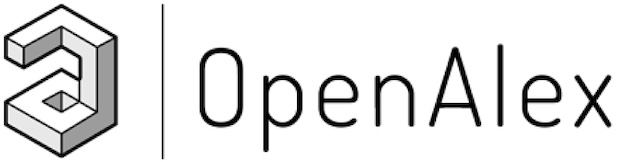
OpenAlex is a bibliographic catalogue of scientific papers, authors and institutions accessible in open access mode, named after the Library of Alexandria. It's citation coverage is excellent and I hope you will find utility in this listing of citing articles!
If you click the article title, you'll navigate to the article, as listed in CrossRef. If you click the Open Access links, you'll navigate to the "best Open Access location". Clicking the citation count will open this listing for that article. Lastly at the bottom of the page, you'll find basic pagination options.
Requested Article:
Flood hazard risk assessment model based on random forest
Zhaoli Wang, Chengguang Lai, Xiaohong Chen, et al.
Journal of Hydrology (2015) Vol. 527, pp. 1130-1141
Closed Access | Times Cited: 689
Zhaoli Wang, Chengguang Lai, Xiaohong Chen, et al.
Journal of Hydrology (2015) Vol. 527, pp. 1130-1141
Closed Access | Times Cited: 689
Showing 1-25 of 689 citing articles:
A Brief Review of Random Forests for Water Scientists and Practitioners and Their Recent History in Water Resources
Hristos Tyralis, Georgia Papacharalampous, Andreas Langousis
Water (2019) Vol. 11, Iss. 5, pp. 910-910
Open Access | Times Cited: 561
Hristos Tyralis, Georgia Papacharalampous, Andreas Langousis
Water (2019) Vol. 11, Iss. 5, pp. 910-910
Open Access | Times Cited: 561
Assessment of advanced random forest and decision tree algorithms for modeling rainfall-induced landslide susceptibility in the Izu-Oshima Volcanic Island, Japan
Jie Dou, Ali P. Yunus, Dieu Tien Bui, et al.
The Science of The Total Environment (2019) Vol. 662, pp. 332-346
Closed Access | Times Cited: 491
Jie Dou, Ali P. Yunus, Dieu Tien Bui, et al.
The Science of The Total Environment (2019) Vol. 662, pp. 332-346
Closed Access | Times Cited: 491
Flood Prediction Using Machine Learning Models: Literature Review
Amir Mosavi, Pınar Öztürk, Kwok‐wing Chau
Water (2018) Vol. 10, Iss. 11, pp. 1536-1536
Open Access | Times Cited: 474
Amir Mosavi, Pınar Öztürk, Kwok‐wing Chau
Water (2018) Vol. 10, Iss. 11, pp. 1536-1536
Open Access | Times Cited: 474
Flood susceptibility assessment in Hengfeng area coupling adaptive neuro-fuzzy inference system with genetic algorithm and differential evolution
Haoyuan Hong, Mahdi Panahi, Ataollah Shirzadi, et al.
The Science of The Total Environment (2018) Vol. 621, pp. 1124-1141
Closed Access | Times Cited: 375
Haoyuan Hong, Mahdi Panahi, Ataollah Shirzadi, et al.
The Science of The Total Environment (2018) Vol. 621, pp. 1124-1141
Closed Access | Times Cited: 375
Urban flood risk mapping using the GARP and QUEST models: A comparative study of machine learning techniques
Hamid Darabi, Bahram Choubin, Omid Rahmati, et al.
Journal of Hydrology (2018) Vol. 569, pp. 142-154
Open Access | Times Cited: 353
Hamid Darabi, Bahram Choubin, Omid Rahmati, et al.
Journal of Hydrology (2018) Vol. 569, pp. 142-154
Open Access | Times Cited: 353
Urban flooding risk assessment based on an integrated k-means cluster algorithm and improved entropy weight method in the region of Haikou, China
Hongshi Xu, Chao Ma, Jijian Lian, et al.
Journal of Hydrology (2018) Vol. 563, pp. 975-986
Closed Access | Times Cited: 340
Hongshi Xu, Chao Ma, Jijian Lian, et al.
Journal of Hydrology (2018) Vol. 563, pp. 975-986
Closed Access | Times Cited: 340
Spatial prediction of flood susceptibility using random-forest and boosted-tree models in Seoul metropolitan city, Korea
Sunmin Lee, Jeong-Cheol Kim, Hyung-Sup Jung, et al.
