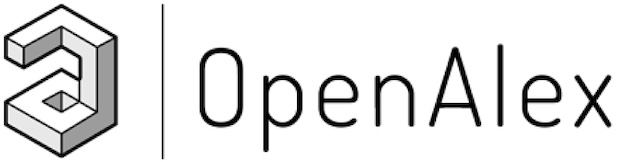
OpenAlex is a bibliographic catalogue of scientific papers, authors and institutions accessible in open access mode, named after the Library of Alexandria. It's citation coverage is excellent and I hope you will find utility in this listing of citing articles!
If you click the article title, you'll navigate to the article, as listed in CrossRef. If you click the Open Access links, you'll navigate to the "best Open Access location". Clicking the citation count will open this listing for that article. Lastly at the bottom of the page, you'll find basic pagination options.
Requested Article:
Comparison of tree-based machine learning algorithms for predicting liquefaction potential using canonical correlation forest, rotation forest, and random forest based on CPT data
Selçuk Demir, Emrehan Kutluğ Şahin
Soil Dynamics and Earthquake Engineering (2021) Vol. 154, pp. 107130-107130
Closed Access | Times Cited: 55
Selçuk Demir, Emrehan Kutluğ Şahin
Soil Dynamics and Earthquake Engineering (2021) Vol. 154, pp. 107130-107130
Closed Access | Times Cited: 55
Showing 1-25 of 55 citing articles:
An investigation of feature selection methods for soil liquefaction prediction based on tree-based ensemble algorithms using AdaBoost, gradient boosting, and XGBoost
Selçuk Demir, Emrehan Kutluğ Şahin
Neural Computing and Applications (2022) Vol. 35, Iss. 4, pp. 3173-3190
Closed Access | Times Cited: 78
Selçuk Demir, Emrehan Kutluğ Şahin
Neural Computing and Applications (2022) Vol. 35, Iss. 4, pp. 3173-3190
Closed Access | Times Cited: 78
Explainable machine learning model for liquefaction potential assessment of soils using XGBoost-SHAP
Kaushik Jas, G. R. Dodagoudar
Soil Dynamics and Earthquake Engineering (2022) Vol. 165, pp. 107662-107662
Closed Access | Times Cited: 77
Kaushik Jas, G. R. Dodagoudar
Soil Dynamics and Earthquake Engineering (2022) Vol. 165, pp. 107662-107662
Closed Access | Times Cited: 77
A Survey of Decision Trees: Concepts, Algorithms, and Applications
Ibomoiye Domor Mienye, Nobert Jere
IEEE Access (2024) Vol. 12, pp. 86716-86727
Open Access | Times Cited: 30
Ibomoiye Domor Mienye, Nobert Jere
IEEE Access (2024) Vol. 12, pp. 86716-86727
Open Access | Times Cited: 30
Evaluation and analysis of liquefaction potential of gravelly soils using explainable probabilistic machine learning model
Kaushik Jas, Sujith Mangalathu, G. R. Dodagoudar
Computers and Geotechnics (2024) Vol. 167, pp. 106051-106051
Closed Access | Times Cited: 19
Kaushik Jas, Sujith Mangalathu, G. R. Dodagoudar
Computers and Geotechnics (2024) Vol. 167, pp. 106051-106051
Closed Access | Times Cited: 19
Liquefaction prediction with robust machine learning algorithms (SVM, RF, and XGBoost) supported by genetic algorithm-based feature selection and parameter optimization from the perspective of data processing
Selçuk Demir, Emrehan Kutluğ Şahin
Environmental Earth Sciences (2022) Vol. 81, Iss. 18
Closed Access | Times Cited: 57
Selçuk Demir, Emrehan Kutluğ Şahin
Environmental Earth Sciences (2022) Vol. 81, Iss. 18
Closed Access | Times Cited: 57
Tree-Based Machine Learning Models with Optuna in Predicting Impedance Values for Circuit Analysis
Jung-Pin Lai, Ying-Lei Lin, Ho-Chuan Lin, et al.
Micromachines (2023) Vol. 14, Iss. 2, pp. 265-265
Open Access | Times Cited: 29
Jung-Pin Lai, Ying-Lei Lin, Ho-Chuan Lin, et al.
Micromachines (2023) Vol. 14, Iss. 2, pp. 265-265
Open Access | Times Cited: 29
Deep learning and big data mining for Metal–Organic frameworks with high performance for simultaneous desulfurization and carbon capture
Kexin Guan, Fangyi Xu, Xiaoshan Huang, et al.
