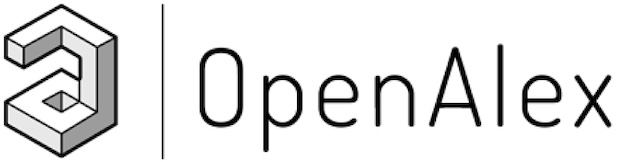
OpenAlex is a bibliographic catalogue of scientific papers, authors and institutions accessible in open access mode, named after the Library of Alexandria. It's citation coverage is excellent and I hope you will find utility in this listing of citing articles!
If you click the article title, you'll navigate to the article, as listed in CrossRef. If you click the Open Access links, you'll navigate to the "best Open Access location". Clicking the citation count will open this listing for that article. Lastly at the bottom of the page, you'll find basic pagination options.
Requested Article:
Prediction of cadmium contents in rice grains from Quaternary sediment-distributed farmland using field investigations and machine learning
Xudong Ma, Tao Yu, Dong‐Xing Guan, et al.
The Science of The Total Environment (2023) Vol. 898, pp. 165482-165482
Closed Access | Times Cited: 17
Xudong Ma, Tao Yu, Dong‐Xing Guan, et al.
The Science of The Total Environment (2023) Vol. 898, pp. 165482-165482
Closed Access | Times Cited: 17
Showing 17 citing articles:
Cadmium accumulation in paddy soils affected by geological weathering and mining: Spatial distribution patterns, bioaccumulation prediction, and safe land usage
Cheng Li, Zhongfang Yang, Tao Yu, et al.
Journal of Hazardous Materials (2023) Vol. 460, pp. 132483-132483
Closed Access | Times Cited: 17
Cheng Li, Zhongfang Yang, Tao Yu, et al.
Journal of Hazardous Materials (2023) Vol. 460, pp. 132483-132483
Closed Access | Times Cited: 17
Utilizing machine learning to evaluate heavy metal pollution in the world's largest mangrove forest
Ram Proshad, Md. Abdur Rahim, Mahfuzur Rahman, et al.
The Science of The Total Environment (2024) Vol. 951, pp. 175746-175746
Closed Access | Times Cited: 5
Ram Proshad, Md. Abdur Rahim, Mahfuzur Rahman, et al.
The Science of The Total Environment (2024) Vol. 951, pp. 175746-175746
Closed Access | Times Cited: 5
Driving factors of soil selenium accumulation in regional enrichment area at selenium-deficient soil belt of China: An enlightenment of Moran's index and machine learning
Yuanli Qin, Li Zhang, Qiaolin Wang, et al.
Journal of Geochemical Exploration (2025), pp. 107746-107746
Closed Access
Yuanli Qin, Li Zhang, Qiaolin Wang, et al.
Journal of Geochemical Exploration (2025), pp. 107746-107746
Closed Access
Improved estimation of soil organic carbon stock in subtropical cropland of Southern China based on digital soil mapping and multi-sources data
Bifeng Hu, Qian Zhu, Modian Xie, et al.
Geo-spatial Information Science (2025), pp. 1-24
Open Access
Bifeng Hu, Qian Zhu, Modian Xie, et al.
Geo-spatial Information Science (2025), pp. 1-24
Open Access
New Advances, Challenges, and Illustrations in Applied Geochemistry
Qingjie Gong, Zeming Shi
Applied Sciences (2025) Vol. 15, Iss. 6, pp. 3407-3407
Open Access
Qingjie Gong, Zeming Shi
Applied Sciences (2025) Vol. 15, Iss. 6, pp. 3407-3407
Open Access
Predicting the Zinc Content in Rice from Farmland Using Machine Learning Models: Insights from Universal Geochemical Parameters
Wenda Geng, Tingting Li, Xin Zhu, et al.
Applied Sciences (2025) Vol. 15, Iss. 3, pp. 1273-1273
Open Access
Wenda Geng, Tingting Li, Xin Zhu, et al.
