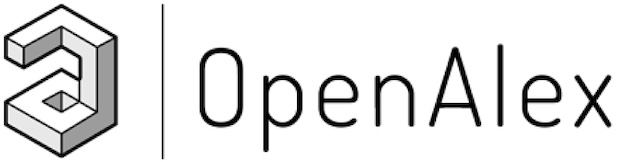
OpenAlex is a bibliographic catalogue of scientific papers, authors and institutions accessible in open access mode, named after the Library of Alexandria. It's citation coverage is excellent and I hope you will find utility in this listing of citing articles!
If you click the article title, you'll navigate to the article, as listed in CrossRef. If you click the Open Access links, you'll navigate to the "best Open Access location". Clicking the citation count will open this listing for that article. Lastly at the bottom of the page, you'll find basic pagination options.
Requested Article:
Tribological properties study and prediction of PTFE composites based on experiments and machine learning
Qihua Wang, Xiaoyue Wang, Xinrui Zhang, et al.
Tribology International (2023) Vol. 188, pp. 108815-108815
Closed Access | Times Cited: 14
Qihua Wang, Xiaoyue Wang, Xinrui Zhang, et al.
Tribology International (2023) Vol. 188, pp. 108815-108815
Closed Access | Times Cited: 14
Showing 14 citing articles:
Machine Learning-Based Prediction of Tribological Properties of Epoxy Composite Coating
Han Yan, Tan Junling, Hui Chen, et al.
Polymers (2025) Vol. 17, Iss. 3, pp. 282-282
Open Access | Times Cited: 1
Han Yan, Tan Junling, Hui Chen, et al.
Polymers (2025) Vol. 17, Iss. 3, pp. 282-282
Open Access | Times Cited: 1
Sustainable Revolution: AI-Driven Enhancements for Composite Polymer Processing and Optimization in Intelligent Food Packaging
Sana Yakoubi
Food and Bioprocess Technology (2024)
Closed Access | Times Cited: 7
Sana Yakoubi
Food and Bioprocess Technology (2024)
Closed Access | Times Cited: 7
Analysis and prediction of erosion behavior of epoxy composites using statistical and machine learning techniques
Sourav Kumar Mahapatra, Alok Satapathy
Proceedings of the Institution of Mechanical Engineers Part E Journal of Process Mechanical Engineering (2024)
Closed Access | Times Cited: 6
Sourav Kumar Mahapatra, Alok Satapathy
Proceedings of the Institution of Mechanical Engineers Part E Journal of Process Mechanical Engineering (2024)
Closed Access | Times Cited: 6
Tribological properties study and prediction of QBe2 beryllium bronze and 7075-T6 aluminum alloys based on machine learning under mixed lubrication
Z.Y. Li, Lijie Qiao, Jiaqi Li, et al.
Proceedings of the Institution of Mechanical Engineers Part J Journal of Engineering Tribology (2025)
Closed Access
Z.Y. Li, Lijie Qiao, Jiaqi Li, et al.
Proceedings of the Institution of Mechanical Engineers Part J Journal of Engineering Tribology (2025)
Closed Access
Prediction of Tribological Properties of PC ‐PBT /GNP ‐MWCNT Nanocomposites Using Machine Learning Models
Tuba Özdemi̇r Öge, Mecit Öge
Journal of Applied Polymer Science (2025)
Open Access
Tuba Özdemi̇r Öge, Mecit Öge
Journal of Applied Polymer Science (2025)
Open Access
Prediction of the Tribological Properties of Polytetrafluoroethylene Composites Based on Experiments and Machine Learning
Yingnan Yan, Jiliang Du, Shiwei Ren, et al.
Polymers (2024) Vol. 16, Iss. 3, pp. 356-356
Open Access | Times Cited: 3
Yingnan Yan, Jiliang Du, Shiwei Ren, et al.
Polymers (2024) Vol. 16, Iss. 3, pp. 356-356
Open Access | Times Cited: 3
A data-driven approach for studying tribology based on experimentation and artificial intelligence coupling tools
Mohamed Kchaou
Sustainable Engineering and Innovation ISSN 2712-0562 (2024) Vol. 6, Iss. 1, pp. 25-36
Open Access | Times Cited: 3
Mohamed Kchaou
Sustainable Engineering and Innovation ISSN 2712-0562 (2024) Vol. 6, Iss. 1, pp. 25-36
Open Access | Times Cited: 3
A machine learning-driven approach to predicting thermo-elasto-hydrodynamic lubrication in journal bearings
Samuel A. Cartwright, Benjamin Rothwell, Grazziela P. Figueredo, et al.
Tribology International (2024) Vol. 196, pp. 109670-109670
Open Access | Times Cited: 3
Samuel A. Cartwright, Benjamin Rothwell, Grazziela P. Figueredo, et al.
Tribology International (2024) Vol. 196, pp. 109670-109670
Open Access | Times Cited: 3
Tribological investigation into nickel-coated graphite polytetrafluoroethylene composites
YP Deepthi, Santosh Kumar Sahu, D. Anitha, et al.
Proceedings of the Institution of Mechanical Engineers Part E Journal of Process Mechanical Engineering (2024)
Closed Access | Times Cited: 3
YP Deepthi, Santosh Kumar Sahu, D. Anitha, et al.
Proceedings of the Institution of Mechanical Engineers Part E Journal of Process Mechanical Engineering (2024)
Closed Access | Times Cited: 3
Prediction of Wear Rate of Glass-Filled PTFE Composites Based on Machine Learning Approaches
Abhijeet Deshpande, Atul Kulkarni, Namrata N. Wasatkar, et al.
Polymers (2024) Vol. 16, Iss. 18, pp. 2666-2666
Open Access | Times Cited: 3
Abhijeet Deshpande, Atul Kulkarni, Namrata N. Wasatkar, et al.
Polymers (2024) Vol. 16, Iss. 18, pp. 2666-2666
Open Access | Times Cited: 3
Synergetic Enhancement of Wear Resistance of Polyimide Coatings through the Integration of MoS2 Nanoflowers and MXene Nanosheets
Jiayuan Yan, Chunxia Wu, Yongxing Liu, et al.
Wear (2024) Vol. 558-559, pp. 205584-205584
Closed Access | Times Cited: 2
Jiayuan Yan, Chunxia Wu, Yongxing Liu, et al.
Wear (2024) Vol. 558-559, pp. 205584-205584
Closed Access | Times Cited: 2
Tribological Analysis of Ti6Al4V Using Machine Learning to Determine Coefficient of Friction and Wear Rate
İsmail Enes Parlak, Cantekin Kaykılarlı, Taha Yasin Eken
Research Square (Research Square) (2024)
Open Access
İsmail Enes Parlak, Cantekin Kaykılarlı, Taha Yasin Eken
Research Square (Research Square) (2024)
Open Access
Prediction of the Tribological Properties of Graphite-Filled Ptfe Composites Based on Machine Learning Algorithms
Huifeng Ning, Wenwen WANG, Gui Gao, et al.
(2024)
Closed Access
Huifeng Ning, Wenwen WANG, Gui Gao, et al.
(2024)
Closed Access
Advanced Machine Learning and Experimental Studies of Polypropylene Based Polyesters Tribological Composite Systems for Sustainable Recycling Automation and Digitalization
Abrar Hussain, Jakob Kübarsepp, Fjodor Sergejev, et al.
International Journal of Lightweight Materials and Manufacture (2024)
Open Access
Abrar Hussain, Jakob Kübarsepp, Fjodor Sergejev, et al.
International Journal of Lightweight Materials and Manufacture (2024)
Open Access