Geomatics Natural Hazards and Risk (2017) Vol. 8, Iss. 2, pp. 1185-1203
Open Access | Times Cited: 328
Sunmin Lee, Jeong-Cheol Kim, Hyung-Sup Jung, et al.
Geomatics Natural Hazards and Risk (2017) Vol. 8, Iss. 2, pp. 1185-1203
Open Access | Times Cited: 328
Mapping flood susceptibility in mountainous areas on a national scale in China
Gang Zhao, Bo Pang, Zongxue Xu, et al.
The Science of The Total Environment (2017) Vol. 615, pp. 1133-1142
Closed Access | Times Cited: 323
Gang Zhao, Bo Pang, Zongxue Xu, et al.
The Science of The Total Environment (2017) Vol. 615, pp. 1133-1142
Closed Access | Times Cited: 323
Flash-flood hazard assessment using ensembles and Bayesian-based machine learning models: Application of the simulated annealing feature selection method
Farzaneh Sajedi Hosseini, Bahram Choubin, Amir Mosavi, et al.
The Science of The Total Environment (2019) Vol. 711, pp. 135161-135161
Open Access | Times Cited: 297
Farzaneh Sajedi Hosseini, Bahram Choubin, Amir Mosavi, et al.
The Science of The Total Environment (2019) Vol. 711, pp. 135161-135161
Open Access | Times Cited: 297
Inundation risk assessment of metro system using AHP and TFN-AHP in Shenzhen
Hai‐Min Lyu, Wan‐Huan Zhou, Shui‐Long Shen, et al.
Sustainable Cities and Society (2020) Vol. 56, pp. 102103-102103
Closed Access | Times Cited: 290
Hai‐Min Lyu, Wan‐Huan Zhou, Shui‐Long Shen, et al.
Sustainable Cities and Society (2020) Vol. 56, pp. 102103-102103
Closed Access | Times Cited: 290
XGBoost-based method for flash flood risk assessment
Meihong Ma, Gang Zhao, Bingshun He, et al.
Journal of Hydrology (2021) Vol. 598, pp. 126382-126382
Closed Access | Times Cited: 274
Meihong Ma, Gang Zhao, Bingshun He, et al.
Journal of Hydrology (2021) Vol. 598, pp. 126382-126382
Closed Access | Times Cited: 274
Bearing Fault Diagnosis Method Based on Deep Convolutional Neural Network and Random Forest Ensemble Learning
Gaowei Xu, Min Liu, Zhuofu Jiang, et al.
Sensors (2019) Vol. 19, Iss. 5, pp. 1088-1088
Open Access | Times Cited: 261
Gaowei Xu, Min Liu, Zhuofu Jiang, et al.
Sensors (2019) Vol. 19, Iss. 5, pp. 1088-1088
Open Access | Times Cited: 261
GIS-based MCDM – AHP modeling for flood susceptibility mapping of arid areas, southeastern Tunisia
Dhekra Souissi, Lahcen Zouhri, Salma Hammami, et al.
Geocarto International (2019) Vol. 35, Iss. 9, pp. 991-1017
Closed Access | Times Cited: 258
Dhekra Souissi, Lahcen Zouhri, Salma Hammami, et al.
Geocarto International (2019) Vol. 35, Iss. 9, pp. 991-1017
Closed Access | Times Cited: 258
Basic Tenets of Classification Algorithms K-Nearest-Neighbor, Support Vector Machine, Random Forest and Neural Network: A Review
Ernest Yeboah Boateng, Joseph Otoo, Daniel A. Abaye
Journal of Data Analysis and Information Processing (2020) Vol. 08, Iss. 04, pp. 341-357
Open Access | Times Cited: 225
Ernest Yeboah Boateng, Joseph Otoo, Daniel A. Abaye
Journal of Data Analysis and Information Processing (2020) Vol. 08, Iss. 04, pp. 341-357
Open Access | Times Cited: 225
Flood Prediction Using Machine Learning, Literature Review
Amir Mosavi, Pınar Öztürk, Kwok‐wing Chau
(2018)
Open Access | Times Cited: 225
Amir Mosavi, Pınar Öztürk, Kwok‐wing Chau
(2018)
Open Access | Times Cited: 225
Assessment of urban flood susceptibility using semi-supervised machine learning model
Gang Zhao, Bo Pang, Zongxue Xu, et al.
The Science of The Total Environment (2018) Vol. 659, pp. 940-949
Closed Access | Times Cited: 223
Gang Zhao, Bo Pang, Zongxue Xu, et al.
