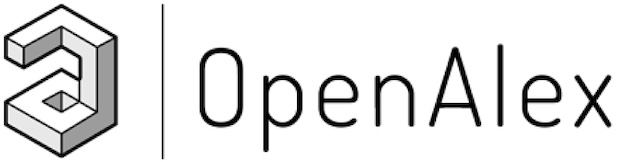
OpenAlex is a bibliographic catalogue of scientific papers, authors and institutions accessible in open access mode, named after the Library of Alexandria. It's citation coverage is excellent and I hope you will find utility in this listing of citing articles!
If you click the article title, you'll navigate to the article, as listed in CrossRef. If you click the Open Access links, you'll navigate to the "best Open Access location". Clicking the citation count will open this listing for that article. Lastly at the bottom of the page, you'll find basic pagination options.
Requested Article:
Machine learning-assisted selection of adsorption-based carbon dioxide capture materials
Eslam G. Al-Sakkari, Ahmed Ragab, Terry M.Y. So, et al.
Journal of environmental chemical engineering (2023) Vol. 11, Iss. 5, pp. 110732-110732
Closed Access | Times Cited: 13
Eslam G. Al-Sakkari, Ahmed Ragab, Terry M.Y. So, et al.
Journal of environmental chemical engineering (2023) Vol. 11, Iss. 5, pp. 110732-110732
Closed Access | Times Cited: 13
Showing 13 citing articles:
Carbon capture, utilization and sequestration systems design and operation optimization: Assessment and perspectives of artificial intelligence opportunities
Eslam G. Al-Sakkari, Ahmed Ragab, Hanane Dagdougui, et al.
The Science of The Total Environment (2024) Vol. 917, pp. 170085-170085
Closed Access | Times Cited: 23
Eslam G. Al-Sakkari, Ahmed Ragab, Hanane Dagdougui, et al.
The Science of The Total Environment (2024) Vol. 917, pp. 170085-170085
Closed Access | Times Cited: 23
Highly re-usable porous carbon-based particles as adsorbents for the development of CO2 capture technologies
Betül Ari, Aydın K. Sunol, Nurettin Şahiner
Journal of CO2 Utilization (2024) Vol. 82, pp. 102767-102767
Open Access | Times Cited: 7
Betül Ari, Aydın K. Sunol, Nurettin Şahiner
Journal of CO2 Utilization (2024) Vol. 82, pp. 102767-102767
Open Access | Times Cited: 7
Direct Air Capture (DAC) for Achieving Net-Zero CO2 Emissions: Advances, Applications, and Challenges
Guihe Li, Jia Yao
Eng—Advances in Engineering (2024) Vol. 5, Iss. 3, pp. 1298-1336
Open Access | Times Cited: 7
Guihe Li, Jia Yao
Eng—Advances in Engineering (2024) Vol. 5, Iss. 3, pp. 1298-1336
Open Access | Times Cited: 7
Optimization of CO2 absorption rate for environmental applications and effective carbon capture
Imtiaz Afzal Khan, Sani I. Abba, Jamilu Usman, et al.
Journal of Cleaner Production (2025), pp. 144707-144707
Closed Access
Imtiaz Afzal Khan, Sani I. Abba, Jamilu Usman, et al.
Journal of Cleaner Production (2025), pp. 144707-144707
Closed Access
Supervised machine learning-based categorization and prediction of uranium adsorption capacity on various process parameters
Niken Siwi Pamungkas, Zico Pratama Putra, Hendra Adhi Pratama, et al.
Journal of Hazardous Materials Advances (2024) Vol. 17, pp. 100523-100523
Open Access | Times Cited: 2
Niken Siwi Pamungkas, Zico Pratama Putra, Hendra Adhi Pratama, et al.
Journal of Hazardous Materials Advances (2024) Vol. 17, pp. 100523-100523
Open Access | Times Cited: 2
Hierarchically porous carbon foams coated with carbon nitride: Insights into adsorbents for pre-combustion and post-combustion CO2 separation
Maryna Vorokhta, Muhammad Irfan Maulana Kusdhany, Martina Švábová, et al.
Separation and Purification Technology (2024) Vol. 354, pp. 129054-129054
Open Access | Times Cited: 1
Maryna Vorokhta, Muhammad Irfan Maulana Kusdhany, Martina Švábová, et al.
Separation and Purification Technology (2024) Vol. 354, pp. 129054-129054
Open Access | Times Cited: 1
Accelerated Discovery of CO2 Solid Sorbents Using Active Machine Learning: Review and Perspectives
Deyang Xu, Jing Yang, Zhaoxiang Xu, et al.
Energy & Fuels (2024)
Closed Access | Times Cited: 1
Deyang Xu, Jing Yang, Zhaoxiang Xu, et al.
Energy & Fuels (2024)
Closed Access | Times Cited: 1
Leveraging experimental and computational tools for advancing carbon capture adsorbents research
Niranjan Ramasamy, Anitha Jegadeeshwari Lakshmana Peru Raj, Vedha Varshini Akula, et al.
Environmental Science and Pollution Research (2024) Vol. 31, Iss. 43, pp. 55069-55098
Closed Access | Times Cited: 1
Niranjan Ramasamy, Anitha Jegadeeshwari Lakshmana Peru Raj, Vedha Varshini Akula, et al.
Environmental Science and Pollution Research (2024) Vol. 31, Iss. 43, pp. 55069-55098
Closed Access | Times Cited: 1
Recent Applications of Explainable AI (XAI): A Systematic Literature Review
Mirka Saarela, Vili Podgorelec
Applied Sciences (2024) Vol. 14, Iss. 19, pp. 8884-8884
Open Access | Times Cited: 1
Mirka Saarela, Vili Podgorelec
Applied Sciences (2024) Vol. 14, Iss. 19, pp. 8884-8884
Open Access | Times Cited: 1
Advancements and Challenges in Adsorption‐Based Carbon Capture Technology: From Fundamentals to Deployment
Hamid Zentou, Mansur Aliyu, Mahmoud A. Abdalla, et al.
The Chemical Record (2024)
Closed Access | Times Cited: 1
Hamid Zentou, Mansur Aliyu, Mahmoud A. Abdalla, et al.
The Chemical Record (2024)
Closed Access | Times Cited: 1
Ensemble machine learning to accelerate industrial decarbonization: Prediction of Hansen solubility parameters for streamlined chemical solvent selection
Eslam G. Al-Sakkari, Ahmed Ragab, Mostafa Amer, et al.
Digital Chemical Engineering (2024), pp. 100207-100207
Open Access | Times Cited: 1
Eslam G. Al-Sakkari, Ahmed Ragab, Mostafa Amer, et al.
Digital Chemical Engineering (2024), pp. 100207-100207
Open Access | Times Cited: 1
Learn-To-Design: Reinforcement Learning-Assisted Chemical Process Optimization
Eslam G. Al-Sakkari, Ahmed Ragab, Mohamed Ali, et al.
Systems & control transactions. (2024) Vol. 3, pp. 245-252
Closed Access
Eslam G. Al-Sakkari, Ahmed Ragab, Mohamed Ali, et al.
Systems & control transactions. (2024) Vol. 3, pp. 245-252
Closed Access
Prediction of the enhanced performance of Cu(I)-modified porous materials towards CO adsorption by using tree-based machine learning models
Wuqing Tao, Yongjie Cui, Qidong Zhao, et al.
Separation and Purification Technology (2024), pp. 130850-130850
Closed Access
Wuqing Tao, Yongjie Cui, Qidong Zhao, et al.
Separation and Purification Technology (2024), pp. 130850-130850
Closed Access