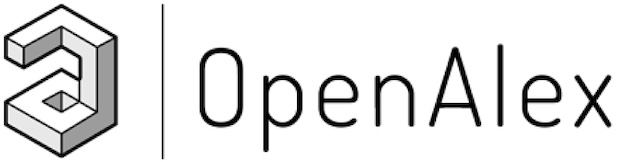
OpenAlex is a bibliographic catalogue of scientific papers, authors and institutions accessible in open access mode, named after the Library of Alexandria. It's citation coverage is excellent and I hope you will find utility in this listing of citing articles!
If you click the article title, you'll navigate to the article, as listed in CrossRef. If you click the Open Access links, you'll navigate to the "best Open Access location". Clicking the citation count will open this listing for that article. Lastly at the bottom of the page, you'll find basic pagination options.
Requested Article:
MoDL-QSM: Model-based deep learning for quantitative susceptibility mapping
Ruimin Feng, Jiayi Zhao, He Wang, et al.
NeuroImage (2021) Vol. 240, pp. 118376-118376
Open Access | Times Cited: 35
Ruimin Feng, Jiayi Zhao, He Wang, et al.
NeuroImage (2021) Vol. 240, pp. 118376-118376
Open Access | Times Cited: 35
Showing 1-25 of 35 citing articles:
SwinGAN: A dual-domain Swin Transformer-based generative adversarial network for MRI reconstruction
Xiang Zhao, Tiejun Yang, Bingjie Li, et al.
Computers in Biology and Medicine (2022) Vol. 153, pp. 106513-106513
Closed Access | Times Cited: 60
Xiang Zhao, Tiejun Yang, Bingjie Li, et al.
Computers in Biology and Medicine (2022) Vol. 153, pp. 106513-106513
Closed Access | Times Cited: 60
Improving quantitative MRI using self‐supervised deep learning with model reinforcement: Demonstration for rapid T1 mapping
Wanyu Bian, Albert Jang, Fang Liu
Magnetic Resonance in Medicine (2024) Vol. 92, Iss. 1, pp. 98-111
Closed Access | Times Cited: 12
Wanyu Bian, Albert Jang, Fang Liu
Magnetic Resonance in Medicine (2024) Vol. 92, Iss. 1, pp. 98-111
Closed Access | Times Cited: 12
Knowledge‐driven deep learning for fast MR imaging: Undersampled MR image reconstruction from supervised to un‐supervised learning
Shanshan Wang, Ruoyou Wu, Sen Jia, et al.
Magnetic Resonance in Medicine (2024) Vol. 92, Iss. 2, pp. 496-518
Open Access | Times Cited: 6
Shanshan Wang, Ruoyou Wu, Sen Jia, et al.
Magnetic Resonance in Medicine (2024) Vol. 92, Iss. 2, pp. 496-518
Open Access | Times Cited: 6
Instant tissue field and magnetic susceptibility mapping from MRI raw phase using Laplacian enhanced deep neural networks
Yang Gao, Zhuang Xiong, Amir Fazlollahi, et al.
NeuroImage (2022) Vol. 259, pp. 119410-119410
Open Access | Times Cited: 19
Yang Gao, Zhuang Xiong, Amir Fazlollahi, et al.
NeuroImage (2022) Vol. 259, pp. 119410-119410
Open Access | Times Cited: 19
Physics-Driven Deep Learning Methods for Fast Quantitative Magnetic Resonance Imaging: Performance improvements through integration with deep neural networks
Yanjie Zhu, Jing Cheng, Zhuo‐Xu Cui, et al.
IEEE Signal Processing Magazine (2023) Vol. 40, Iss. 2, pp. 116-128
Closed Access | Times Cited: 11
Yanjie Zhu, Jing Cheng, Zhuo‐Xu Cui, et al.
IEEE Signal Processing Magazine (2023) Vol. 40, Iss. 2, pp. 116-128
Closed Access | Times Cited: 11
Quantitative susceptibility mapping via deep neural networks with iterative reverse concatenations and recurrent modules
Min Li, Chen Chen, Xiong Zhuang, et al.
Medical Physics (2025)
Closed Access
Min Li, Chen Chen, Xiong Zhuang, et al.
Medical Physics (2025)
Closed Access
Chronic active lesions in multiple sclerosis: classification, terminology, and clinical significance
Assunta Dal‐Bianco, Jiwon Oh, Pascal Sati, et al.