The Science of The Total Environment (2018) Vol. 659, pp. 940-949
Closed Access | Times Cited: 223
Linking ecosystem services and ecosystem health to ecological risk assessment: A case study of the Beijing-Tianjin-Hebei urban agglomeration
Peng Kang, Weiping Chen, Ying Hou, et al.
The Science of The Total Environment (2018) Vol. 636, pp. 1442-1454
Closed Access | Times Cited: 216
Peng Kang, Weiping Chen, Ying Hou, et al.
The Science of The Total Environment (2018) Vol. 636, pp. 1442-1454
Closed Access | Times Cited: 216
Comparison of random forests and support vector machine for real-time radar-derived rainfall forecasting
Pao‐Shan Yu, Tao-Chang Yang, Szu-Yin Chen, et al.
Journal of Hydrology (2017) Vol. 552, pp. 92-104
Closed Access | Times Cited: 214
Pao‐Shan Yu, Tao-Chang Yang, Szu-Yin Chen, et al.
Journal of Hydrology (2017) Vol. 552, pp. 92-104
Closed Access | Times Cited: 214
Scenario-based flood risk assessment for urbanizing deltas using future land-use simulation (FLUS): Guangzhou Metropolitan Area as a case study
Weibin Lin, Yimin Sun, Steffen Nijhuis, et al.
The Science of The Total Environment (2020) Vol. 739, pp. 139899-139899
Closed Access | Times Cited: 209
Weibin Lin, Yimin Sun, Steffen Nijhuis, et al.
The Science of The Total Environment (2020) Vol. 739, pp. 139899-139899
Closed Access | Times Cited: 209
GIS-based flood hazard mapping using relative frequency ratio method: A case study of Panjkora River Basin, eastern Hindu Kush, Pakistan
Kashif Ullah, Jiquan Zhang
PLoS ONE (2020) Vol. 15, Iss. 3, pp. e0229153-e0229153
Open Access | Times Cited: 207
Kashif Ullah, Jiquan Zhang
PLoS ONE (2020) Vol. 15, Iss. 3, pp. e0229153-e0229153
Open Access | Times Cited: 207
Evaluating urban flood risk using hybrid method of TOPSIS and machine learning
Elham Rafiei-Sardooi, Ali Azareh, Bahram Choubin, et al.
International Journal of Disaster Risk Reduction (2021) Vol. 66, pp. 102614-102614
Open Access | Times Cited: 204
Elham Rafiei-Sardooi, Ali Azareh, Bahram Choubin, et al.
International Journal of Disaster Risk Reduction (2021) Vol. 66, pp. 102614-102614
Open Access | Times Cited: 204
Assessing and mapping multi-hazard risk susceptibility using a machine learning technique
Hamid Reza Pourghasemi, Narges Kariminejad, Mahdis Amiri, et al.
Scientific Reports (2020) Vol. 10, Iss. 1
Open Access | Times Cited: 194
Hamid Reza Pourghasemi, Narges Kariminejad, Mahdis Amiri, et al.
Scientific Reports (2020) Vol. 10, Iss. 1
Open Access | Times Cited: 194
Response of net primary production to land use and land cover change in mainland China since the late 1980s
Jun Li, Zhaoli Wang, Chengguang Lai, et al.
The Science of The Total Environment (2018) Vol. 639, pp. 237-247
Closed Access | Times Cited: 178
Jun Li, Zhaoli Wang, Chengguang Lai, et al.
The Science of The Total Environment (2018) Vol. 639, pp. 237-247
Closed Access | Times Cited: 178
Predicting types of occupational accidents at construction sites in Korea using random forest model
Kyungsu Kang, Han-Guk Ryu
Safety Science (2019) Vol. 120, pp. 226-236
Closed Access | Times Cited: 171
Kyungsu Kang, Han-Guk Ryu
Safety Science (2019) Vol. 120, pp. 226-236
Closed Access | Times Cited: 171
Are precipitation concentration and intensity changing in Bangladesh overtimes? Analysis of the possible causes of changes in precipitation systems
Md. Siddiqur Rahman, Abu Reza Md. Towfiqul Islam
The Science of The Total Environment (2019) Vol. 690, pp. 370-387
Closed Access | Times Cited: 166
Md. Siddiqur Rahman, Abu Reza Md. Towfiqul Islam
The Science of The Total Environment (2019) Vol. 690, pp. 370-387
Closed Access | Times Cited: 166