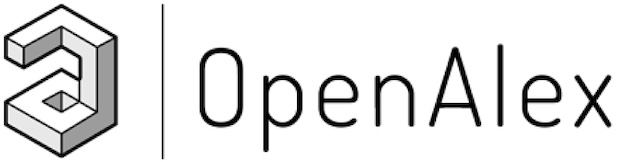
OpenAlex is a bibliographic catalogue of scientific papers, authors and institutions accessible in open access mode, named after the Library of Alexandria. It's citation coverage is excellent and I hope you will find utility in this listing of citing articles!
If you click the article title, you'll navigate to the article, as listed in CrossRef. If you click the Open Access links, you'll navigate to the "best Open Access location". Clicking the citation count will open this listing for that article. Lastly at the bottom of the page, you'll find basic pagination options.
Requested Article:
Integration of deep feature representations and handcrafted features to improve the prediction of N6-methyladenosine sites
Leyi Wei, Ran Su, Bing Wang, et al.
Neurocomputing (2018) Vol. 324, pp. 3-9
Closed Access | Times Cited: 137
Leyi Wei, Ran Su, Bing Wang, et al.
Neurocomputing (2018) Vol. 324, pp. 3-9
Closed Access | Times Cited: 137
Showing 1-25 of 137 citing articles:
Gene2vec: gene subsequence embedding for prediction of mammalian N6-methyladenosine sites from mRNA
Quan Zou, Pengwei Xing, Leyi Wei, et al.
RNA (2018) Vol. 25, Iss. 2, pp. 205-218
Open Access | Times Cited: 464
Quan Zou, Pengwei Xing, Leyi Wei, et al.
RNA (2018) Vol. 25, Iss. 2, pp. 205-218
Open Access | Times Cited: 464
Deep-Resp-Forest: A deep forest model to predict anti-cancer drug response
Ran Su, Xinyi Liu, Leyi Wei, et al.
Methods (2019) Vol. 166, pp. 91-102
Closed Access | Times Cited: 225
Ran Su, Xinyi Liu, Leyi Wei, et al.
Methods (2019) Vol. 166, pp. 91-102
Closed Access | Times Cited: 225
WHISTLE: a high-accuracy map of the human N6-methyladenosine (m6A) epitranscriptome predicted using a machine learning approach
Kunqi Chen, Zhen Wei, Qing Zhang, et al.
Nucleic Acids Research (2019) Vol. 47, Iss. 7, pp. e41-e41
Open Access | Times Cited: 197
Kunqi Chen, Zhen Wei, Qing Zhang, et al.
Nucleic Acids Research (2019) Vol. 47, Iss. 7, pp. e41-e41
Open Access | Times Cited: 197
Clustering and classification methods for single-cell RNA-sequencing data
Ren Qi, Anjun Ma, Qin Ma, et al.
Briefings in Bioinformatics (2019) Vol. 21, Iss. 4, pp. 1196-1208
Open Access | Times Cited: 169
Ren Qi, Anjun Ma, Qin Ma, et al.
Briefings in Bioinformatics (2019) Vol. 21, Iss. 4, pp. 1196-1208
Open Access | Times Cited: 169
mACPpred: A Support Vector Machine-Based Meta-Predictor for Identification of Anticancer Peptides
Vinothini Boopathi, Sathiyamoorthy Subramaniyam, Adeel Malik, et al.
International Journal of Molecular Sciences (2019) Vol. 20, Iss. 8, pp. 1964-1964
Open Access | Times Cited: 167
Vinothini Boopathi, Sathiyamoorthy Subramaniyam, Adeel Malik, et al.
International Journal of Molecular Sciences (2019) Vol. 20, Iss. 8, pp. 1964-1964
Open Access | Times Cited: 167
A feasibility study on an automated method to generate patient‐specific dose distributions for radiotherapy using deep learning
Xinyuan Chen, Kuo Men, Ye‐Xiong Li, et al.
Medical Physics (2018) Vol. 46, Iss. 1, pp. 56-64
Open Access | Times Cited: 165
Xinyuan Chen, Kuo Men, Ye‐Xiong Li, et al.
Medical Physics (2018) Vol. 46, Iss. 1, pp. 56-64
Open Access | Times Cited: 165
iProEP: A Computational Predictor for Predicting Promoter
Hong-Yan Lai, Zhao‐Yue Zhang, Zhendong Su, et al.