Therapeutic Advances in Neurological Disorders (2024) Vol. 17
Open Access | Times Cited: 3
Assunta Dal‐Bianco, Jiwon Oh, Pascal Sati, et al.
Therapeutic Advances in Neurological Disorders (2024) Vol. 17
Open Access | Times Cited: 3
Plug-and-Play latent feature editing for orientation-adaptive quantitative susceptibility mapping neural networks
Yang Gao, Zhuang Xiong, Shanshan Shan, et al.
Medical Image Analysis (2024) Vol. 94, pp. 103160-103160
Open Access | Times Cited: 2
Yang Gao, Zhuang Xiong, Shanshan Shan, et al.
Medical Image Analysis (2024) Vol. 94, pp. 103160-103160
Open Access | Times Cited: 2
Quantitative susceptibility mapping through model-based deep image prior (MoDIP)
Zhuang Xiong, Yang Gao, Yin Liu, et al.
NeuroImage (2024) Vol. 291, pp. 120583-120583
Open Access | Times Cited: 2
Zhuang Xiong, Yang Gao, Yin Liu, et al.
NeuroImage (2024) Vol. 291, pp. 120583-120583
Open Access | Times Cited: 2
A subject-specific unsupervised deep learning method for quantitative susceptibility mapping using implicit neural representation
Ming Zhang, Ruimin Feng, Zhenghao Li, et al.
Medical Image Analysis (2024) Vol. 95, pp. 103173-103173
Closed Access | Times Cited: 2
Ming Zhang, Ruimin Feng, Zhenghao Li, et al.
Medical Image Analysis (2024) Vol. 95, pp. 103173-103173
Closed Access | Times Cited: 2
Affine transformation edited and refined deep neural network for quantitative susceptibility mapping
Zhuang Xiong, Yang Gao, Feng Liu, et al.
NeuroImage (2022) Vol. 267, pp. 119842-119842
Open Access | Times Cited: 10
Zhuang Xiong, Yang Gao, Feng Liu, et al.
NeuroImage (2022) Vol. 267, pp. 119842-119842
Open Access | Times Cited: 10
Towards in vivo ground truth susceptibility for single-orientation deep learning QSM: A multi-orientation gradient-echo MRI dataset
Yuting Shi, Ruimin Feng, Zhenghao Li, et al.
NeuroImage (2022) Vol. 261, pp. 119522-119522
Open Access | Times Cited: 9
Yuting Shi, Ruimin Feng, Zhenghao Li, et al.
NeuroImage (2022) Vol. 261, pp. 119522-119522
Open Access | Times Cited: 9
A spatially adaptive regularization based three-dimensional reconstruction network for quantitative susceptibility mapping
Lijun Bao, Hongyuan Zhang, Zifeng Liao
Physics in Medicine and Biology (2024) Vol. 69, Iss. 4, pp. 045030-045030
Closed Access | Times Cited: 1
Lijun Bao, Hongyuan Zhang, Zifeng Liao
Physics in Medicine and Biology (2024) Vol. 69, Iss. 4, pp. 045030-045030
Closed Access | Times Cited: 1
Spinet-QSM: model-based deep learning with schatten p-norm regularization for improved quantitative susceptibility mapping
V. Venkatesh, Raji Susan Mathew, Phaneendra K. Yalavarthy
Magnetic Resonance Materials in Physics Biology and Medicine (2024) Vol. 37, Iss. 3, pp. 411-427
Closed Access | Times Cited: 1
V. Venkatesh, Raji Susan Mathew, Phaneendra K. Yalavarthy
Magnetic Resonance Materials in Physics Biology and Medicine (2024) Vol. 37, Iss. 3, pp. 411-427
Closed Access | Times Cited: 1
NeXtQSM—A complete deep learning pipeline for data-consistent Quantitative Susceptibility Mapping trained with hybrid data
Francesco Cognolato, Kieran O’Brien, Jin Jin, et al.
Medical Image Analysis (2022) Vol. 84, pp. 102700-102700
Open Access | Times Cited: 6
Francesco Cognolato, Kieran O’Brien, Jin Jin, et al.
Medical Image Analysis (2022) Vol. 84, pp. 102700-102700
Open Access | Times Cited: 6
msQSM: Morphology-based self-supervised deep learning for quantitative susceptibility mapping
Junjie He, Yunsong Peng, Bangkang Fu, et al.