Journal of Colloid and Interface Science (2024) Vol. 662, pp. 941-952
Closed Access | Times Cited: 10
Kexin Guan, Fangyi Xu, Xiaoshan Huang, et al.
Journal of Colloid and Interface Science (2024) Vol. 662, pp. 941-952
Closed Access | Times Cited: 10
Advanced tree-based machine learning methods for predicting the seismic response of regular and irregular RC frames
Ahmet Demir, Emrehan Kutluğ Şahin, Selçuk Demir
Structures (2024) Vol. 64, pp. 106524-106524
Closed Access | Times Cited: 7
Ahmet Demir, Emrehan Kutluğ Şahin, Selçuk Demir
Structures (2024) Vol. 64, pp. 106524-106524
Closed Access | Times Cited: 7
Implementation of free and open-source semi-automatic feature engineering tool in landslide susceptibility mapping using the machine-learning algorithms RF, SVM, and XGBoost
Emrehan Kutluğ Şahin
Stochastic Environmental Research and Risk Assessment (2022) Vol. 37, Iss. 3, pp. 1067-1092
Closed Access | Times Cited: 29
Emrehan Kutluğ Şahin
Stochastic Environmental Research and Risk Assessment (2022) Vol. 37, Iss. 3, pp. 1067-1092
Closed Access | Times Cited: 29
Modeling and predicting the sensitivity of high-performance concrete compressive strength using machine learning methods
Walaa Hussein Al Yamani, Dalin Mohammad Ghunimat, Majdi Bisharah
Asian Journal of Civil Engineering (2023) Vol. 24, Iss. 7, pp. 1943-1955
Closed Access | Times Cited: 19
Walaa Hussein Al Yamani, Dalin Mohammad Ghunimat, Majdi Bisharah
Asian Journal of Civil Engineering (2023) Vol. 24, Iss. 7, pp. 1943-1955
Closed Access | Times Cited: 19
Conjunct application of machine learning and game theory in groundwater quality mapping
Ali Nasiri Khiavi, Mohammad Tavoosi, Alban Kuriqi
Environmental Earth Sciences (2023) Vol. 82, Iss. 17
Open Access | Times Cited: 17
Ali Nasiri Khiavi, Mohammad Tavoosi, Alban Kuriqi
Environmental Earth Sciences (2023) Vol. 82, Iss. 17
Open Access | Times Cited: 17
Data-driven intelligent modeling of unconfined compressive strength of heavy metal-contaminated soil
Syed Taseer Abbas Jaffar, Xiangsheng Chen, Xiaohua Bao, et al.
Journal of Rock Mechanics and Geotechnical Engineering (2024)
Open Access | Times Cited: 6
Syed Taseer Abbas Jaffar, Xiangsheng Chen, Xiaohua Bao, et al.
Journal of Rock Mechanics and Geotechnical Engineering (2024)
Open Access | Times Cited: 6
Cone penetration test-based assessment of liquefaction potential using machine and hybrid learning approaches
Jitendra Khatti, Yewuhalashet Fissha, Kamaldeep Singh Grover, et al.
Multiscale and Multidisciplinary Modeling Experiments and Design (2024) Vol. 7, Iss. 4, pp. 3841-3864
Closed Access | Times Cited: 5
Jitendra Khatti, Yewuhalashet Fissha, Kamaldeep Singh Grover, et al.
Multiscale and Multidisciplinary Modeling Experiments and Design (2024) Vol. 7, Iss. 4, pp. 3841-3864
Closed Access | Times Cited: 5
Reducing product waste within the retail industry: a post-COVID-19 era study on enhancing demand prediction with hybrid prediction models
Maider Elorza, Sara Segura Querol, Eduardo Castellano
Applied Economics (2025), pp. 1-16
Closed Access
Maider Elorza, Sara Segura Querol, Eduardo Castellano
Applied Economics (2025), pp. 1-16
Closed Access
Greedy-AutoML: A novel greedy-based stacking ensemble learning framework for assessing soil liquefaction potential
Emrehan Kutluğ Şahin, Selçuk Demir
Engineering Applications of Artificial Intelligence (2022) Vol. 119, pp. 105732-105732
Closed Access | Times Cited: 26
Emrehan Kutluğ Şahin, Selçuk Demir
Engineering Applications of Artificial Intelligence (2022) Vol. 119, pp. 105732-105732
Closed Access | Times Cited: 26
Experimental and intelligent modelling for predicting the amplitude of footing resting on geocell-reinforced soil bed under vibratory load
Hasthi Venkateswarlu, Muhammad Nouman Amjad Raja, A. Hegde, et al.