Molecular Therapy — Nucleic Acids (2019) Vol. 17, pp. 337-346
Open Access | Times Cited: 152
Hong-Yan Lai, Zhao‐Yue Zhang, Zhendong Su, et al.
Molecular Therapy — Nucleic Acids (2019) Vol. 17, pp. 337-346
Open Access | Times Cited: 152
Anticancer peptides prediction with deep representation learning features
Zhibin Lv, Feifei Cui, Quan Zou, et al.
Briefings in Bioinformatics (2021) Vol. 22, Iss. 5
Closed Access | Times Cited: 113
Zhibin Lv, Feifei Cui, Quan Zou, et al.
Briefings in Bioinformatics (2021) Vol. 22, Iss. 5
Closed Access | Times Cited: 113
Biological Sequence Classification: A Review on Data and General Methods
Chunyan Ao, Shihu Jiao, Yansu Wang, et al.
Research (2022) Vol. 2022
Open Access | Times Cited: 68
Chunyan Ao, Shihu Jiao, Yansu Wang, et al.
Research (2022) Vol. 2022
Open Access | Times Cited: 68
Bitter-RF: A random forest machine model for recognizing bitter peptides
Yufei Zhang, Yuhao Wang, Zhi-Feng Gu, et al.
Frontiers in Medicine (2023) Vol. 10
Open Access | Times Cited: 40
Yufei Zhang, Yuhao Wang, Zhi-Feng Gu, et al.
Frontiers in Medicine (2023) Vol. 10
Open Access | Times Cited: 40
Empirical comparison and analysis of web-based cell-penetrating peptide prediction tools
Ran Su, Jie Hu, Quan Zou, et al.
Briefings in Bioinformatics (2018) Vol. 21, Iss. 2, pp. 408-420
Closed Access | Times Cited: 148
Ran Su, Jie Hu, Quan Zou, et al.
Briefings in Bioinformatics (2018) Vol. 21, Iss. 2, pp. 408-420
Closed Access | Times Cited: 148
Evaluation of different computational methods on 5-methylcytosine sites identification
Hao Lv, Zimei Zhang, Shi-Hao Li, et al.
Briefings in Bioinformatics (2019) Vol. 21, Iss. 3, pp. 982-995
Closed Access | Times Cited: 140
Hao Lv, Zimei Zhang, Shi-Hao Li, et al.
Briefings in Bioinformatics (2019) Vol. 21, Iss. 3, pp. 982-995
Closed Access | Times Cited: 140
Identification of hormone binding proteins based on machine learning methods
Jiu-Xin Tan, Shi-Hao Li, Zimei Zhang, et al.
Mathematical Biosciences & Engineering (2019) Vol. 16, Iss. 4, pp. 2466-2480
Open Access | Times Cited: 134
Jiu-Xin Tan, Shi-Hao Li, Zimei Zhang, et al.
Mathematical Biosciences & Engineering (2019) Vol. 16, Iss. 4, pp. 2466-2480
Open Access | Times Cited: 134
Protein Function Prediction: From Traditional Classifier to Deep Learning
Zhibin Lv, Chunyan Ao, Quan Zou
PROTEOMICS (2019) Vol. 19, Iss. 14
Closed Access | Times Cited: 127
Zhibin Lv, Chunyan Ao, Quan Zou
PROTEOMICS (2019) Vol. 19, Iss. 14
Closed Access | Times Cited: 127
Comprehensive review and assessment of computational methods for predicting RNA post-transcriptional modification sites from RNA sequences
Zhen Chen, Pei Zhao, Fuyi Li, et al.
Briefings in Bioinformatics (2019) Vol. 21, Iss. 5, pp. 1676-1696
Closed Access | Times Cited: 121
Zhen Chen, Pei Zhao, Fuyi Li, et al.
Briefings in Bioinformatics (2019) Vol. 21, Iss. 5, pp. 1676-1696
Closed Access | Times Cited: 121
iGHBP: Computational identification of growth hormone binding proteins from sequences using extremely randomised tree
Shaherin Basith, Balachandran Manavalan, Tae Hwan Shin, et al.
Computational and Structural Biotechnology Journal (2018) Vol. 16, pp. 412-420
Open Access | Times Cited: 111
Shaherin Basith, Balachandran Manavalan, Tae Hwan Shin, et al.
