Mengqi Zhu, et al.
Advanced Engineering Informatics (2023) Vol. 57, pp. 102032-102032
Closed Access | Times Cited: 28
Zeyu Dai, Peinan Li, Mengqi Zhu, et al.
Advanced Engineering Informatics (2023) Vol. 57, pp. 102032-102032
Closed Access | Times Cited: 28
Prediction of jacking force using PSO-BPNN and PSO-SVR algorithm in curved pipe roof
Hao Zhou, Sheng Huang, Peng Zhang, et al.
Tunnelling and Underground Space Technology (2023) Vol. 138, pp. 105159-105159
Closed Access | Times Cited: 27
Hao Zhou, Sheng Huang, Peng Zhang, et al.
Tunnelling and Underground Space Technology (2023) Vol. 138, pp. 105159-105159
Closed Access | Times Cited: 27
Effects of data smoothing and recurrent neural network (RNN) algorithms for real-time forecasting of tunnel boring machine (TBM) performance
Feng Shan, Xuzhen He, Danial Jahed Armaghani, et al.
Journal of Rock Mechanics and Geotechnical Engineering (2023) Vol. 16, Iss. 5, pp. 1538-1551
Open Access | Times Cited: 27
Feng Shan, Xuzhen He, Danial Jahed Armaghani, et al.
Journal of Rock Mechanics and Geotechnical Engineering (2023) Vol. 16, Iss. 5, pp. 1538-1551
Open Access | Times Cited: 27
TBM performance prediction using LSTM-based hybrid neural network model: Case study of Baimang River tunnel project in Shenzhen, China
Qihang Xu, Xin Huang, Baogang Zhang, et al.
Underground Space (2023) Vol. 11, pp. 130-152
Open Access | Times Cited: 22
Qihang Xu, Xin Huang, Baogang Zhang, et al.
Underground Space (2023) Vol. 11, pp. 130-152
Open Access | Times Cited: 22
A spatiotemporal deep learning method for excavation-induced wall deflections
Yuanqin Tao, Shaoxiang Zeng, Honglei Sun, et al.
Journal of Rock Mechanics and Geotechnical Engineering (2024) Vol. 16, Iss. 8, pp. 3327-3338
Open Access | Times Cited: 11
Yuanqin Tao, Shaoxiang Zeng, Honglei Sun, et al.
Journal of Rock Mechanics and Geotechnical Engineering (2024) Vol. 16, Iss. 8, pp. 3327-3338
Open Access | Times Cited: 11