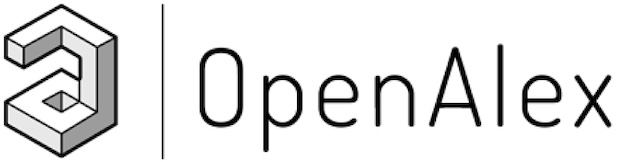
OpenAlex is a bibliographic catalogue of scientific papers, authors and institutions accessible in open access mode, named after the Library of Alexandria. It's citation coverage is excellent and I hope you will find utility in this listing of citing articles!
If you click the article title, you'll navigate to the article, as listed in CrossRef. If you click the Open Access links, you'll navigate to the "best Open Access location". Clicking the citation count will open this listing for that article. Lastly at the bottom of the page, you'll find basic pagination options.
Requested Article:
Tribological performance study and prediction of copper coated by MoS2 based on GBRT method
Guoqing Wang, Yuling Ruan, Hongxing Wang, et al.
Tribology International (2022) Vol. 179, pp. 108149-108149
Closed Access | Times Cited: 24
Guoqing Wang, Yuling Ruan, Hongxing Wang, et al.
Tribology International (2022) Vol. 179, pp. 108149-108149
Closed Access | Times Cited: 24
Showing 24 citing articles:
AI for tribology: Present and future
Nian Yin, Pufan Yang, Songkai Liu, et al.
Friction (2024) Vol. 12, Iss. 6, pp. 1060-1097
Open Access | Times Cited: 18
Nian Yin, Pufan Yang, Songkai Liu, et al.
Friction (2024) Vol. 12, Iss. 6, pp. 1060-1097
Open Access | Times Cited: 18
2D materials for Tribo-corrosion and -oxidation protection: A review
Sangharatna M. Ramteke, Magdalena Walczak, Marco De Stefano, et al.
Advances in Colloid and Interface Science (2024) Vol. 331, pp. 103243-103243
Open Access | Times Cited: 18
Sangharatna M. Ramteke, Magdalena Walczak, Marco De Stefano, et al.
Advances in Colloid and Interface Science (2024) Vol. 331, pp. 103243-103243
Open Access | Times Cited: 18
Revealing the anti-friction mechanism of in-situ synthesized MoS2-S nanocomposite coating under different shear stress
Zehui Yang, Bingkun Ning, Yongnan Chen, et al.
Tribology International (2024) Vol. 195, pp. 109587-109587
Closed Access | Times Cited: 8
Zehui Yang, Bingkun Ning, Yongnan Chen, et al.
Tribology International (2024) Vol. 195, pp. 109587-109587
Closed Access | Times Cited: 8
Modeling the line contact on an elastic half-space with the statistical approach: Self-affine fractal roughness and numerical framework
Tao Yang, Hanmin Peng, Xiongfeng Tang, et al.
Tribology International (2024) Vol. 194, pp. 109527-109527
Closed Access | Times Cited: 6
Tao Yang, Hanmin Peng, Xiongfeng Tang, et al.
Tribology International (2024) Vol. 194, pp. 109527-109527
Closed Access | Times Cited: 6
Tribological properties and machine learning prediction of FeCoCrNiAlN high entropy coatings
Binyuan Jia, Qiang Wan, Long Yan, et al.
Surface and Coatings Technology (2023) Vol. 477, pp. 130341-130341
Closed Access | Times Cited: 16
Binyuan Jia, Qiang Wan, Long Yan, et al.
Surface and Coatings Technology (2023) Vol. 477, pp. 130341-130341
Closed Access | Times Cited: 16
Tribological properties study and prediction of PTFE composites based on experiments and machine learning
Qihua Wang, Xiaoyue Wang, Xinrui Zhang, et al.
Tribology International (2023) Vol. 188, pp. 108815-108815
Closed Access | Times Cited: 14
Qihua Wang, Xiaoyue Wang, Xinrui Zhang, et al.