NeuroImage (2023) Vol. 275, pp. 120181-120181
Open Access | Times Cited: 3
Junjie He, Yunsong Peng, Bangkang Fu, et al.
NeuroImage (2023) Vol. 275, pp. 120181-120181
Open Access | Times Cited: 3
Meta-Learning Based Interactively Connected Clique U-Net for Quantitative Susceptibility Mapping
Zheng Li, Jun Li, Chaoyue Wang, et al.
IEEE Transactions on Computational Imaging (2021) Vol. 7, pp. 1385-1399
Closed Access | Times Cited: 7
Zheng Li, Jun Li, Chaoyue Wang, et al.
IEEE Transactions on Computational Imaging (2021) Vol. 7, pp. 1385-1399
Closed Access | Times Cited: 7
Reconstruction of Quantitative Susceptibility Mapping from Total Field Maps with Local Field Maps Guided UU-Net
Zheng Li, Shihui Ying, Jun Wang, et al.
IEEE Journal of Biomedical and Health Informatics (2023), pp. 1-12
Closed Access | Times Cited: 2
Zheng Li, Shihui Ying, Jun Wang, et al.
IEEE Journal of Biomedical and Health Informatics (2023), pp. 1-12
Closed Access | Times Cited: 2
Quantitative susceptibility mapping using multi-channel convolutional neural networks with dipole-adaptive multi-frequency inputs
Wenbin Si, Yihao Guo, Qianqian Zhang, et al.
Frontiers in Neuroscience (2023) Vol. 17
Open Access | Times Cited: 2
Wenbin Si, Yihao Guo, Qianqian Zhang, et al.
Frontiers in Neuroscience (2023) Vol. 17
Open Access | Times Cited: 2
Magnetic Resonance Parameter Mapping using Self-supervised Deep Learning with Model Reinforcement
Wanyu Bian, Albert Jang, Zhiyuan Liu
arXiv (Cornell University) (2023)
Open Access | Times Cited: 2
Wanyu Bian, Albert Jang, Zhiyuan Liu
arXiv (Cornell University) (2023)
Open Access | Times Cited: 2
Learn Less, Infer More: Learning in the Fourier Domain for Quantitative Susceptibility Mapping
Junjie He, Lihui Wang, Ying Cao, et al.
Frontiers in Neuroscience (2022) Vol. 16
Open Access | Times Cited: 4
Junjie He, Lihui Wang, Ying Cao, et al.
Frontiers in Neuroscience (2022) Vol. 16
Open Access | Times Cited: 4
Incorporating a-priori information in deep learning models for quantitative susceptibility mapping via adaptive convolution
Simon Graf, Walter A. Wohlgemuth, Andreas Deistung
Frontiers in Neuroscience (2024) Vol. 18
Open Access
Simon Graf, Walter A. Wohlgemuth, Andreas Deistung
Frontiers in Neuroscience (2024) Vol. 18
Open Access
ProxiMO: Proximal Multi-operator Networks for Quantitative Susceptibility Mapping
Shmuel Orenstein, Zhenghan Fang, Hyeong‐Geol Shin, et al.
Lecture notes in computer science (2024), pp. 13-23
Closed Access
Shmuel Orenstein, Zhenghan Fang, Hyeong‐Geol Shin, et al.
Lecture notes in computer science (2024), pp. 13-23
Closed Access
NoQSM-net: Combining Convolutional Neural Network With Numerical Optimization Algorithm for Quantitative Susceptibility Mapping Reconstruction
Qianqian Zhang, Yihao Guo, Wufan Chen
IEEE Access (2024) Vol. 12, pp. 33129-33139
Open Access
Qianqian Zhang, Yihao Guo, Wufan Chen
IEEE Access (2024) Vol. 12, pp. 33129-33139
Open Access
DF‐QSM: Data Fidelity based Hybrid Approach for Improved Quantitative Susceptibility Mapping of the Brain
Naveen Paluru, Raji Susan Mathew, Phaneendra K. Yalavarthy
NMR in Biomedicine (2024) Vol. 37, Iss. 9
Closed Access
Naveen Paluru, Raji Susan Mathew, Phaneendra K. Yalavarthy
NMR in Biomedicine (2024) Vol. 37, Iss. 9
Closed Access