Applied Sciences (2025) Vol. 15, Iss. 3, pp. 1273-1273
Open Access
Prediction of soil-rice selenium transfer by machine learning
Ying Wang, Junliang Cai, Zhongfang Yang
Journal of Soils and Sediments (2025)
Closed Access
Ying Wang, Junliang Cai, Zhongfang Yang
Journal of Soils and Sediments (2025)
Closed Access
Spatial-machine learning framework for rapid identification of soil cadmium risk in high geochemical background areas
Cheng Li, Zhongfang Yang, Dong‐Xing Guan, et al.
Journal of Hazardous Materials (2025), pp. 138091-138091
Closed Access
Cheng Li, Zhongfang Yang, Dong‐Xing Guan, et al.
Journal of Hazardous Materials (2025), pp. 138091-138091
Closed Access
Application of machine learning methods for predicting selenium accumulation in the soil‒rice system of a typical karst area
Molan Tang, Bolun Fan, LU Guang-hui, et al.
Journal of Soils and Sediments (2025)
Closed Access
Molan Tang, Bolun Fan, LU Guang-hui, et al.
Journal of Soils and Sediments (2025)
Closed Access
Decoupling soil pH and geography: Universal drivers of cadmium bioavailability in rice across terrains
Qiang Liu, Shuyue Liu, Danling Wang, et al.
Journal of Environmental Management (2025) Vol. 381, pp. 125297-125297
Closed Access
Qiang Liu, Shuyue Liu, Danling Wang, et al.
Journal of Environmental Management (2025) Vol. 381, pp. 125297-125297
Closed Access
Machine learning-based prediction of cadmium pollution in topsoil and identification of critical driving factors in a mining area
Cheng Li, Zhongcheng Jiang, Wenli Li, et al.
Environmental Geochemistry and Health (2024) Vol. 46, Iss. 9
Closed Access | Times Cited: 1
Cheng Li, Zhongcheng Jiang, Wenli Li, et al.
Environmental Geochemistry and Health (2024) Vol. 46, Iss. 9
Closed Access | Times Cited: 1
Enhanced food authenticity control using machine learning-assisted elemental analysis
Ying Yang, Lu Zhang, Xinquan Qu, et al.
Food Research International (2024) Vol. 198, pp. 115330-115330
Closed Access | Times Cited: 1
Ying Yang, Lu Zhang, Xinquan Qu, et al.
Food Research International (2024) Vol. 198, pp. 115330-115330
Closed Access | Times Cited: 1
Ensemble learning-assisted quantitative identifying influencing factors of cadmium and arsenic concentration in rice grain based multiplexed data
Yakun Wang, Zhuo Zhang, Cheng Cheng, et al.
Journal of Hazardous Materials (2024) Vol. 485, pp. 136869-136869
Closed Access | Times Cited: 1
Yakun Wang, Zhuo Zhang, Cheng Cheng, et al.
Journal of Hazardous Materials (2024) Vol. 485, pp. 136869-136869
Closed Access | Times Cited: 1
An ensemble framework for farmland quality evaluation based on machine learning and physical models
Weixuan Xian, Hang Liu, Xingjian Yang, et al.
The Science of The Total Environment (2023) Vol. 912, pp. 168914-168914
Closed Access | Times Cited: 2
Weixuan Xian, Hang Liu, Xingjian Yang, et al.
The Science of The Total Environment (2023) Vol. 912, pp. 168914-168914
Closed Access | Times Cited: 2
Prediction of Maize Copper Content in Copper-Poor Area Based on Machine Learning
Husheng Zhang, Linshu Hu, Zhongfang Yang, et al.
(2024)
Open Access
Husheng Zhang, Linshu Hu, Zhongfang Yang, et al.
(2024)
Open Access
Machine Learning and Structural Equation Modeling for Revealing the Influence Factors and Pathways of Different Water Management Regimes Acting on Brown Rice Cadmium
Yingxia Liu, Jinchuan Ma, Junjie Chu, et al.
(2024)
Closed Access
Yingxia Liu, Jinchuan Ma, Junjie Chu, et al.
(2024)
Closed Access
Prediction of maize copper content in copper-poor area based on machine learning
Husheng Zhang, Linshu Hu, Zhongfang Yang, et al.
Research Square (Research Square) (2024)
Open Access
Husheng Zhang, Linshu Hu, Zhongfang Yang, et al.
Research Square (Research Square) (2024)
Open Access