Tribology International (2023) Vol. 188, pp. 108815-108815
Closed Access | Times Cited: 14
Harnessing Machine Learning to Predict MoS2 Solid Lubricant Performance
Dayton J. Vogel, Tomas F. Babuska, Alexander Mings, et al.
Tribology Letters (2025) Vol. 73, Iss. 1
Closed Access
Dayton J. Vogel, Tomas F. Babuska, Alexander Mings, et al.
Tribology Letters (2025) Vol. 73, Iss. 1
Closed Access
Tribological properties study and prediction of QBe2 beryllium bronze and 7075-T6 aluminum alloys based on machine learning under mixed lubrication
Z.Y. Li, Lijie Qiao, Jiaqi Li, et al.
Proceedings of the Institution of Mechanical Engineers Part J Journal of Engineering Tribology (2025)
Closed Access
Z.Y. Li, Lijie Qiao, Jiaqi Li, et al.
Proceedings of the Institution of Mechanical Engineers Part J Journal of Engineering Tribology (2025)
Closed Access
Machine Learning-Based Prediction of Tribological Properties of Epoxy Composite Coating
Han Yan, Tan Junling, Hui Chen, et al.
Polymers (2025) Vol. 17, Iss. 3, pp. 282-282
Open Access
Han Yan, Tan Junling, Hui Chen, et al.
Polymers (2025) Vol. 17, Iss. 3, pp. 282-282
Open Access
Multiple temporal scale variation characteristics and driving factors of arid inland runoff: A case study of Urumqi River, China
Kun Liu, Yunfei Chen, Bin Wu, et al.
Journal of Hydrology Regional Studies (2025) Vol. 58, pp. 102298-102298
Open Access
Kun Liu, Yunfei Chen, Bin Wu, et al.
Journal of Hydrology Regional Studies (2025) Vol. 58, pp. 102298-102298
Open Access
Temperature-Driven Tribological Behavior of MoS₂ Coatings on Copper: Experimental and Molecular Dynamics Insights
Guoqing Wang, Tianqiang Yin, Tao Yang, et al.
Materials Today Communications (2025), pp. 112537-112537
Closed Access
Guoqing Wang, Tianqiang Yin, Tao Yang, et al.
Materials Today Communications (2025), pp. 112537-112537
Closed Access
Prediction of the Tribological Properties of Polytetrafluoroethylene Composites Based on Experiments and Machine Learning
Yingnan Yan, Jiliang Du, Shiwei Ren, et al.
Polymers (2024) Vol. 16, Iss. 3, pp. 356-356
Open Access | Times Cited: 3
Yingnan Yan, Jiliang Du, Shiwei Ren, et al.
Polymers (2024) Vol. 16, Iss. 3, pp. 356-356
Open Access | Times Cited: 3
Enhanced wear resistance of 304 stainless steel by an improved method based on ultrasonic strengthening grinding process and PTFE coating
Jinrui Xiao, Yan Hui Liu, Jianhui Wang, et al.
Construction and Building Materials (2024) Vol. 431, pp. 136598-136598
Closed Access | Times Cited: 3
Jinrui Xiao, Yan Hui Liu, Jianhui Wang, et al.
Construction and Building Materials (2024) Vol. 431, pp. 136598-136598
Closed Access | Times Cited: 3
Can machine learning predict friction from third body morphology?
Alizée Bouchot, Amandine Ferrieux, Johan Debayle, et al.
Tribology International (2024) Vol. 193, pp. 109361-109361
Open Access | Times Cited: 2
Alizée Bouchot, Amandine Ferrieux, Johan Debayle, et al.
Tribology International (2024) Vol. 193, pp. 109361-109361
Open Access | Times Cited: 2
Machine Learning Composite-Nanoparticle-Enriched Lubricant Oil Development for Improved Frictional Performance—An Experiment
Ali Usman, Saad Arif, Ahmed Hassan Raja, et al.
Lubricants (2023) Vol. 11, Iss. 6, pp. 254-254
Open Access | Times Cited: 4
Ali Usman, Saad Arif, Ahmed Hassan Raja, et al.
