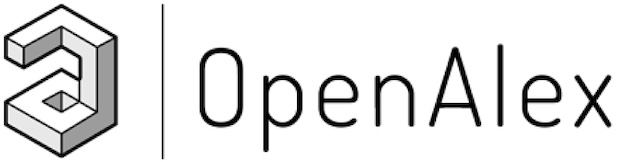
OpenAlex is a bibliographic catalogue of scientific papers, authors and institutions accessible in open access mode, named after the Library of Alexandria. It's citation coverage is excellent and I hope you will find utility in this listing of citing articles!
If you click the article title, you'll navigate to the article, as listed in CrossRef. If you click the Open Access links, you'll navigate to the "best Open Access location". Clicking the citation count will open this listing for that article. Lastly at the bottom of the page, you'll find basic pagination options.
Requested Article:
Machine learning and Python assisted design and verification of Fe–based amorphous/nanocrystalline alloy
Yichuan Tang, Yuan Wan, Zhongqi Wang, et al.
Materials & Design (2022) Vol. 219, pp. 110726-110726
Open Access | Times Cited: 15
Yichuan Tang, Yuan Wan, Zhongqi Wang, et al.
Materials & Design (2022) Vol. 219, pp. 110726-110726
Open Access | Times Cited: 15
Showing 15 citing articles:
Interpretable machine learning-assisted design of Fe-based nanocrystalline alloys with high saturation magnetic induction and low coercivity
Ning Zhang, Aina He, Gan Zhang, et al.
Journal of Material Science and Technology (2024) Vol. 188, pp. 73-83
Closed Access | Times Cited: 15
Ning Zhang, Aina He, Gan Zhang, et al.
Journal of Material Science and Technology (2024) Vol. 188, pp. 73-83
Closed Access | Times Cited: 15
Prediction of Vickers hardness of amorphous alloys based on interpretable machine learning
Xiaowei Liu, Zhilin Long, Peng Li
Journal of Non-Crystalline Solids (2022) Vol. 602, pp. 122095-122095
Closed Access | Times Cited: 28
Xiaowei Liu, Zhilin Long, Peng Li
Journal of Non-Crystalline Solids (2022) Vol. 602, pp. 122095-122095
Closed Access | Times Cited: 28
Machine learning assisted optimization of soft magnetic properties in ternary Fe–Si–Al alloys
V. A. Milyutin, Radovan Búreš, Mária Fáberová, et al.
Journal of Materials Research and Technology (2024) Vol. 29, pp. 5060-5073
Open Access | Times Cited: 7
V. A. Milyutin, Radovan Búreš, Mária Fáberová, et al.
Journal of Materials Research and Technology (2024) Vol. 29, pp. 5060-5073
Open Access | Times Cited: 7
Experimentally validated inverse design of multi-property Fe-Co-Ni alloys
Shakti P. Padhy, Varun Chaudhary, Yee-Fun Lim, et al.
iScience (2024) Vol. 27, Iss. 5, pp. 109723-109723
Open Access | Times Cited: 7
Shakti P. Padhy, Varun Chaudhary, Yee-Fun Lim, et al.
iScience (2024) Vol. 27, Iss. 5, pp. 109723-109723
Open Access | Times Cited: 7
Highly effective design of high GFA alloys with different metal-based and various components by machine learning
Yichuan Tang, Yifan He, ZhuoQun Fan, et al.
Science China Technological Sciences (2024) Vol. 67, Iss. 5, pp. 1431-1442
Closed Access | Times Cited: 7
Yichuan Tang, Yifan He, ZhuoQun Fan, et al.
Science China Technological Sciences (2024) Vol. 67, Iss. 5, pp. 1431-1442
Closed Access | Times Cited: 7
Alloys innovation through machine learning: a statistical literature review
Alireza Valizadeh, Ryoji Sahara, Maaouia Souissi
Science and Technology of Advanced Materials Methods (2024) Vol. 4, Iss. 1
Open Access | Times Cited: 4
Alireza Valizadeh, Ryoji Sahara, Maaouia Souissi
Science and Technology of Advanced Materials Methods (2024) Vol. 4, Iss. 1
Open Access | Times Cited: 4
Predicting the magnetic properties of Fe-based bulk metallic glasses by ensemble machine learning and interpretable information
Qiulin Bi, Jialiang Liu, Junting Li, et al.
Journal of Alloys and Compounds (2025), pp. 178858-178858
Closed Access
Qiulin Bi, Jialiang Liu, Junting Li, et al.
Journal of Alloys and Compounds (2025), pp. 178858-178858
Closed Access
Machine learning approach for predicting the fracture toughness of bulk metallic glasses
Hui Guo, Wenhai Sun, Weiyan Lü, et al.
The Philosophical Magazine A Journal of Theoretical Experimental and Applied Physics (2025), pp. 1-15
Closed Access
Hui Guo, Wenhai Sun, Weiyan Lü, et al.
The Philosophical Magazine A Journal of Theoretical Experimental and Applied Physics (2025), pp. 1-15
Closed Access
Investigation on the thermal stability, crystallization kinetics and magnetic properties of Fe-Si-B-P-C-Nb amorphous alloys
Yifan He, Rui Sun, Zilong Xu, et al.