Transportation Geotechnics (2022) Vol. 35, pp. 100783-100783
Closed Access | Times Cited: 25
Hasthi Venkateswarlu, Muhammad Nouman Amjad Raja, A. Hegde, et al.
Transportation Geotechnics (2022) Vol. 35, pp. 100783-100783
Closed Access | Times Cited: 25
Bayesian Network Ensemble Models Applied to Seismic Liquefaction Prediction Based on Different In-situ Test Databases
Wenjun Zou, Jilei Hu
Applied Soft Computing (2025), pp. 112668-112668
Closed Access
Wenjun Zou, Jilei Hu
Applied Soft Computing (2025), pp. 112668-112668
Closed Access
Estimation of soil liquefaction using artificial intelligence techniques: an extended comparison between machine and deep learning approaches
Eyyüp Hakan Şehmusoğlu, Талас Фикрет Курназ, Caner Erden
Environmental Earth Sciences (2025) Vol. 84, Iss. 5
Open Access
Eyyüp Hakan Şehmusoğlu, Талас Фикрет Курназ, Caner Erden
Environmental Earth Sciences (2025) Vol. 84, Iss. 5
Open Access
Interpretable Soil Liquefaction Prediction with Genetic Programming Derived Closed-Form Models
Laith Sadik, Sara Khoshnevisan
Geotechnical Frontiers 2017 (2025), pp. 59-66
Closed Access
Laith Sadik, Sara Khoshnevisan
Geotechnical Frontiers 2017 (2025), pp. 59-66
Closed Access
A critical performance assessment of the machine learning algorithms for slope stability analysis
Satyam Tiwari, Sarat Kumar Das, Madhumita Mohanty
Neural Computing and Applications (2025)
Closed Access
Satyam Tiwari, Sarat Kumar Das, Madhumita Mohanty
Neural Computing and Applications (2025)
Closed Access
Application of machine learning in early warning system of geotechnical disaster: a systematic and comprehensive review
Shan Lin, Zenglong Liang, Hongwei Guo, et al.
Artificial Intelligence Review (2025) Vol. 58, Iss. 6
Open Access
Shan Lin, Zenglong Liang, Hongwei Guo, et al.
Artificial Intelligence Review (2025) Vol. 58, Iss. 6
Open Access
Combining data envelopment analysis and Random Forest for selecting optimal locations of solar PV plants
Gilles Cattani
Energy and AI (2022) Vol. 11, pp. 100222-100222
Open Access | Times Cited: 20
Gilles Cattani
Energy and AI (2022) Vol. 11, pp. 100222-100222
Open Access | Times Cited: 20
Predicting building damage grade by earthquake: a Bayesian Optimization-based comparative study of machine learning algorithms
Mohammad Al-Rawashdeh, Mohammad Al-Nawaiseh, Isam Yousef, et al.
Asian Journal of Civil Engineering (2023) Vol. 25, Iss. 1, pp. 253-264
Closed Access | Times Cited: 11
Mohammad Al-Rawashdeh, Mohammad Al-Nawaiseh, Isam Yousef, et al.
Asian Journal of Civil Engineering (2023) Vol. 25, Iss. 1, pp. 253-264
Closed Access | Times Cited: 11
Why “AI” models for predicting soil liquefaction have been ignored, plus some that shouldn’t be
Brett W. Maurer, Morgan Sanger
Earthquake Spectra (2023) Vol. 39, Iss. 3, pp. 1883-1910
Closed Access | Times Cited: 10
Brett W. Maurer, Morgan Sanger
Earthquake Spectra (2023) Vol. 39, Iss. 3, pp. 1883-1910
Closed Access | Times Cited: 10
Analysis of Data Splitting on Streamflow Prediction using Random Forest
Diksha Puri, Parveen Sihag, Mohindra Singh Thakur, et al.
AIMS environmental science (2024) Vol. 11, Iss. 4, pp. 593-609
Open Access | Times Cited: 3
Diksha Puri, Parveen Sihag, Mohindra Singh Thakur, et al.
AIMS environmental science (2024) Vol. 11, Iss. 4, pp. 593-609
Open Access | Times Cited: 3