Lubricants (2023) Vol. 11, Iss. 6, pp. 254-254
Open Access | Times Cited: 4
Surface and interface mechanical properties of inorganic nanolayers
Chia-Wei Huang, Tang-Yu Lai, Te‐Hua Fang
Japanese Journal of Applied Physics (2024) Vol. 63, Iss. 3, pp. 03SP09-03SP09
Open Access | Times Cited: 1
Chia-Wei Huang, Tang-Yu Lai, Te‐Hua Fang
Japanese Journal of Applied Physics (2024) Vol. 63, Iss. 3, pp. 03SP09-03SP09
Open Access | Times Cited: 1
Physics-Informed machine learning for tribological properties prediction of S32750/CFRPEEK tribopair under seawater lubrication via PISSA-CNN-LSTM
Fanglong Yin, Hao Luo, Songlin Nie, et al.
Tribology International (2024) Vol. 199, pp. 109965-109965
Closed Access | Times Cited: 1
Fanglong Yin, Hao Luo, Songlin Nie, et al.
Tribology International (2024) Vol. 199, pp. 109965-109965
Closed Access | Times Cited: 1
Prediction of Daily Water Consumption in Residential Areas Based on Meteorologic Conditions—Applying Gradient Boosting Regression Tree Algorithm
Zhengxuan Li, Sen Peng, Zheng Guolei, et al.
Water (2023) Vol. 15, Iss. 19, pp. 3455-3455
Open Access | Times Cited: 2
Zhengxuan Li, Sen Peng, Zheng Guolei, et al.
Water (2023) Vol. 15, Iss. 19, pp. 3455-3455
Open Access | Times Cited: 2
Predicting friction coefficient of textured 45# steel based on machine learning and analytical calculation
Zhenshun Li, Jiaqi Li, Ben An, et al.
Industrial Lubrication and Tribology (2024)
Closed Access
Zhenshun Li, Jiaqi Li, Ben An, et al.
Industrial Lubrication and Tribology (2024)
Closed Access
Prediction of the Tribological Properties of Graphite-Filled Ptfe Composites Based on Machine Learning Algorithms
Huifeng Ning, Wenwen WANG, Gui Gao, et al.
(2024)
Closed Access
Huifeng Ning, Wenwen WANG, Gui Gao, et al.
(2024)
Closed Access
Integration of machine learning prediction and optimization for determination of the coefficient of friction of textured UHMWPE surfaces
Huihui Feng, Jing Liu, Ron A.J. van Ostayen, et al.
Proceedings of the Institution of Mechanical Engineers Part J Journal of Engineering Tribology (2024)
Closed Access
Huihui Feng, Jing Liu, Ron A.J. van Ostayen, et al.
Proceedings of the Institution of Mechanical Engineers Part J Journal of Engineering Tribology (2024)
Closed Access
Tribological Properties of MoN (Ag‐W)‐MoS2 (W) Multilayer Films in Wide Temperature Range
Gensheng Wu, Fulei Song, Jianjun Xie, et al.
Advanced Engineering Materials (2024)
Closed Access
Gensheng Wu, Fulei Song, Jianjun Xie, et al.
Advanced Engineering Materials (2024)
Closed Access
Crystalline Carbon Deposition on Piston Ring Substrates and Its Effects on Tribological Characteristics, Engine Performance, and Emissions
Mehmet Esen, Ali Can Yılmaz
Advanced Engineering Materials (2024)
Closed Access
Mehmet Esen, Ali Can Yılmaz
Advanced Engineering Materials (2024)
Closed Access
INVESTIGATING WEAR OF PIN FABRICATED BY PRESSURE-LESS SINTERING OF COPPER POWDER INTO 3D PRINTED CLAY MOULDS
Harshit K. Dave, Savan Fefa
International Journal of Modern Manufacturing Technologies (2024) Vol. 16, Iss. 2, pp. 89-96
Closed Access
Harshit K. Dave, Savan Fefa
International Journal of Modern Manufacturing Technologies (2024) Vol. 16, Iss. 2, pp. 89-96
Closed Access