Progress in Natural Science Materials International (2025)
Closed Access
Yifan He, Rui Sun, Zilong Xu, et al.
Progress in Natural Science Materials International (2025)
Closed Access
Machine Learning Tools and Web Services for Materials Science Modeling
Shahin Ahmadi, Azizeh Abdolmaleki, Fereshteh Shiri, et al.
Challenges and advances in computational chemistry and physics (2025), pp. 253-282
Closed Access
Shahin Ahmadi, Azizeh Abdolmaleki, Fereshteh Shiri, et al.
Challenges and advances in computational chemistry and physics (2025), pp. 253-282
Closed Access
Extremely wide annealing processing window in Fe-based amorphous/nanocrystalline alloy guided by machine learning
Rui Sun, Lichen Liu, Yifan He, et al.
Journal of Alloys and Compounds (2025), pp. 180238-180238
Closed Access
Rui Sun, Lichen Liu, Yifan He, et al.
Journal of Alloys and Compounds (2025), pp. 180238-180238
Closed Access
A Review of Artificial Intelligence‐Driven Innovations in Soft Magnetic Materials Optimization: Current Trends and Future Horizons
Yichuan Tang, Shaopeng Liu, Silong Li, et al.
MetalMat (2025)
Open Access
Yichuan Tang, Shaopeng Liu, Silong Li, et al.
MetalMat (2025)
Open Access
XGBoost algorithm-assisted prediction of amorphous formability of Fe-based nanocrystalline precursor
Yu Han, Gan Zhang, Fuyao Yang, et al.
Intermetallics (2024) Vol. 166, pp. 108207-108207
Closed Access | Times Cited: 3
Yu Han, Gan Zhang, Fuyao Yang, et al.
Intermetallics (2024) Vol. 166, pp. 108207-108207
Closed Access | Times Cited: 3
Prediction of magnetocaloric properties of Fe-based amorphous alloys based on interpretable machine learning
Chengcheng Liu, Xuandong Wang, Weidong Cai, et al.
Journal of Non-Crystalline Solids (2023) Vol. 625, pp. 122749-122749
Closed Access | Times Cited: 7
Chengcheng Liu, Xuandong Wang, Weidong Cai, et al.
Journal of Non-Crystalline Solids (2023) Vol. 625, pp. 122749-122749
Closed Access | Times Cited: 7
Customized design of amorphous solids by generative deep learning
Yinghui Shang, Ziqing Zhou, Han Rong, et al.
The Innovation Materials (2024) Vol. 2, Iss. 2, pp. 100071-100071
Open Access | Times Cited: 1
Yinghui Shang, Ziqing Zhou, Han Rong, et al.
The Innovation Materials (2024) Vol. 2, Iss. 2, pp. 100071-100071
Open Access | Times Cited: 1
Accurate prediction of magnetocaloric effect in NiMn-based Heusler alloys by prioritizing phase transitions through explainable machine learning
Yichuan Tang, Kaiyan Cao, Ruonan Ma, et al.
Rare Metals (2024) Vol. 44, Iss. 1, pp. 639-651
Closed Access | Times Cited: 1
Yichuan Tang, Kaiyan Cao, Ruonan Ma, et al.
Rare Metals (2024) Vol. 44, Iss. 1, pp. 639-651
Closed Access | Times Cited: 1
Prediction of external corrosion rate in Oil and Gas platforms using ensemble learning
Fernanda Ramos Elmas, Marina Polonia Rios, Eduardo R. A. Lima, et al.
Brazilian Journal of Operations & Production Management (2023) Vol. 20, Iss. 3, pp. 1952-1952
Open Access | Times Cited: 4
Fernanda Ramos Elmas, Marina Polonia Rios, Eduardo R. A. Lima, et al.
Brazilian Journal of Operations & Production Management (2023) Vol. 20, Iss. 3, pp. 1952-1952
Open Access | Times Cited: 4
Machine learning assisted design and preparation of Fe85Si2B8.5P3.5C1 amorphous/nanocrystalline alloy with high B and low H
Shihao Tang, Rui Sun, Yifan He, et al.
Materials & Design (2024), pp. 113461-113461
Open Access | Times Cited: 1
Shihao Tang, Rui Sun, Yifan He, et al.
Materials & Design (2024), pp. 113461-113461
Open Access | Times Cited: 1
Integrating Ultra-high Saturation Magnetization Intensity and Extreme-low Coercivity in FeCoBSiCu Alloy Assisted by Machine Learning
Wenhui Guo, You Wu, Lingxiang Shi, et al.
Acta Materialia (2024), pp. 120643-120643
Closed Access
Wenhui Guo, You Wu, Lingxiang Shi, et al.
Acta Materialia (2024), pp. 120643-120643
Closed Access
Machine Learning Application for Functional Properties Prediction in Magnetic Materials
V. A. Milyutin, N. N. Nikulchenkov
The Physics of Metals and Metallography (2024) Vol. 125, Iss. 12, pp. 1351-1366
Closed Access
V. A. Milyutin, N. N. Nikulchenkov
The Physics of Metals and Metallography (2024) Vol. 125, Iss. 12, pp. 1351-1366
Closed Access