Computational and Structural Biotechnology Journal (2018) Vol. 16, pp. 412-420
Open Access | Times Cited: 111
Convolutional neural network-based annotation of bacterial type IV secretion system effectors with enhanced accuracy and reduced false discovery
Jiajun Hong, Yongchao Luo, Minjie Mou, et al.
Briefings in Bioinformatics (2019) Vol. 21, Iss. 5, pp. 1825-1836
Closed Access | Times Cited: 107
Jiajun Hong, Yongchao Luo, Minjie Mou, et al.
Briefings in Bioinformatics (2019) Vol. 21, Iss. 5, pp. 1825-1836
Closed Access | Times Cited: 107
A Random Forest Sub-Golgi Protein Classifier Optimized via Dipeptide and Amino Acid Composition Features
Zhibin Lv, Shunshan Jin, Hui Ding, et al.
Frontiers in Bioengineering and Biotechnology (2019) Vol. 7
Open Access | Times Cited: 106
Zhibin Lv, Shunshan Jin, Hui Ding, et al.
Frontiers in Bioengineering and Biotechnology (2019) Vol. 7
Open Access | Times Cited: 106
M6AMRFS: Robust Prediction of N6-Methyladenosine Sites With Sequence-Based Features in Multiple Species
Xiaoli Qiang, Huangrong Chen, Xiucai Ye, et al.
Frontiers in Genetics (2018) Vol. 9
Open Access | Times Cited: 104
Xiaoli Qiang, Huangrong Chen, Xiucai Ye, et al.
Frontiers in Genetics (2018) Vol. 9
Open Access | Times Cited: 104
DeepFunc: A Deep Learning Framework for Accurate Prediction of Protein Functions from Protein Sequences and Interactions
Fuhao Zhang, Hong Song, Min Zeng, et al.
PROTEOMICS (2019) Vol. 19, Iss. 12
Closed Access | Times Cited: 93
Fuhao Zhang, Hong Song, Min Zeng, et al.
PROTEOMICS (2019) Vol. 19, Iss. 12
Closed Access | Times Cited: 93
k-Skip-n-Gram-RF: A Random Forest Based Method for Alzheimer's Disease Protein Identification
Lei Xu, Guangmin Liang, Changrui Liao, et al.
Frontiers in Genetics (2019) Vol. 10
Open Access | Times Cited: 76
Lei Xu, Guangmin Liang, Changrui Liao, et al.
Frontiers in Genetics (2019) Vol. 10
Open Access | Times Cited: 76
Prediction of bio-sequence modifications and the associations with diseases
Chunyan Ao, Liang Yu, Quan Zou
Briefings in Functional Genomics (2020) Vol. 20, Iss. 1, pp. 1-18
Closed Access | Times Cited: 72
Chunyan Ao, Liang Yu, Quan Zou
Briefings in Functional Genomics (2020) Vol. 20, Iss. 1, pp. 1-18
Closed Access | Times Cited: 72
A convolutional neural network approach for IMRT dose distribution prediction in prostate cancer patients
Tomohiro Kajikawa, Noriyuki Kadoya, Kengo Ito, et al.
Journal of Radiation Research (2019) Vol. 60, Iss. 5, pp. 685-693
Open Access | Times Cited: 61
Tomohiro Kajikawa, Noriyuki Kadoya, Kengo Ito, et al.
Journal of Radiation Research (2019) Vol. 60, Iss. 5, pp. 685-693
Open Access | Times Cited: 61
Fold-LTR-TCP: protein fold recognition based on triadic closure principle
Bin Liu, Yulin Zhu, Ke Yan
Briefings in Bioinformatics (2019) Vol. 21, Iss. 6, pp. 2185-2193
Closed Access | Times Cited: 61
Bin Liu, Yulin Zhu, Ke Yan
Briefings in Bioinformatics (2019) Vol. 21, Iss. 6, pp. 2185-2193
Closed Access | Times Cited: 61
A comprehensive comparison and analysis of computational predictors for RNA N6-methyladenosine sites of Saccharomyces cerevisiae
Xiaolei Zhu, Jingjing He, Shihao Zhao, et al.
Briefings in Functional Genomics (2019)
Closed Access | Times Cited: 60
Xiaolei Zhu, Jingjing He, Shihao Zhao, et al.
Briefings in Functional Genomics (2019)
Closed Access | Times Cited: 60